Machine Learning vs Traditional Methods in Cyber Threat Intelligence
Topic: AI Coding Tools
Industry: Cybersecurity
Discover how machine learning transforms cybersecurity by enhancing threat detection and response capabilities compared to traditional methods in our latest blog post.
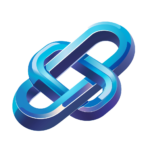
Machine Learning vs. Traditional Methods: A New Era in Cyber Threat Intelligence
Understanding the Shift in Cybersecurity Approaches
In recent years, the landscape of cybersecurity has evolved significantly, driven by the increasing sophistication of cyber threats. Traditional methods of threat intelligence, which often rely on human analysis and static rules, are being supplemented and, in some cases, replaced by machine learning (ML) and artificial intelligence (AI) technologies. This transition marks a pivotal moment in how organizations approach cybersecurity, enabling them to respond more effectively to emerging threats.The Limitations of Traditional Methods
Traditional cybersecurity methods typically involve manual processes, including the collection of threat data, analysis using predefined rules, and reactive measures to incidents. While these methods have served organizations well in the past, they face several limitations:- Scalability: As the volume of data generated by cyber threats increases, traditional methods struggle to keep pace.
- Speed: Manual analysis can be time-consuming, leaving organizations vulnerable to fast-moving threats.
- Adaptability: Static rules may fail to recognize new attack vectors, making it difficult to preemptively address threats.
Machine Learning: A Game Changer in Cyber Threat Intelligence
Machine learning offers a transformative approach to cybersecurity by leveraging algorithms that can learn from data, identify patterns, and make predictions. This capability allows organizations to detect anomalies and potential threats more efficiently than traditional methods. Here are several ways in which AI can be implemented in cybersecurity:1. Automated Threat Detection
Machine learning algorithms can analyze vast amounts of data in real-time, identifying unusual behaviors that may indicate a cyber threat. For instance, tools like Darktrace utilize unsupervised learning to create a baseline of normal network behavior, enabling the detection of deviations that could signify an attack.2. Predictive Analytics
By analyzing historical data, machine learning models can predict potential future threats. Solutions such as IBM Watson for Cyber Security utilize natural language processing to sift through unstructured data, providing insights that help organizations anticipate and mitigate risks before they materialize.3. Incident Response Optimization
AI-driven tools can streamline incident response by automating repetitive tasks and prioritizing alerts based on severity. For example, Cortex XSOAR by Palo Alto Networks integrates various security tools, allowing security teams to respond to incidents more effectively and efficiently.Examples of AI-Driven Products in Cybersecurity
The integration of machine learning into cybersecurity is reflected in various innovative products currently available in the market:1. CrowdStrike Falcon
CrowdStrike Falcon employs machine learning to provide endpoint protection. Its algorithms analyze data from millions of endpoints, enabling real-time detection and response to threats while minimizing false positives.2. Splunk Enterprise Security
Splunk harnesses machine learning to enhance its security analytics capabilities. By applying predictive analytics, it helps organizations identify potential threats and respond proactively, rather than reactively.3. Microsoft Azure Sentinel
Azure Sentinel is a cloud-native SIEM (Security Information and Event Management) solution that utilizes AI to analyze security data across an organization. It automates threat detection and response, providing security teams with valuable insights to safeguard their environments.Conclusion: Embracing the Future of Cybersecurity
As cyber threats continue to evolve, organizations must adapt their cybersecurity strategies to leverage the capabilities of machine learning and artificial intelligence. While traditional methods have their place, the advantages offered by AI-driven tools are undeniable. By embracing these technologies, organizations can enhance their threat intelligence, improve incident response times, and ultimately create a more resilient cybersecurity posture. The future of cybersecurity lies in the synergy between human expertise and machine intelligence, paving the way for a new era in cyber threat intelligence.Keyword: machine learning in cybersecurity