Multimodal AI Benefits for Decision Making in Manufacturing
Topic: AI Research Tools
Industry: Manufacturing
Discover how multimodal AI enhances decision-making in factory operations by improving efficiency predictive maintenance and quality control for smarter manufacturing.
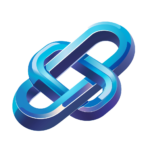
Multimodal AI: Enhancing Decision-Making in Factory Operations
Understanding Multimodal AI in Manufacturing
Multimodal AI refers to the integration of various data types—such as text, images, audio, and sensor data—into a cohesive analytical framework. In the context of manufacturing, this technology facilitates improved decision-making by providing a comprehensive view of operations. By leveraging multimodal AI, manufacturers can analyze diverse data sources simultaneously, leading to more informed and timely decisions.
Key Benefits of Multimodal AI in Factory Operations
- Improved Efficiency: Multimodal AI can streamline processes by analyzing performance data from different machines and production lines, identifying bottlenecks, and suggesting optimizations.
- Enhanced Predictive Maintenance: By integrating sensor data with historical maintenance records, AI can predict equipment failures before they occur, reducing downtime and maintenance costs.
- Better Quality Control: AI tools can analyze visual data from cameras to detect defects in real-time, ensuring that only high-quality products reach the market.
Implementation of AI in Manufacturing
To effectively implement multimodal AI in factory operations, organizations can follow a structured approach:
1. Data Collection
The first step involves gathering data from various sources, including IoT devices, production logs, and quality inspection systems. This diverse dataset forms the foundation for AI analysis.
2. Tool Selection
Selecting the right AI tools is crucial. Manufacturers can choose from a variety of platforms tailored for specific applications. Some notable examples include:
IBM Watson IoT
IBM Watson IoT combines AI with IoT data to enhance operational efficiency. It enables manufacturers to monitor equipment in real-time, analyze sensor data, and generate actionable insights for maintenance and production improvements.
Siemens MindSphere
Siemens MindSphere is a cloud-based IoT operating system that connects industrial machines and devices. It utilizes machine learning algorithms to analyze data from these devices, facilitating predictive maintenance and performance optimization.
Microsoft Azure Machine Learning
Microsoft Azure Machine Learning provides a robust environment for building, training, and deploying AI models. Manufacturers can use this platform to develop custom solutions that analyze production data and improve decision-making processes.
3. Model Training and Validation
Once the tools are selected, organizations must train AI models using the collected data. This involves validating the models to ensure accuracy and reliability in real-world applications.
4. Integration and Deployment
After successful training, the AI models can be integrated into existing manufacturing systems. This step often involves collaboration with IT and operational teams to ensure seamless deployment.
Real-World Examples of Multimodal AI in Action
Several manufacturers have successfully implemented multimodal AI to enhance their operations:
General Electric (GE)
GE utilizes AI-driven analytics in its manufacturing processes to monitor equipment health and optimize production schedules. By analyzing data from various sources, GE has significantly reduced downtime and improved overall efficiency.
Toyota
Toyota has integrated AI into its quality control processes, using computer vision to inspect vehicles for defects. This multimodal approach has enhanced the accuracy of inspections and reduced the number of faulty products reaching consumers.
Conclusion
As the manufacturing landscape becomes increasingly competitive, the adoption of multimodal AI represents a significant opportunity for organizations to enhance decision-making and operational efficiency. By leveraging the right AI tools and implementing a systematic approach, manufacturers can unlock the full potential of their data, leading to smarter operations and improved business outcomes.
Keyword: Multimodal AI in manufacturing