Predictive Analytics in Home Health to Reduce Hospital Readmissions
Topic: AI Home Tools
Industry: Home Healthcare
Discover how predictive analytics and AI in home health can reduce hospital readmissions enhance patient care and improve health outcomes for at-risk patients
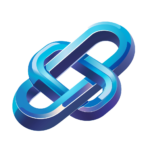
Predictive Analytics in Home Health: Using AI to Prevent Hospital Readmissions
The Role of Predictive Analytics in Home Healthcare
In recent years, the healthcare industry has witnessed a significant shift towards the integration of artificial intelligence (AI) and predictive analytics, particularly in home health settings. With an increasing emphasis on patient-centered care, the need for effective tools to monitor patient health and prevent hospital readmissions has never been more critical. Predictive analytics leverages data to forecast patient outcomes, enabling healthcare providers to intervene proactively and enhance patient care.
Understanding Hospital Readmissions
Hospital readmissions are a pressing concern for healthcare providers, as they not only indicate potential gaps in patient care but also contribute to increased healthcare costs. According to the Centers for Medicare & Medicaid Services (CMS), nearly 20% of Medicare patients are readmitted within 30 days of discharge, often due to complications that could have been prevented with timely interventions. By utilizing predictive analytics, healthcare providers can identify at-risk patients and implement strategies to mitigate these risks.
How AI Can Be Implemented in Home Health
AI technologies can be seamlessly integrated into home health care through various tools and platforms designed to enhance patient monitoring and engagement. The implementation of AI-driven solutions can be categorized into three key areas:
1. Data Collection and Analysis
AI systems can gather and analyze vast amounts of patient data, including medical history, medication adherence, and lifestyle factors. This data can be processed using machine learning algorithms to identify patterns and predict potential health issues. For example, platforms like Health Catalyst utilize AI to analyze patient data and provide actionable insights to healthcare providers, enabling them to make informed decisions about patient care.
2. Remote Monitoring Tools
Remote monitoring devices equipped with AI capabilities can track vital signs and other health metrics in real time. Tools such as Wearable Health Technology (e.g., Fitbit, Apple Watch) can monitor heart rates, activity levels, and even sleep patterns. These devices can alert healthcare providers to any concerning changes in a patient’s condition, allowing for timely interventions that can prevent hospital readmissions.
3. Patient Engagement and Support
AI-driven applications can enhance patient engagement by providing personalized care plans and reminders for medication adherence. For instance, MyTherapy is an app that helps patients manage their medications and track their health metrics. By sending reminders and providing educational resources, these applications empower patients to take an active role in their health, ultimately reducing the likelihood of complications that lead to readmissions.
Case Studies and Success Stories
Several healthcare organizations have successfully implemented AI-driven predictive analytics to reduce hospital readmissions. For example, Mount Sinai Health System in New York utilized an AI model to predict which patients were at the highest risk of readmission. By focusing their resources on these patients, they were able to reduce readmission rates by 20% within a year.
Another notable example is the use of the CarePredict system, which employs AI to monitor seniors in their homes. By analyzing daily activities and identifying deviations from normal behavior, CarePredict can alert caregivers to potential health issues before they escalate, thereby preventing unnecessary hospital visits.
Challenges and Considerations
While the benefits of predictive analytics in home health are substantial, there are challenges that organizations must address. Data privacy and security are paramount concerns, as sensitive patient information is involved. Additionally, healthcare providers must ensure that their staff is adequately trained to utilize these AI tools effectively.
Conclusion
As the healthcare landscape continues to evolve, the integration of predictive analytics and AI in home health care represents a transformative opportunity to improve patient outcomes and reduce hospital readmissions. By leveraging data-driven insights, healthcare providers can proactively manage patient care, ultimately leading to a more efficient and effective healthcare system. Investing in AI-driven tools and fostering a culture of innovation will be essential for organizations looking to thrive in this new era of healthcare.
Keyword: predictive analytics home health care