Machine Learning Transforming Retail Location Selection Strategies
Topic: AI Real Estate Tools
Industry: Retail Chains (for location selection)
Discover how machine learning transforms retail by identifying emerging hotspots through predictive analytics GIS technology and real-time data analysis for strategic growth
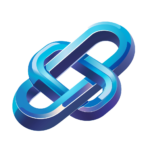
The Role of Machine Learning in Identifying Emerging Retail Hotspots
Understanding the Importance of Location in Retail
In the retail industry, the adage “location, location, location” holds significant weight. The success of a retail chain often hinges on its ability to identify and capitalize on emerging hotspots—areas that show potential for high foot traffic and consumer engagement. In recent years, the integration of artificial intelligence (AI) and machine learning (ML) has revolutionized how businesses approach location selection, providing data-driven insights that were previously unattainable.
The Power of Machine Learning in Retail Location Selection
Machine learning algorithms analyze vast amounts of data to uncover patterns and trends that inform strategic decision-making. By leveraging these technologies, retail chains can gain a competitive edge in identifying locations that are poised for growth. Here are several ways machine learning can be implemented in this context:
1. Predictive Analytics
Predictive analytics is a cornerstone of machine learning applications in retail. By utilizing historical sales data, demographic information, and market trends, AI tools can forecast future performance in potential retail locations. For instance, a retail chain might employ a tool like IBM Watson Studio to analyze consumer behavior patterns and predict which neighborhoods are likely to experience increased demand for their products.
2. Geographic Information Systems (GIS)
GIS technology, when combined with machine learning, allows retailers to visualize and analyze spatial data effectively. Tools such as ArcGIS leverage ML algorithms to assess geographic factors influencing retail success. Retailers can evaluate aspects such as proximity to competitors, accessibility, and socio-economic demographics to make informed location decisions.
3. Real-Time Data Analysis
In today’s fast-paced retail environment, real-time data analysis is crucial. Machine learning algorithms can process data from various sources, including social media, traffic patterns, and local events, to identify emerging trends. For example, Placer.ai provides insights into foot traffic patterns, helping retailers understand consumer behavior in specific areas and adjust their strategies accordingly.
4. Consumer Sentiment Analysis
Understanding consumer sentiment is vital for retail chains looking to expand. Machine learning can analyze online reviews, social media interactions, and customer feedback to gauge public perception of a brand in specific locations. Tools like Brandwatch utilize natural language processing (NLP) to sift through large volumes of text, providing retailers with actionable insights into consumer preferences and sentiments.
Case Studies: Successful Implementations
Several retail chains have successfully harnessed the power of machine learning to identify emerging hotspots:
Case Study 1: Starbucks
Starbucks has long relied on data-driven strategies for location selection. By employing machine learning algorithms to analyze demographic data, foot traffic, and even weather patterns, the company has successfully identified high-potential locations for new stores, optimizing its expansion strategy.
Case Study 2: Walmart
Walmart uses advanced analytics and machine learning to assess market conditions and consumer behavior. By integrating these insights into their site selection process, Walmart has been able to strategically position new stores in areas that align with consumer demand, ensuring long-term profitability.
Conclusion
The integration of machine learning and AI in retail location selection is not just a trend; it is a transformative approach that equips retail chains with the insights needed to thrive in a competitive landscape. By leveraging predictive analytics, GIS technology, real-time data analysis, and consumer sentiment analysis, retailers can identify emerging hotspots with unprecedented accuracy. As the retail landscape continues to evolve, embracing these AI-driven tools will be essential for sustained growth and success.
Keyword: machine learning retail location selection