Predictive Analytics and AI Transforming Risk Assessment in Insurance
Topic: AI Business Tools
Industry: Insurance
Discover how predictive analytics and AI are transforming risk assessment in insurance with enhanced accuracy real-time insights and cost efficiency
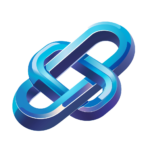
Predictive Analytics and AI: Shaping the Future of Risk Assessment
Understanding Predictive Analytics in Insurance
Predictive analytics refers to the use of statistical algorithms and machine learning techniques to identify the likelihood of future outcomes based on historical data. In the insurance sector, this approach is transforming how companies assess risk, underwrite policies, and manage claims. By leveraging vast amounts of data, insurers can make informed decisions, optimize pricing strategies, and enhance customer satisfaction.
The Role of Artificial Intelligence
Artificial Intelligence (AI) plays a pivotal role in enhancing predictive analytics capabilities. By automating data analysis and improving the accuracy of predictions, AI empowers insurers to better understand risk profiles and anticipate future events. This shift not only streamlines operations but also fosters a more proactive approach to risk management.
Key Benefits of AI in Risk Assessment
- Enhanced Accuracy: AI algorithms can analyze complex datasets far more efficiently than traditional methods, resulting in more precise risk evaluations.
- Real-Time Insights: AI-driven tools can provide real-time analytics, allowing insurers to swiftly react to emerging risks and market changes.
- Cost Efficiency: By automating routine processes, AI reduces operational costs and allows human resources to focus on strategic initiatives.
Implementing AI in Risk Assessment
To effectively implement AI in risk assessment, insurance companies should consider the following steps:
1. Data Collection and Integration
Gathering comprehensive data is the foundation of predictive analytics. Insurers should integrate various data sources, including customer demographics, claim history, and external factors such as economic indicators and weather patterns.
2. Selecting the Right AI Tools
Choosing the appropriate AI tools is critical for successful implementation. Some notable AI-driven products in the insurance sector include:
- IBM Watson: This powerful AI platform can analyze large datasets and provide insights into risk assessment and fraud detection.
- Zest AI: Zest AI specializes in machine learning models that help insurers make better underwriting decisions by predicting borrower behavior more accurately.
- Shift Technology: This AI solution focuses on claims automation and fraud detection, enabling insurers to streamline their claims processes while minimizing losses.
3. Continuous Learning and Adaptation
AI systems thrive on continuous learning. Insurers should regularly update their models with new data and insights to ensure that their predictive capabilities remain relevant and accurate.
Case Studies: AI in Action
Example 1: Progressive Insurance
Progressive Insurance utilizes AI to enhance its pricing models. By analyzing customer data and driving behavior through telematics, Progressive can offer personalized premiums that reflect individual risk profiles, leading to increased customer satisfaction and retention.
Example 2: Lemonade
Lemonade, a tech-driven insurance company, employs AI to streamline its claims process. Through an AI chatbot, customers can file claims instantly, and the system can assess and approve claims in minutes, significantly reducing processing time and improving customer experience.
Conclusion
The integration of predictive analytics and AI in risk assessment is revolutionizing the insurance industry. By adopting these advanced technologies, insurers can enhance their decision-making processes, improve operational efficiency, and ultimately deliver better services to their customers. As the landscape continues to evolve, those who embrace AI-driven solutions will be well-positioned to lead in the competitive insurance market.
Keyword: Predictive analytics in insurance