Predictive Analytics and AI for Supply Chain Risk Management
Topic: AI Collaboration Tools
Industry: Logistics and Supply Chain
Discover how predictive analytics and AI enhance supply chain risk management with insights tools and strategies to improve decision-making and operational efficiency
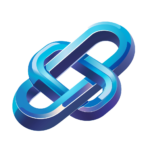
Predictive Analytics and AI: Collaborative Approaches to Supply Chain Risk Management
The Importance of Supply Chain Risk Management
In today’s fast-paced business environment, effective supply chain risk management is crucial for maintaining operational efficiency and ensuring customer satisfaction. Companies face various risks, including demand fluctuations, geopolitical uncertainties, and supply disruptions. To navigate these challenges, businesses are increasingly turning to predictive analytics and artificial intelligence (AI) to enhance their risk management strategies.
Understanding Predictive Analytics in Supply Chain
Predictive analytics involves using historical data, statistical algorithms, and machine learning techniques to identify the likelihood of future outcomes. In the context of supply chain management, predictive analytics can help organizations anticipate potential disruptions and mitigate risks before they escalate.
Key Benefits of Predictive Analytics
- Enhanced Decision-Making: By providing insights into potential risks and trends, predictive analytics allows supply chain managers to make informed decisions.
- Improved Inventory Management: Companies can optimize inventory levels by predicting demand patterns, reducing excess stock and minimizing stockouts.
- Proactive Risk Mitigation: Predictive models can identify vulnerabilities in the supply chain, enabling organizations to develop contingency plans.
The Role of AI in Supply Chain Risk Management
Artificial intelligence complements predictive analytics by automating processes, analyzing vast amounts of data, and providing real-time insights. The integration of AI into supply chain operations can significantly enhance risk management capabilities.
AI-Driven Tools for Logistics and Supply Chain
Several AI-driven tools and products are available to help organizations effectively manage supply chain risks:
1. IBM Watson Supply Chain
IBM Watson Supply Chain leverages AI to provide insights into supply chain operations. The platform uses machine learning to analyze data from various sources, enabling businesses to predict disruptions and optimize their supply chain processes. By utilizing Watson, companies can enhance visibility and responsiveness in their supply chains.
2. Llamasoft Supply Chain Guru
Llamasoft’s Supply Chain Guru is a powerful tool that combines AI and advanced analytics to model supply chain scenarios. It allows organizations to simulate different risk scenarios, assess their impact, and develop strategies to mitigate potential disruptions. This tool is particularly useful for companies looking to enhance their strategic planning capabilities.
3. ClearMetal
ClearMetal offers an AI-driven inventory optimization platform that provides end-to-end visibility across the supply chain. By analyzing data from various touchpoints, ClearMetal helps companies forecast demand more accurately, reducing the risk of overstocking or stockouts. This tool is essential for organizations looking to streamline their inventory management processes.
4. Resilinc
Resilinc is a supply chain risk management platform that uses AI to monitor and assess supply chain risks in real-time. The platform provides alerts and insights related to potential disruptions, allowing companies to respond proactively. Resilinc’s capabilities are particularly beneficial for organizations operating in industries with high volatility.
Implementing AI Collaboration Tools
To successfully implement AI collaboration tools in supply chain risk management, organizations should consider the following steps:
1. Assess Current Capabilities
Before integrating AI tools, businesses should evaluate their existing supply chain processes and identify areas for improvement. Understanding current capabilities will help organizations select the most suitable AI solutions.
2. Invest in Data Quality
High-quality data is essential for accurate predictive analytics and AI insights. Organizations should invest in data management practices to ensure that they have access to reliable and relevant information.
3. Foster a Culture of Collaboration
Successful implementation of AI tools requires collaboration across departments. Encouraging cross-functional teams to work together will enhance the effectiveness of AI-driven solutions in managing supply chain risks.
Conclusion
As supply chains become increasingly complex, the need for effective risk management strategies is more critical than ever. Predictive analytics and AI offer powerful collaborative approaches to addressing these challenges. By leveraging AI-driven tools, organizations can enhance their risk management capabilities, improve decision-making, and ultimately drive operational efficiency. The future of supply chain risk management lies in the successful integration of these innovative technologies.
Keyword: supply chain risk management AI