Predictive Maintenance AI Reducing Trucking Fleet Downtime
Topic: AI Productivity Tools
Industry: Logistics and Transportation
Discover how AI-driven predictive maintenance is reducing downtime and enhancing efficiency for trucking fleets while saving costs and improving safety
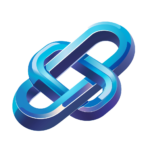
How Predictive Maintenance AI is Reducing Downtime for Trucking Fleets
The Importance of Predictive Maintenance in the Trucking Industry
In the fast-paced world of logistics and transportation, minimizing downtime is crucial for maintaining efficiency and profitability. Predictive maintenance, powered by artificial intelligence (AI), is emerging as a transformative solution for trucking fleets. By leveraging data analytics and machine learning, predictive maintenance enables fleet managers to anticipate vehicle issues before they escalate into costly breakdowns.
Understanding Predictive Maintenance
Predictive maintenance involves the use of AI algorithms to analyze data from various sources, including vehicle sensors, maintenance records, and historical performance data. This approach not only helps in identifying potential failures but also optimizes maintenance schedules, ensuring that trucks are serviced at the right time.
Key Benefits of AI-Driven Predictive Maintenance
- Reduced Downtime: By predicting when a vehicle is likely to fail, fleets can schedule maintenance proactively, thereby reducing unexpected breakdowns.
- Cost Savings: Early detection of issues can prevent costly repairs and extend the lifespan of vehicles.
- Improved Safety: Regular maintenance based on predictive analytics ensures that vehicles are safe to operate, reducing the risk of accidents.
- Enhanced Efficiency: AI-driven maintenance allows for better resource allocation, optimizing both labor and parts inventory.
Implementing AI in Predictive Maintenance
Integrating AI into predictive maintenance requires a strategic approach that encompasses data collection, analysis, and actionable insights. Here are the steps involved in implementing AI-driven predictive maintenance in trucking fleets:
1. Data Collection
Collecting data from various sources is the first step. This includes telemetry data from vehicle sensors, historical maintenance records, and external factors such as weather conditions. Tools like telematics systems can provide real-time data on vehicle performance.
2. Data Analysis
Once data is collected, AI algorithms analyze it to identify patterns and trends. Machine learning models can be trained to recognize signs of potential failures based on historical data. Platforms such as IBM Watson IoT and Microsoft Azure Machine Learning offer robust tools for data analysis.
3. Predictive Modeling
Using the insights gained from data analysis, predictive models can be developed. These models forecast when maintenance should be performed, allowing fleet managers to act before issues arise. Tools like Uptake and Predii offer specialized predictive maintenance solutions tailored for the transportation sector.
4. Implementation and Monitoring
Finally, the predictive maintenance strategy should be implemented across the fleet. Continuous monitoring is essential to refine models and improve accuracy over time. Fleet management software, such as Fleet Complete or Samsara, can facilitate the integration of predictive maintenance into daily operations.
Examples of AI-Driven Tools for Predictive Maintenance
Several AI-driven products are making significant strides in predictive maintenance for trucking fleets:
1. Geotab
Geotab offers a comprehensive telematics solution that provides insights into vehicle health and performance. Their AI-powered analytics can predict maintenance needs based on real-time data, helping fleet managers make informed decisions.
2. Zubie
Zubie provides a connected vehicle platform that monitors vehicle diagnostics and performance. Their predictive maintenance features alert fleet managers to potential issues, enabling proactive maintenance scheduling.
3. Fleetio
Fleetio is a fleet management software that incorporates predictive maintenance capabilities. It allows for tracking maintenance schedules and utilizing data analytics to predict future maintenance needs.
Conclusion
As the logistics and transportation industry continues to evolve, the integration of AI-driven predictive maintenance tools is becoming increasingly vital. By reducing downtime and enhancing operational efficiency, these technologies not only save costs but also improve safety and reliability in trucking fleets. Embracing predictive maintenance powered by AI is not just a trend; it is a strategic necessity for fleets aiming to thrive in a competitive landscape.
Keyword: AI predictive maintenance trucking fleets