Optimizing Air Traffic Management with Machine Learning Algorithms
Topic: AI Self Improvement Tools
Industry: Aerospace and Defense
Discover how machine learning algorithms enhance air traffic management systems by optimizing efficiency safety and decision-making in the aerospace sector
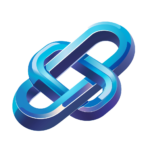
Machine Learning Algorithms Optimizing Air Traffic Management Systems
Introduction to AI in Aerospace and Defense
In recent years, the aerospace and defense sectors have witnessed a transformative shift towards the integration of artificial intelligence (AI) and machine learning (ML) technologies. These advancements are particularly evident in air traffic management systems, where optimizing operational efficiency and safety is paramount. By leveraging AI self-improvement tools, organizations can enhance decision-making processes, reduce human error, and streamline air traffic operations.
The Role of Machine Learning in Air Traffic Management
Machine learning algorithms are pivotal in analyzing vast amounts of data generated by air traffic systems. These algorithms can identify patterns, predict traffic flows, and recommend optimal routing for aircraft. As air traffic continues to grow, the need for efficient management systems becomes increasingly critical. ML algorithms can assist in:
1. Predictive Analytics
Predictive analytics powered by machine learning can forecast air traffic volumes and potential congestion points. By analyzing historical data and real-time inputs, systems can anticipate busy periods and suggest preventive measures, such as adjusting flight schedules or rerouting aircraft to avoid delays.
2. Real-Time Decision Making
AI-driven tools can facilitate real-time decision-making by providing air traffic controllers with actionable insights. For instance, advanced algorithms can process live data feeds from radar and satellite systems, enabling controllers to make informed decisions quickly, thereby enhancing safety and efficiency.
3. Automated Conflict Resolution
Machine learning can automate conflict resolution by predicting potential aircraft collisions and suggesting corrective actions. By continuously learning from past incidents and near-misses, these systems can improve their accuracy and response time, significantly reducing the risk of accidents.
Examples of AI-Driven Tools in Air Traffic Management
Several AI-driven products and tools are currently making waves in the air traffic management landscape. Here are a few noteworthy examples:
1. NASA’s Airspace Technology Demonstration (ATD)
NASA’s ATD program utilizes machine learning algorithms to enhance air traffic management capabilities. The program focuses on improving traffic flow and reducing delays through predictive modeling and real-time data analysis. By employing AI, ATD aims to create a more efficient airspace system.
2. Airbus’ Skywise
Airbus has developed Skywise, an open data platform that harnesses machine learning to optimize aircraft operations and maintenance. By analyzing data from various sources, Skywise can predict maintenance needs and improve flight scheduling, ultimately enhancing overall air traffic management.
3. Thales’ Smart Airport Solutions
Thales offers a range of smart airport solutions that incorporate machine learning to optimize air traffic management. Their systems analyze real-time data to improve resource allocation, streamline airport operations, and enhance passenger experience, showcasing the potential of AI in the aerospace sector.
Challenges and Considerations
While the benefits of machine learning in air traffic management are substantial, there are challenges to consider. Data privacy and security are paramount, as sensitive information is often involved. Additionally, integrating AI systems with existing infrastructure requires careful planning and investment. Organizations must also ensure that human operators are adequately trained to work alongside AI tools, fostering a collaborative environment.
Conclusion
The integration of machine learning algorithms into air traffic management systems presents a significant opportunity for the aerospace and defense industries. By adopting AI self-improvement tools, organizations can enhance operational efficiency, improve safety, and better manage the complexities of modern air traffic. As technology continues to evolve, the potential for AI in this sector is boundless, paving the way for a safer and more efficient future in aviation.
Keyword: machine learning air traffic management