Natural Language Processing Tools for Analyzing Clinical Notes
Topic: AI Developer Tools
Industry: Healthcare
Discover top Natural Language Processing tools for analyzing clinical notes in healthcare Enhance patient care and streamline operations with AI-driven solutions
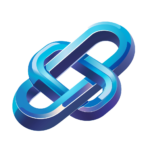
Natural Language Processing Tools for Analyzing Clinical Notes: A Comparison
Introduction to Natural Language Processing in Healthcare
Natural Language Processing (NLP) has emerged as a pivotal technology in the healthcare sector, particularly for analyzing clinical notes. With the increasing volume of unstructured data generated in medical settings, NLP tools provide a means to extract meaningful insights from clinical narratives, enhancing patient care and operational efficiency.
The Role of Artificial Intelligence in Clinical Data Analysis
Artificial intelligence (AI), particularly through NLP, enables healthcare providers to automate the extraction of information from clinical notes. This automation not only saves time but also reduces the risk of human error in data interpretation. By leveraging AI-driven tools, healthcare organizations can improve decision-making processes, enhance patient outcomes, and streamline administrative tasks.
Key NLP Tools for Analyzing Clinical Notes
Several NLP tools are specifically designed for the healthcare sector, each offering unique features and capabilities. Below, we compare some of the most prominent options available today.
1. IBM Watson Natural Language Understanding
IBM Watson NLU is a powerful tool that leverages machine learning and NLP to analyze clinical notes. It can identify entities, sentiments, and concepts within unstructured text. For instance, Watson can extract patient symptoms, medications, and diagnoses, providing healthcare professionals with a comprehensive overview of a patient’s condition.
2. Google Cloud Natural Language API
Google Cloud’s NLP API is another robust option that offers powerful text analysis capabilities. It can analyze the structure and meaning of clinical notes, enabling users to extract relevant information efficiently. The API supports multiple languages and can be integrated with existing healthcare systems, making it a flexible choice for organizations looking to enhance their data analytics capabilities.
3. Microsoft Azure Text Analytics
Microsoft Azure’s Text Analytics service provides a suite of NLP features, including entity recognition, sentiment analysis, and language detection. This tool is particularly useful for healthcare organizations that need to process large volumes of clinical notes quickly. By integrating Azure Text Analytics into their workflows, providers can gain insights into patient sentiment and treatment effectiveness.
4. Clinithink CLiX
Clinithink’s CLiX is specifically designed for healthcare applications, focusing on the extraction of clinical concepts from free-text notes. With its advanced algorithms, CLiX can convert unstructured clinical data into structured information, facilitating better data management and analysis. This tool is particularly valuable for clinical research and quality improvement initiatives.
5. Amazon Comprehend Medical
Amazon Comprehend Medical uses machine learning to extract relevant medical information from clinical notes. It can identify medical conditions, medications, and treatment plans, making it an essential tool for healthcare providers looking to leverage their clinical data for improved patient outcomes. Its integration with other AWS services allows for scalability and flexibility in data management.
Implementation Considerations
When selecting an NLP tool for analyzing clinical notes, healthcare organizations should consider several factors:
- Integration Capabilities: The selected tool should seamlessly integrate with existing electronic health record (EHR) systems to ensure a smooth workflow.
- Customization: The ability to customize the tool to meet specific organizational needs can enhance its effectiveness.
- Compliance and Security: Given the sensitive nature of healthcare data, tools must comply with HIPAA and other regulations to ensure data privacy and security.
- Scalability: As healthcare organizations grow, their data needs will evolve. Choosing a scalable solution can accommodate future demands.
Conclusion
The integration of NLP tools in healthcare is revolutionizing the way clinical notes are analyzed. By utilizing AI-driven products such as IBM Watson, Google Cloud NLP, Microsoft Azure Text Analytics, Clinithink CLiX, and Amazon Comprehend Medical, healthcare organizations can enhance their data analysis capabilities. As the landscape of healthcare continues to evolve, the adoption of these tools will be crucial in driving improved patient outcomes and operational efficiency.
Keyword: natural language processing healthcare tools