Predictive Analytics Transforming Customer Care in Utilities
Topic: AI Customer Support Tools
Industry: Energy and Utilities
Discover how predictive analytics is transforming customer care in utilities by anticipating needs and resolving issues proactively for enhanced satisfaction and loyalty
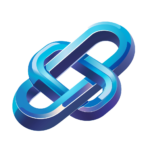
Predictive Analytics: The Future of Proactive Customer Care in Utilities
In the rapidly evolving landscape of energy and utilities, organizations are increasingly turning to artificial intelligence (AI) to enhance customer support services. Predictive analytics, a key component of AI, is transforming how utilities anticipate customer needs and streamline service delivery. By leveraging data-driven insights, companies can not only improve customer satisfaction but also foster long-term loyalty. This article explores how predictive analytics can be implemented in the utilities sector and highlights specific AI-driven tools that can facilitate this transition.
The Role of Predictive Analytics in Customer Care
Predictive analytics involves using historical data, machine learning algorithms, and statistical techniques to forecast future events. In the context of customer care within the utilities sector, this means anticipating customer inquiries, identifying potential issues before they escalate, and personalizing service offerings. By utilizing predictive analytics, utilities can shift from reactive to proactive customer care, ultimately enhancing the customer experience.
Anticipating Customer Needs
One of the primary advantages of predictive analytics is its ability to analyze customer behavior patterns. For example, utilities can track usage data to identify trends, such as increased energy consumption during specific seasons. By predicting these peaks, companies can proactively reach out to customers with energy-saving tips or special programs, thus preventing potential service disruptions and enhancing customer satisfaction.
Identifying and Resolving Issues
Predictive analytics can also be instrumental in identifying potential service issues before they impact customers. By analyzing data from smart meters and IoT devices, utilities can detect anomalies that may indicate equipment failures or outages. For instance, if a particular region shows a sudden spike in energy usage, this could signal an underlying problem that requires immediate attention. By addressing these issues proactively, utilities can minimize downtime and maintain customer trust.
Implementing AI-Driven Tools
To harness the power of predictive analytics, utilities can employ a range of AI-driven tools and platforms. Below are some notable examples:
1. IBM Watson
IBM Watson offers advanced analytics capabilities that can be tailored to the needs of energy and utility companies. By integrating Watson’s AI with existing customer relationship management (CRM) systems, utilities can gain insights into customer preferences and behaviors, enabling personalized communication and service delivery.
2. Salesforce Einstein
Salesforce Einstein is an AI-powered analytics tool that helps organizations predict customer behavior and automate responses. In the utilities sector, this tool can be used to analyze customer data and provide recommendations for service improvements, ensuring that customer inquiries are addressed promptly and effectively.
3. Google Cloud AI
Google Cloud AI offers machine learning tools that can process vast amounts of data in real-time. Utilities can utilize these capabilities to analyze consumption patterns and forecast demand, allowing for better resource allocation and customer engagement strategies.
Case Studies: Success Stories in Predictive Analytics
Several utilities have successfully implemented predictive analytics to enhance customer care:
Case Study 1: Pacific Gas and Electric (PG&E)
PG&E has leveraged predictive analytics to improve its customer service operations. By analyzing historical data, the company can forecast outage events and proactively communicate with affected customers, providing estimated restoration times and updates. This initiative has significantly improved customer satisfaction ratings.
Case Study 2: Enel
Enel, a multinational energy company, has integrated AI-driven analytics into its customer service strategy. By utilizing machine learning algorithms, Enel can predict customer needs and tailor its offerings accordingly. This approach has not only enhanced customer engagement but also optimized operational efficiency.
Conclusion
As the energy and utilities sector continues to evolve, the integration of predictive analytics and AI-driven tools will play a pivotal role in shaping the future of customer care. By adopting these technologies, utilities can proactively address customer needs, enhance service delivery, and ultimately drive customer loyalty. Embracing predictive analytics is not just a technological advancement; it is a strategic imperative for utilities aiming to thrive in a competitive landscape.
Keyword: predictive analytics customer care utilities