Machine Learning Revolutionizes Material Cost Estimation for Builders
Topic: AI Real Estate Tools
Industry: Construction Companies
Discover how machine learning revolutionizes material cost estimation in construction enhancing accuracy efficiency and competitiveness for builders.
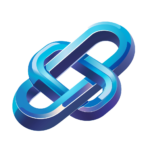
Machine Learning in Material Cost Estimation: Game-Changing Tools for Builders
The Evolution of Cost Estimation in Construction
In the ever-evolving landscape of construction, accurate material cost estimation is paramount for project success. Traditional methods, often reliant on manual calculations and historical data, can lead to inefficiencies and inaccuracies. However, the advent of machine learning (ML) has introduced innovative solutions that not only streamline the estimation process but also enhance precision and reduce costs.
Understanding Machine Learning in Cost Estimation
Machine learning, a subset of artificial intelligence (AI), involves algorithms that learn from data, identify patterns, and make decisions with minimal human intervention. In the context of material cost estimation, ML can analyze vast datasets, including historical project costs, market trends, and supplier pricing, to provide real-time estimates that adapt to changing conditions.
Key Benefits of Machine Learning in Construction
- Increased Accuracy: By leveraging historical data and predictive analytics, ML algorithms can significantly reduce estimation errors.
- Time Efficiency: Automated processes save time, allowing construction companies to focus on project execution rather than tedious calculations.
- Dynamic Pricing: ML tools can adjust estimates based on real-time market fluctuations, ensuring that builders remain competitive.
- Risk Mitigation: Enhanced forecasting capabilities help identify potential cost overruns before they occur, allowing for proactive management.
Implementing AI in Material Cost Estimation
To effectively integrate machine learning into material cost estimation, construction companies can utilize various AI-driven tools designed specifically for this purpose. Below are some notable examples:
1. ProEst
ProEst is a cloud-based construction estimating software that incorporates machine learning algorithms to provide accurate material cost estimates. By analyzing previous projects and current market data, ProEst helps builders generate estimates quickly while minimizing the risk of errors.
2. PlanSwift
PlanSwift offers takeoff and estimating solutions that leverage AI to streamline the process of material quantity takeoffs. By automating measurements and calculations, PlanSwift enables builders to produce precise estimates, saving both time and resources.
3. BidClerk
BidClerk is an AI-powered platform that aggregates construction project data and offers insights into material costs. By utilizing historical bidding data and project specifics, BidClerk assists builders in making informed decisions about material procurement and pricing strategies.
4. SmartBid
SmartBid is a construction bid management software that employs machine learning to enhance the bidding process. It analyzes past bids and project outcomes to provide builders with insights that can improve their cost estimation accuracy and competitiveness.
Challenges and Considerations
While the benefits of machine learning in material cost estimation are substantial, construction companies must also consider several challenges. Data quality and availability are critical; inaccurate or incomplete data can lead to misguided estimates. Additionally, the integration of AI tools into existing workflows requires training and change management to ensure that teams can effectively utilize these new technologies.
The Future of Cost Estimation in Construction
As machine learning continues to advance, the potential for innovation in material cost estimation is vast. Builders who embrace these AI-driven tools will not only enhance their operational efficiency but also position themselves as leaders in a highly competitive market. By leveraging the capabilities of machine learning, construction companies can transform their approach to cost estimation, ensuring that they remain agile and responsive to the demands of the industry.
Conclusion
The integration of machine learning in material cost estimation represents a significant shift in how construction companies approach project budgeting. With tools like ProEst, PlanSwift, BidClerk, and SmartBid, builders can harness the power of AI to drive accuracy, efficiency, and profitability. As the industry continues to evolve, those who adapt and innovate will undoubtedly reap the benefits of this transformative technology.
Keyword: machine learning cost estimation construction