Overcoming AI Hallucinations in Medical Research Best Practices
Topic: AI News Tools
Industry: Research and Development
Discover best practices for overcoming AI hallucinations in medical research and ensure accurate insights for improved patient outcomes in 2025.
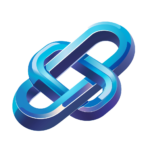
Overcoming AI Hallucinations in Medical Research: Best Practices for 2025
Understanding AI Hallucinations
Artificial Intelligence (AI) has revolutionized the landscape of medical research, offering unprecedented capabilities in data analysis, predictive modeling, and patient care. However, one of the significant challenges that researchers face is the phenomenon known as AI hallucinations—instances where AI systems generate outputs that are not grounded in reality. These inaccuracies can lead to misguided conclusions and potentially harmful decisions in clinical settings.
The Implications of AI Hallucinations in Medical Research
In the context of medical research, AI hallucinations can have severe implications. Misinterpretations of data can result in ineffective treatments, misdiagnoses, and a waste of resources. As AI continues to integrate into research and development processes, it is crucial to address these hallucinations to ensure the reliability of AI-generated insights.
Best Practices for Mitigating AI Hallucinations
To combat the challenge of AI hallucinations, researchers and developers must adopt best practices that enhance the reliability and accuracy of AI tools. Here are several strategies to consider:
1. Rigorous Data Validation
Ensuring the quality of input data is paramount. Researchers should implement robust data validation processes to filter out inaccuracies before feeding data into AI systems. Tools like DataRobot offer automated data quality checks that can help identify and rectify potential issues in datasets.
2. Continuous Model Training
AI models should not be static; they require continuous training with updated datasets to adapt to new information and reduce the likelihood of hallucinations. Utilizing platforms such as Google Cloud AI allows researchers to regularly update their models with the latest research findings and clinical data.
3. Implementing Explainable AI (XAI)
Explainable AI tools, such as H2O.ai, provide transparency in AI decision-making processes. By understanding how AI arrives at certain conclusions, researchers can better assess the validity of the outputs and identify any hallucinations that may arise.
4. Collaboration with Domain Experts
Integrating insights from medical professionals and domain experts can significantly enhance the accuracy of AI outputs. Collaborative platforms like IBM Watson for Health facilitate the convergence of AI capabilities with expert knowledge, ensuring that AI-generated insights are clinically relevant and grounded in real-world applications.
5. Establishing Feedback Loops
Creating feedback mechanisms where researchers can report inaccuracies or unexpected outputs is essential for refining AI systems. Tools such as Microsoft Azure Machine Learning allow for the integration of user feedback, which can be used to improve the model’s performance over time.
AI Tools and Products for Medical Research
Several AI-driven products have emerged as valuable assets in the medical research landscape. These tools not only aid in data analysis but also help mitigate the risks associated with AI hallucinations:
1. DeepMind Health
DeepMind Health leverages advanced machine learning techniques to analyze medical images and provide insights that can assist in diagnosis. Its focus on accuracy and reliability makes it a leading tool in the fight against AI hallucinations.
2. Tempus
Tempus utilizes AI to analyze clinical and molecular data, providing oncologists with actionable insights. By continuously updating its algorithms with new data, Tempus minimizes the risk of hallucinations in cancer research.
3. PathAI
PathAI specializes in pathology, using AI to assist pathologists in diagnosing diseases accurately. Its commitment to improving diagnostic accuracy helps reduce the incidence of AI hallucinations in pathology reports.
Conclusion
As we look toward 2025, overcoming AI hallucinations in medical research is not just a technical challenge but a critical imperative for ensuring patient safety and advancing healthcare outcomes. By implementing best practices and utilizing advanced AI tools, researchers can harness the full potential of artificial intelligence while minimizing the risks associated with inaccurate outputs. The future of medical research relies on a collaborative approach that integrates AI technology with human expertise, ensuring that we move forward with confidence and precision.
Keyword: overcoming AI hallucinations in research