Machine Learning Optimizing Renewable Energy Integration Solutions
Topic: AI Research Tools
Industry: Energy and Utilities
Discover how machine learning optimizes renewable energy integration enhancing efficiency in production distribution and consumption for a sustainable future
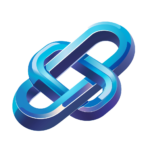
How Machine Learning is Optimizing Renewable Energy Integration
Introduction to Machine Learning in Energy
As the world increasingly shifts towards sustainable energy sources, the integration of renewable energy into existing power systems has become a critical challenge. Machine learning (ML), a subset of artificial intelligence (AI), plays a pivotal role in addressing this challenge by optimizing various aspects of energy production, distribution, and consumption. This article explores how machine learning can be implemented in the energy sector, focusing on AI research tools that enhance the integration of renewable energy sources.
Understanding the Role of Machine Learning
Machine learning algorithms can analyze vast amounts of data generated by renewable energy systems, such as solar panels and wind turbines. By identifying patterns and trends, these algorithms provide valuable insights that can lead to more efficient energy management. The primary areas where ML can optimize renewable energy integration include:
1. Predictive Analytics
Predictive analytics involves forecasting energy generation and consumption patterns. ML models can analyze historical weather data, energy usage trends, and other relevant factors to predict the availability of renewable energy. For instance, tools like IBM Watson Studio allow energy companies to build predictive models that enhance decision-making processes related to energy supply and demand.
2. Grid Management
Integrating renewable energy sources into the grid requires real-time monitoring and management to ensure stability. Machine learning algorithms can optimize grid operations by predicting load demands and adjusting the energy supply accordingly. Solutions like Siemens’ Spectrum Power leverage AI to enhance grid management, enabling utilities to balance supply and demand efficiently.
3. Energy Storage Optimization
Energy storage systems are essential for managing the intermittent nature of renewable energy sources. Machine learning can optimize the charging and discharging cycles of batteries, ensuring that energy is stored when production exceeds demand and released when needed. Tools such as Fluence’s AI-driven energy storage solutions utilize machine learning to enhance the performance and lifespan of storage systems.
AI-Driven Products Enhancing Renewable Energy Integration
Several AI-driven products are making significant contributions to the integration of renewable energy. Below are a few notable examples:
1. Google DeepMind’s Energy Optimization
Google DeepMind has developed AI algorithms that optimize energy usage in data centers by predicting cooling needs based on historical data. This not only reduces energy consumption but also supports the integration of renewable energy sources into the grid.
2. AutoGrid’s Flexibility Management
AutoGrid’s AI platform helps utilities manage demand response programs by predicting customer behavior and optimizing energy distribution. This tool enhances the integration of renewable energy by ensuring that demand aligns with supply, particularly during peak production times.
3. Enel’s Advanced Analytics
Enel, a global energy company, employs advanced analytics powered by machine learning to optimize its renewable energy portfolio. The company uses AI to analyze data from various sources, including weather forecasts and market trends, to make informed decisions regarding energy production and distribution.
Challenges and Future Directions
While the potential of machine learning in optimizing renewable energy integration is substantial, several challenges remain. Data privacy, algorithm transparency, and the need for robust data infrastructure are critical considerations that must be addressed. However, as technology continues to evolve, the future of machine learning in the energy sector looks promising.
Conclusion
Machine learning is revolutionizing the way renewable energy is integrated into existing power systems. By leveraging AI research tools and innovative products, energy companies can optimize operations, enhance grid stability, and promote sustainable practices. The ongoing advancements in machine learning will undoubtedly play a crucial role in shaping a more sustainable energy future.
Keyword: machine learning renewable energy integration