Machine Learning Transforming Sustainable Agriculture Practices
Topic: AI Self Improvement Tools
Industry: Environmental and Climate Tech
Discover how machine learning is transforming sustainable agriculture by optimizing crop yields reducing waste and minimizing environmental impact
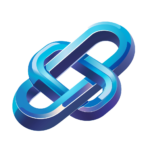
How Machine Learning is Revolutionizing Sustainable Agriculture Practices
The Intersection of AI and Agriculture
As the global population continues to rise, the demand for sustainable agricultural practices has never been more critical. Machine learning (ML), a subset of artificial intelligence (AI), is emerging as a transformative force in this sector. By leveraging advanced data analytics, machine learning enables farmers to optimize crop yields, reduce waste, and minimize environmental impact.
Understanding Machine Learning in Agriculture
Machine learning involves algorithms that can learn from and make predictions based on data. In agriculture, these algorithms analyze vast amounts of information, including weather patterns, soil conditions, and crop health, to provide actionable insights. This capability is essential for farmers aiming to implement sustainable practices that enhance productivity while conserving resources.
Key Applications of Machine Learning in Sustainable Agriculture
1. Precision Farming
Precision farming utilizes machine learning to analyze data collected from various sources, including satellite imagery and sensor networks. Tools like CropX and AgriWebb provide farmers with insights into soil health, moisture levels, and nutrient requirements. By applying these insights, farmers can optimize irrigation and fertilization, reducing waste and improving crop quality.
2. Pest and Disease Prediction
Machine learning algorithms can predict pest infestations and disease outbreaks by analyzing historical data and environmental conditions. Platforms such as Plantix use image recognition to identify plant diseases and recommend treatment options. This proactive approach not only protects crops but also minimizes the need for chemical interventions, supporting sustainable practices.
3. Yield Prediction
Accurate yield prediction is crucial for effective planning and resource allocation. AI-driven tools like IBM Watson Decision Platform for Agriculture harness machine learning to forecast crop yields based on a multitude of factors, including weather forecasts and historical yield data. This information allows farmers to make informed decisions about planting and harvesting, ultimately enhancing sustainability.
AI-Driven Products Supporting Sustainable Agriculture
Several innovative AI-driven products are making significant strides in promoting sustainable agricultural practices:
- FarmLogs: This platform uses machine learning to help farmers track field conditions, manage inputs, and analyze crop performance, all while promoting efficient resource use.
- Raven Applied Technology: With its AI-powered tools, Raven provides farmers with data-driven insights to optimize their operations, reduce waste, and enhance sustainability.
- Trace Genomics: This soil health platform uses machine learning to analyze soil samples, offering farmers insights into microbial communities and nutrient availability, which supports better soil management practices.
Challenges and Considerations
While the benefits of machine learning in sustainable agriculture are substantial, there are challenges to consider. Data privacy, the need for robust infrastructure, and the potential for technology adoption disparities among farmers are significant hurdles. Addressing these challenges requires collaboration between technology providers, agricultural stakeholders, and policymakers to create a supportive environment for innovation.
The Future of Sustainable Agriculture with Machine Learning
As machine learning continues to evolve, its potential to revolutionize sustainable agriculture practices will only grow. By embracing AI-driven tools, farmers can enhance their operations, reduce environmental impacts, and contribute to a more sustainable food system. The integration of machine learning into agriculture is not just a trend; it is a vital step toward ensuring food security and environmental sustainability for future generations.
Conclusion
In conclusion, the intersection of machine learning and sustainable agriculture presents a promising avenue for enhancing productivity and minimizing environmental impact. As more farmers adopt AI-driven tools, we can expect a significant shift toward more sustainable practices in agriculture, ultimately fostering a healthier planet.
Keyword: sustainable agriculture machine learning