Predictive Analytics in Banking Enhancing Customer Experience
Topic: AI Research Tools
Industry: Finance and Banking
Discover how predictive analytics powered by AI is transforming banking by anticipating customer needs enhancing services and boosting satisfaction
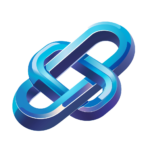
Predictive Analytics in Banking: Using AI to Anticipate Customer Needs
Understanding Predictive Analytics in the Banking Sector
Predictive analytics is transforming the banking industry by leveraging artificial intelligence (AI) to analyze historical data, identify patterns, and forecast future trends. This capability enables financial institutions to anticipate customer needs and enhance their service offerings, ultimately leading to improved customer satisfaction and loyalty.
The Role of AI in Predictive Analytics
AI algorithms can process vast amounts of data at unprecedented speeds, allowing banks to gain insights that were previously unattainable. By implementing machine learning models, banks can predict customer behaviors, assess credit risks, and tailor financial products to individual preferences.
Key AI Techniques in Predictive Analytics
- Machine Learning: Algorithms that learn from data to make predictions without being explicitly programmed.
- Natural Language Processing (NLP): Enables the analysis of customer interactions and sentiments from various communication channels.
- Neural Networks: Mimics the human brain to recognize complex patterns in data, useful for fraud detection and risk assessment.
Implementing AI-Driven Predictive Analytics Tools
To harness the power of predictive analytics, banks can utilize several AI-driven tools and platforms. Here are some notable examples:
1. IBM Watson
IBM Watson offers robust analytics capabilities that help banks analyze customer data and predict future behaviors. By integrating Watson into their systems, financial institutions can create personalized banking experiences and improve customer engagement.
2. SAS Analytics
SAS provides advanced analytics solutions that include predictive modeling and machine learning. With SAS, banks can develop risk management strategies and enhance customer relationship management (CRM) by predicting customer needs based on historical data.
3. Salesforce Einstein
Salesforce Einstein is an AI layer within the Salesforce platform that allows banks to deliver personalized experiences. By utilizing predictive analytics, banks can anticipate customer inquiries and offer tailored financial products, enhancing overall service quality.
4. Zest AI
Zest AI specializes in credit underwriting using machine learning. By analyzing a broader range of data points, Zest AI helps banks make more accurate lending decisions, ultimately reducing risk and improving customer access to credit.
Case Studies: Successful Implementation of Predictive Analytics
Case Study 1: JPMorgan Chase
JPMorgan Chase utilizes AI-driven predictive analytics to enhance its fraud detection capabilities. By analyzing transaction patterns in real-time, the bank can identify and mitigate fraudulent activities, protecting both the institution and its customers.
Case Study 2: Bank of America
Bank of America employs predictive analytics to improve customer service through its virtual assistant, Erica. By analyzing user interactions, Erica can provide personalized financial advice and recommendations, anticipating customer needs before they arise.
Challenges and Considerations
While the benefits of predictive analytics are substantial, banks must also navigate challenges such as data privacy concerns, regulatory compliance, and the need for skilled personnel to manage AI systems. It is crucial for financial institutions to establish robust data governance frameworks and invest in training to maximize the potential of AI-driven analytics.
Conclusion
Predictive analytics powered by AI is revolutionizing the banking sector by enabling institutions to anticipate customer needs effectively. By leveraging advanced tools and technologies, banks can enhance their service offerings, reduce risks, and ultimately drive customer satisfaction. As the landscape continues to evolve, embracing predictive analytics will be essential for banks aiming to stay competitive in an increasingly data-driven world.
Keyword: AI predictive analytics in banking