Predictive Analytics and NLP for Supply Chain Disruption Forecasting
Topic: AI Language Tools
Industry: Logistics and Supply Chain Management
Discover how predictive analytics and natural language processing enhance supply chain management by forecasting disruptions and optimizing operations for success.
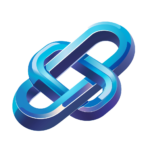
Predictive Analytics Meets Natural Language: Forecasting Supply Chain Disruptions
The Intersection of AI and Supply Chain Management
In today’s rapidly evolving business landscape, organizations are increasingly turning to artificial intelligence (AI) to enhance their logistics and supply chain management processes. The integration of predictive analytics with natural language processing (NLP) is revolutionizing how companies forecast and mitigate supply chain disruptions. By leveraging these advanced technologies, businesses can gain deeper insights, improve decision-making, and ultimately drive operational efficiency.Understanding Predictive Analytics in Supply Chain Management
Predictive analytics involves the use of statistical algorithms and machine learning techniques to analyze historical data and predict future outcomes. In the context of supply chain management, this means identifying potential disruptions before they occur, allowing organizations to proactively address issues and optimize their operations.The Role of Natural Language Processing
Natural language processing is a branch of AI that enables machines to understand and interpret human language. When applied to supply chain management, NLP can analyze vast amounts of unstructured data, such as customer feedback, news articles, and social media posts, to identify trends and sentiments that may indicate upcoming disruptions.Implementing AI-Driven Solutions
To effectively harness the power of predictive analytics and NLP, organizations must implement AI-driven solutions tailored to their specific needs. Here are several tools and products that can facilitate this integration:1. IBM Watson Supply Chain
IBM Watson Supply Chain utilizes AI and machine learning to provide real-time visibility into supply chain operations. By analyzing data from various sources, including IoT devices and external market trends, it can predict potential disruptions and recommend actions to mitigate risks.2. SAP Integrated Business Planning (IBP)
SAP IBP combines predictive analytics with planning tools to enhance supply chain forecasting. Its machine learning capabilities analyze historical data and market signals, while its NLP features allow users to query data using natural language, making it easier for decision-makers to access insights.3. Llamasoft Supply Chain Guru
Llamasoft’s Supply Chain Guru offers advanced analytics and modeling capabilities. By integrating predictive analytics, it helps businesses simulate various scenarios and assess the impact of potential disruptions. The platform’s NLP features enable users to extract insights from complex datasets through simple queries.4. ClearMetal
ClearMetal provides an AI-driven inventory management solution that leverages predictive analytics to optimize stock levels and reduce excess inventory. Its NLP capabilities allow users to monitor customer sentiment and demand fluctuations, ensuring that supply chains remain agile and responsive.Case Studies: Successful Implementations
Several organizations have successfully implemented AI-driven tools to enhance their supply chain resilience:Example 1: Unilever
Unilever utilized IBM Watson to analyze social media data and identify shifts in consumer sentiment. By predicting changes in demand, the company was able to adjust its supply chain strategy, ensuring product availability and minimizing disruptions.Example 2: Coca-Cola
Coca-Cola implemented SAP IBP to improve its forecasting accuracy. The integration of predictive analytics allowed the company to anticipate supply chain challenges, resulting in better inventory management and reduced operational costs.The Future of AI in Supply Chain Management
As AI technologies continue to advance, the potential for predictive analytics and NLP in supply chain management will only grow. Organizations that embrace these innovations will be better equipped to navigate the complexities of global supply chains, respond to market changes, and maintain a competitive edge.Conclusion
The convergence of predictive analytics and natural language processing is transforming supply chain management. By implementing AI-driven tools, organizations can enhance their forecasting capabilities, mitigate disruptions, and optimize their operations. As the landscape continues to evolve, businesses that invest in these technologies will position themselves for long-term success in an increasingly complex and dynamic environment.Keyword: AI predictive analytics supply chain