Multimodal AI in Radiology Enhancing Diagnostic Accuracy
Topic: AI Health Tools
Industry: Diagnostic imaging centers
Discover how multimodal AI is revolutionizing diagnostic accuracy in radiology enhancing patient care and transforming healthcare practices for better outcomes
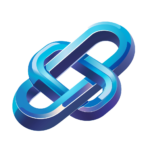
The Rise of Multimodal AI: Transforming Diagnostic Accuracy in Radiology
Understanding Multimodal AI in Radiology
In recent years, the integration of artificial intelligence (AI) in healthcare has gained significant traction, particularly in the field of radiology. Multimodal AI refers to the ability of AI systems to process and analyze data from various sources—such as images, text, and patient records—simultaneously. This capability not only enhances diagnostic accuracy but also streamlines workflows in diagnostic imaging centers.
The Importance of Diagnostic Accuracy
Diagnostic accuracy is crucial in radiology, as it directly impacts patient outcomes. Misdiagnosis can lead to inappropriate treatment plans, increased healthcare costs, and, most importantly, adverse effects on patient health. By leveraging multimodal AI, radiologists can improve their diagnostic precision, thereby enhancing patient care.
How Artificial Intelligence Can Be Implemented
Implementing AI in diagnostic imaging centers involves several key steps:
1. Data Integration
Successful AI deployment begins with the integration of diverse data sources. Radiology departments must ensure that imaging data, electronic health records (EHR), and clinical notes are accessible and can be processed by AI algorithms.
2. Training AI Models
AI models require extensive training with high-quality, annotated datasets. Radiologists and data scientists must collaborate to curate these datasets, ensuring that the AI can learn to recognize patterns and anomalies effectively.
3. Continuous Learning and Adaptation
AI systems should be designed to continuously learn from new data. As more imaging studies are performed, the AI can refine its algorithms, improving diagnostic accuracy over time.
Examples of AI-Driven Tools in Radiology
Several AI-driven products are currently transforming the landscape of diagnostic imaging. Below are notable examples:
1. Zebra Medical Vision
Zebra Medical Vision offers a suite of algorithms that analyze medical imaging data to detect various conditions, including cardiovascular diseases and cancers. By providing radiologists with automated insights, Zebra enhances diagnostic accuracy and reduces the workload on healthcare professionals.
2. Aidoc
Aidoc specializes in real-time analysis of CT scans, identifying critical conditions such as intracranial hemorrhages and pulmonary embolisms. By prioritizing urgent cases, Aidoc aids radiologists in making timely decisions, ultimately improving patient outcomes.
3. Qure.ai
Qure.ai employs deep learning algorithms to interpret chest X-rays and CT scans. Its AI solutions can detect conditions like tuberculosis and pneumonia, providing radiologists with a second opinion and helping to standardize interpretations across different practitioners.
Challenges and Considerations
While the benefits of multimodal AI in radiology are substantial, there are challenges that must be addressed:
1. Data Privacy and Security
Ensuring the privacy and security of patient data is paramount. Diagnostic imaging centers must comply with regulations such as HIPAA to protect sensitive information.
2. Integration with Existing Systems
Integrating AI solutions with existing radiology information systems (RIS) and picture archiving and communication systems (PACS) can be complex. A strategic approach is required to ensure seamless interoperability.
3. Training and Adoption
Radiologists and staff must be trained to effectively utilize AI tools. Emphasizing the role of AI as a supportive tool rather than a replacement is crucial for fostering acceptance within the medical community.
The Future of Multimodal AI in Radiology
The rise of multimodal AI represents a significant advancement in the field of radiology. As technology continues to evolve, we can expect even greater improvements in diagnostic accuracy, efficiency, and patient care. By embracing these innovations, diagnostic imaging centers can position themselves at the forefront of healthcare transformation, ultimately leading to better outcomes for patients.
Conclusion
In conclusion, the integration of multimodal AI in radiology is not merely a trend but a transformative shift that holds the potential to redefine diagnostic practices. As AI tools become more sophisticated and widely adopted, the future of radiology looks promising, with enhanced accuracy and improved patient care at the forefront of this evolution.
Keyword: multimodal AI in radiology