Predictive Analytics in Retail Using AI for Demand Forecasting
Topic: AI Data Tools
Industry: Retail and E-commerce
Discover how predictive analytics and AI can forecast retail trends optimize inventory and enhance customer experiences for better business outcomes
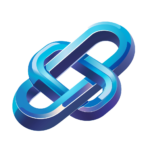
Predictive Analytics: Using AI to Forecast Retail Trends and Demand
Understanding Predictive Analytics in Retail
Predictive analytics is a branch of advanced analytics that uses historical data, machine learning, and statistical algorithms to identify the likelihood of future outcomes. In the retail and e-commerce sectors, this approach can help businesses anticipate customer behavior, optimize inventory levels, and ultimately drive sales. By leveraging artificial intelligence (AI), retailers can gain deeper insights into market trends and consumer demand, making informed decisions that enhance operational efficiency and customer satisfaction.
Implementing AI in Predictive Analytics
To successfully implement AI-driven predictive analytics in retail, companies must follow a structured approach that includes data collection, model development, and continuous improvement. Here are key steps in the process:
1. Data Collection
The foundation of predictive analytics lies in data. Retailers should gather data from various sources, including point-of-sale systems, customer relationship management (CRM) platforms, and social media channels. This data can include sales figures, customer demographics, purchasing patterns, and market trends.
2. Data Processing and Cleaning
Once data is collected, it must be cleaned and processed to ensure accuracy. This step involves removing duplicates, correcting errors, and standardizing formats to create a reliable dataset for analysis.
3. Model Development
After preparing the data, retailers can develop predictive models using machine learning algorithms. These models can analyze historical data to identify patterns and correlations that inform future predictions. Popular algorithms include regression analysis, decision trees, and neural networks.
4. Implementation and Monitoring
Once models are developed, they should be integrated into existing retail systems. Continuous monitoring and refinement of these models are crucial to adapt to changing market conditions and consumer behaviors.
Examples of AI-Driven Tools for Retail Predictive Analytics
Several AI-driven tools and products are available to assist retailers in leveraging predictive analytics effectively. Here are some notable examples:
1. Salesforce Einstein
Salesforce Einstein is an AI-powered analytics tool that enables retailers to analyze customer data and predict future buying behaviors. By integrating seamlessly with Salesforce’s CRM platform, it allows businesses to create personalized marketing campaigns and optimize inventory management based on predicted demand.
2. IBM Watson Analytics
IBM Watson offers advanced analytics capabilities that utilize natural language processing and machine learning to provide actionable insights. Retailers can use Watson to forecast sales trends, analyze customer sentiment, and identify potential market disruptions.
3. Google Cloud AI
Google Cloud AI provides a suite of machine learning tools that can be customized for retail applications. Retailers can utilize these tools to build predictive models that forecast demand, optimize pricing strategies, and enhance customer experiences through personalized recommendations.
4. SAS Analytics
SAS Analytics offers a comprehensive platform for predictive analytics in retail. With capabilities for data mining, forecasting, and optimization, retailers can leverage SAS to improve supply chain efficiency and enhance customer engagement through targeted marketing strategies.
Conclusion: The Future of Retail with Predictive Analytics
The integration of predictive analytics powered by AI is transforming the retail landscape. By harnessing the power of data, retailers can make informed decisions that not only improve operational efficiency but also enhance the customer experience. As technology continues to evolve, those who adopt AI-driven predictive analytics will be well-positioned to stay ahead of market trends and meet the ever-changing demands of consumers.
Keyword: AI predictive analytics retail trends