Predictive Analytics in Retail Using AI for Consumer Trends
Topic: AI Self Improvement Tools
Industry: Retail and E-commerce
Discover how predictive analytics powered by AI can transform retail by forecasting consumer trends optimizing inventory and enhancing customer experiences
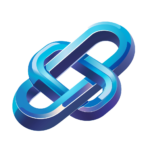
Predictive Analytics in Retail: Using AI to Forecast Consumer Trends
The Role of Predictive Analytics in Retail
In the fast-paced world of retail and e-commerce, understanding consumer behavior is crucial for maintaining a competitive edge. Predictive analytics, powered by artificial intelligence (AI), enables businesses to forecast consumer trends effectively. By analyzing historical data and identifying patterns, retailers can make informed decisions that enhance customer experiences and optimize inventory management.
Implementing AI in Predictive Analytics
Integrating AI into predictive analytics involves several key steps:
1. Data Collection
The first step is to gather data from various sources, including sales records, customer interactions, and social media. This data serves as the foundation for any predictive model.
2. Data Processing
Once collected, the data must be cleaned and processed to ensure accuracy. This involves removing duplicates, filling in missing values, and normalizing data formats.
3. Model Development
Using machine learning algorithms, businesses can develop predictive models that analyze historical data and identify trends. These models can range from simple linear regressions to more complex neural networks, depending on the specific needs of the retailer.
4. Implementation and Monitoring
After developing the model, it can be implemented into business operations. Continuous monitoring and refinement are necessary to ensure the model remains accurate and relevant as consumer behavior evolves.
AI-Driven Tools for Retail Predictive Analytics
Several AI-driven tools can assist retailers in leveraging predictive analytics:
1. Salesforce Einstein
Salesforce Einstein is an AI-powered analytics tool that helps retailers understand customer preferences and predict future buying behaviors. By integrating with existing Salesforce platforms, it provides actionable insights that can enhance marketing strategies and improve customer engagement.
2. IBM Watson Analytics
IBM Watson Analytics offers advanced data analysis capabilities, enabling retailers to uncover hidden patterns in their data. With natural language processing features, users can ask questions and receive insights in real-time, making it easier to adapt to changing consumer trends.
3. Google Cloud AI
Google Cloud AI provides machine learning tools that allow retailers to build custom predictive models. Its AutoML feature enables users to train models without extensive coding knowledge, making advanced analytics accessible to businesses of all sizes.
4. SAS Analytics
SAS Analytics offers a suite of tools designed for predictive modeling and forecasting. It provides robust data visualization capabilities, allowing retailers to present insights in a way that is easily digestible for decision-makers.
Case Studies of Successful Implementation
Case Study: Walmart
Walmart utilizes predictive analytics to optimize its inventory management. By analyzing sales data from various locations, Walmart can forecast demand for specific products, ensuring that shelves are stocked appropriately and reducing waste.
Case Study: Amazon
Amazon leverages predictive analytics to enhance its recommendation engine. By analyzing previous purchases and browsing behavior, Amazon can suggest products that consumers are likely to buy, significantly increasing conversion rates.
Conclusion
As the retail landscape continues to evolve, the importance of predictive analytics cannot be overstated. By implementing AI-driven tools, retailers can gain valuable insights into consumer trends, allowing them to make data-driven decisions that enhance customer satisfaction and drive sales. Embracing these technologies is no longer optional; it is essential for success in the modern retail environment.
Keyword: AI predictive analytics in retail