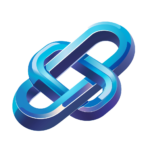
ADLN - Detailed Review
Analytics Tools
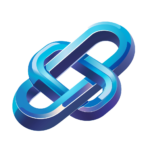
ADLN - Product Overview
ADLN.io is not an analytics tools AI-driven product, but rather a versatile customer service and communication platform. Here’s a brief overview of its primary function, target audience, and key features:
Primary Function
ADLN.io is designed to help businesses efficiently manage customer interactions across multiple channels, including websites, social media, and messaging platforms like Telegram. It consolidates all communications into a single, unified inbox, simplifying the process of tracking, responding to, and managing customer inquiries.
Target Audience
ADLN.io is suitable for a wide range of businesses, including startups, freelancers, small to medium-sized businesses, large enterprises, and non-profit organizations. It caters to various industries, such as B2B SaaS and eCommerce companies, aiming to enhance their customer service and sales operations.
Key Features
- Live Chat: Allows real-time communication between customers and support staff, significantly increasing the likelihood of conversions.
- AI-Powered Chatbot: Utilizes natural language processing to understand queries and provide accurate, relevant responses to common customer questions. The chatbot’s conversational flow and responses are highly customizable.
- Shared Inbox: Enables internal teams to communicate, prioritize tasks, and delegate work efficiently, eliminating the need to sift through scattered email threads or messages from different platforms.
- Integration: Seamlessly integrates with Telegram, social media, and other critical applications using API keys.
- Automated Messages: Allows configuration of automated messages to proactively offer assistance to website visitors.
- Customizable Branding: Enables businesses to customize the chatbot flows and responses to match their brand and ensure a consistent customer experience across channels.
- Workflow Management: Helps teams manage their tasks and work efficiently within a unified platform.
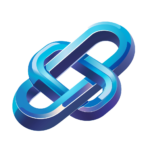
ADLN - User Interface and Experience
Given the lack of specific information about the user interface of ADLN (Advanced Distributed Learning Network) in the provided sources or the website itself, it’s challenging to provide a detailed description of its UI and user experience.
However, based on general principles of user interface design in analytics and AI-driven tools, here are some key elements that are typically important for such products:
Key Components of UI in Analytics Tools
Navigation and Accessibility
A well-organized navigation system, including clear menus, sub-menus, and possibly breadcrumb trails, is essential for helping users quickly find what they need.Search and Filtering
An easily accessible and powerful search bar with advanced search options and filters can significantly enhance the user’s ability to locate specific data or analytics results.Dashboard and Overview
A customizable dashboard provides users with an overview of important information, recent activity, and quick access to frequently used features. This helps in keeping the user informed and efficient.Intuitive Buttons and Icons
Intuitive buttons and icons that are easily recognizable and consistently used throughout the interface facilitate easy interaction with the system.Layout Design and Visual Elements
The layout should be logical and organized, with a clear hierarchy of information, proper spacing, alignment, and grouping of elements. Consistent use of colors and fonts enhances readability and visual appeal.Feedback and Notifications
Providing immediate feedback and notifications helps users understand the results of their actions, such as confirmation messages, error alerts, and progress indicators.Customization and Responsiveness
Allowing users to customize their UI, such as rearranging dashboard widgets or changing display settings, enhances flexibility and usability. Implementing responsive design ensures the UI works well on different devices and screen sizes.Ease of Use and User Experience
For an analytics tool like ADLN, ease of use is crucial. Here are some general best practices:User-Centered Design
The UI should be designed with the user in mind, involving user research, personas, and usability testing to ensure it meets user needs and preferences.Consistency and Standards
Following UI design standards and maintaining consistency across all elements ensures a cohesive and professional look and feel.Accessibility
The UI should be designed to be accessible to all users, including those with disabilities, to ensure compliance with accessibility standards and provide an inclusive user experience. Without specific details about ADLN’s UI, it is important to emphasize that a good user interface in analytics tools should balance functionality with simplicity, provide clear and intuitive interactions, and ensure a consistent and accessible experience across different devices and user needs. If you need detailed information about ADLN’s UI, it would be best to refer directly to their official documentation or contact their support team.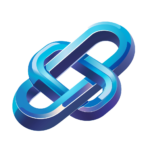
ADLN - Key Features and Functionality
Overview
ADLN, as an AI-powered content creation platform, offers a variety of features that streamline the writing process, enhance productivity, and integrate advanced AI technologies. Here are the main features and how they work:Content Generation and Rewriting
Content Rewriter
ADLN includes a feature called “Content Rewriter” that uses AI and natural language processing (NLP) to generate unique versions of existing content. This tool is particularly useful for repurposing content, avoiding plagiarism, and saving time. It allows users to create original content quickly and efficiently, which is beneficial for marketers, students, and anyone needing to produce high-quality content.Conversational Prompts and Chat
Chat Feature
The “Chat” feature leverages AI and NLP to understand complex queries and enhance creativity. This tool uses conversational prompts to help users generate content or responses quickly. It is valuable for businesses and individuals looking to improve their engagement and communication skills.Code Generation
Codex Feature
ADLN’s “Codex” feature is designed to streamline the code generation process. Whether you are a beginner or an experienced developer, Codex can generate high-quality code quickly and efficiently, making it an invaluable asset for streamlining workflows.Multilingual Support
ADLN allows users to write and generate content in over 20 different languages. This multilingual support makes it a versatile tool for users from various linguistic backgrounds, enhancing communication and content creation across different regions.Automation and Productivity Tools
Automation Features
The platform offers a range of automation tools that increase productivity and streamline workflows. These tools include features like auto-completion, summarization, and the generation of unique stories. ADLN also integrates with other tools like WordPress and Google Drive, allowing users to export content into popular formats such as PDF, Word, and TXT.SEO Optimization
SEO-Optimized Blog Drafting
ADLN includes an SEO-optimized blog drafting assistant, which helps users create content that is optimized for search engines. This feature is crucial for bloggers and marketers looking to boost their website traffic and improve site rankings.Grammar Checking and Writing Assistance
Writing Improvement Tools
The platform provides advanced grammar checking and writing assistance, improving the quality and creativity of written content. This feature is particularly helpful for college students, professionals, and anyone looking to refine their writing skills.Team Support and Collaboration
Collaboration Features
ADLN offers features that support team collaboration, including a shared inbox and integration with various applications. This facilitates efficient team communication and task management, especially beneficial for enterprise teams, digital marketers, and customer service managers.Pricing and Plans
ADLN offers tiered pricing plans to suit different user needs. The free plan allows users to write up to 2,000 words, while the Creator plan ($12.99/month) and Business plan ($99.99/month) offer higher word limits and additional features. The Enterprise plan provides unlimited content generation, custom OpenAI API access, and the ability to add managers and employees, along with fully white-label options.Conclusion
In summary, ADLN integrates AI technologies to provide a comprehensive suite of tools for content creation, code generation, and team collaboration. Its features are designed to enhance productivity, improve writing quality, and streamline workflows, making it a valuable resource for businesses and individuals alike.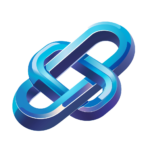
ADLN - Performance and Accuracy
Evaluation of ADLN in AI-Driven Analytics Tools
To evaluate the performance and accuracy of ADLN (Advanced Distributed Learning Network) in the Analytics Tools AI-driven product category, we need to consider several key aspects, although specific details about ADLN’s performance and accuracy may not be readily available from the provided sources or the ADLN website itself.Accuracy in AI-Driven Analytics
Accuracy in AI-driven analytics tools, such as those potentially offered by ADLN, is crucial for reliable and trustworthy outcomes. Here are some general principles that can be applied to evaluate accuracy:Data Annotation Accuracy
Accurate data annotation is essential for the efficacy of machine learning models. Techniques like hierarchical labeling, quality control, automated tools, and active learning can significantly enhance annotation accuracy, which in turn improves the model’s performance metrics.Model Validation
The accuracy of AI models can be validated through post-hoc processing, such as using accuracy monitor models that predict whether the model’s prediction is correct or not. This approach helps in estimating the true inference accuracy on a user’s dataset.Performance Considerations
Precision vs. Accuracy
In neural networks, reducing precision (the number of bits used in computations) does not necessarily reduce accuracy. In fact, using lower precision can sometimes improve accuracy and significantly reduce computational costs and hardware requirements.Real-World Deployment
The performance of AI models in real-world applications can be affected by various factors, including the quality of the input data and the specific use case. For safety-critical applications, high accuracy is paramount, and any reduction in precision must be carefully balanced against potential losses in accuracy.Limitations and Areas for Improvement
Data Quality
One of the primary limitations of any AI-driven analytics tool is the quality of the data it is trained on. Poor data quality can lead to suboptimal model performance and compromised accuracy. Ensuring high-quality, accurately annotated data is essential.Model Generalizability
AI models may not always generalize well to new, unseen data. Techniques such as ensemble methods and active learning can help improve a model’s ability to generalize and maintain high accuracy across different datasets.Computational Resources
The computational resources required to run AI models can be significant. Balancing precision and accuracy with computational efficiency is crucial for practical deployment. Using lower precision computations or specialized hardware like FPGAs can help reduce costs and improve performance.Conclusion
Without specific information on ADLN’s product, it is challenging to provide a detailed evaluation of its performance and accuracy. However, any AI-driven analytics tool should focus on ensuring high data annotation accuracy, validating model performance through rigorous testing, and balancing precision and accuracy with computational efficiency. If ADLN employs techniques such as hierarchical labeling, quality control, automated tools, and active learning, it is likely to achieve high accuracy and reliable performance in its analytics tools.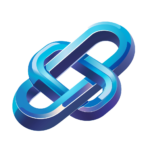
ADLN - Pricing and Plans
Pricing Structure of ADLN
The pricing structure of ADLN, an AI-driven content creation platform, is structured to cater to a variety of user needs, ranging from free to enterprise plans. Here’s a breakdown of the different tiers and their associated features:
Free Plan
- Users can write up to 2,000 words per month.
- Access to all available tools, including Codex for code generation, Chat for conversational prompts, and Content Rewriter for unique content creation.
- Support for writing in over 20 languages.
Creator Plan
- This plan allows users to write up to 120,000 words per month.
- It includes all the features available in the free plan, with increased word limits to accommodate more extensive content creation needs.
Business Plan
- Users can write up to 1,000,000 words per month.
- This plan includes all the features from the Creator plan, with even higher word limits to support larger content generation requirements.
Enterprise Plan
- Offers unlimited content generation.
- Includes custom OpenAI API access.
- Allows the addition of managers and employees.
- Provides fully white-label options for customization and seamless integration with existing workflows.
- This plan is ideal for large-scale businesses and organizations that require extensive content creation capabilities.
Additional Features
- Codex: Streamlines the code generation process, beneficial for both beginner and experienced developers.
- Chat: Uses AI and natural language processing to understand complex queries and enhance creativity through conversational prompts.
- Content Rewriter: Generates unique versions of existing content, useful for repurposing content, avoiding plagiarism, and saving time.
ADLN’s pricing structure is designed to be flexible and scalable, allowing users to choose the plan that best fits their specific needs and budget.
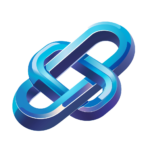
ADLN - Integration and Compatibility
Integration and Compatibility of ADLumin
Integration with Third-Party Tools and Platforms
ADLumin, a cybersecurity platform, is known for its extensive integration capabilities with various third-party devices and applications. Here are some highlights:Cloud-Based SaaS Solutions
ADLumin integrates with a wide range of cloud-based SaaS solutions, including network and endpoint security solutions, as well as office and collaboration suites like Google Workspace.AWS and Google Workspace
Specifically, ADLumin has launched API integrations with AWS and Google Workspace, allowing for the automatic collection, tracking, and alerting on IAM user events and audit logs from these services.Event Log Aggregation
The platform aggregates event logs from multiple sources, parses the data into a native format, and correlates events across existing data. This enables real-time tracking of incidents and alerts on potential threats.Compatibility Across Different Platforms and Devices
Cloud-Native Solutions
ADLumin is built as a cloud-native solution, which makes it compatible with the increasing role of SaaS in enterprise environments. This ensures seamless integration with remote work setups and various cloud services.Multi-Platform Support
The platform combines network, endpoint, and cloud security data, making it versatile and compatible with a wide range of devices and platforms. It supports data collection from sources such as network traffic, web servers, VPNs, firewalls, and custom applications.Real-Time Analysis and Alerts
Custom Detections
Users can create custom detections for integrated services like AWS and Google Workspace, allowing for alerts based on user-specified criteria in the event logs.24/7 Security Operation Center (SOC)
ADLumin provides continuous monitoring, investigation, and response through its SOC, ensuring real-time visibility and action against security threats. In summary, ADLumin’s integration capabilities are extensive and designed to work seamlessly with a variety of third-party tools and platforms, particularly in cloud-based environments. This ensures comprehensive security monitoring and real-time threat detection across multiple devices and services.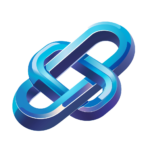
ADLN - Customer Support and Resources
Customer Support
ADLN does not provide detailed information on direct customer support contact options such as phone numbers or specific support email addresses in the sources available. However, it is clear that users can interact with the platform through its integrated tools.
Additional Resources
AI-Powered Tools
ADLN offers a suite of AI-powered tools that include features like Codex for code generation, Chat for enhancing communication, and a Content Rewriter to avoid plagiarism and generate unique content. These tools are aimed at streamlining work processes and increasing productivity.
Content Generation
Users can generate high-quality content quickly, including blog posts, social media ads, emails, and more. The platform uses natural language processing to enhance creativity and engagement.
Automation and Integration
ADLN provides automation tools for data analysis, decision-making, and workflow streamlining. It also integrates with other tools like WordPress and Google Drive, allowing users to export content into various formats such as PDF, Word, and TXT.
Language Support
The platform supports over 20 different languages, making it accessible to a wide range of users.
Pricing Plans
ADLN offers tiered pricing plans, including a free option, a Creator plan ($12.99/month), a Business plan ($99.99/month), and an Enterprise plan with custom options and a fully white-labeled experience.
While the specific contact details for customer support are not provided, users can leverage the comprehensive suite of AI-powered tools and features to address many of their needs directly through the platform. For any additional inquiries or support, users might need to refer to the general contact or support sections if available on the ADLN website, though this information is not explicitly mentioned in the sources.
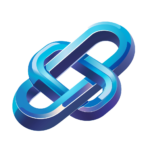
ADLN - Pros and Cons
Advantages
Efficiency and Speed
AI-driven tools can process enormous datasets at high speeds, providing insights much faster than traditional methods. This accelerates decision-making and helps organizations stay ahead of the competition.Improved Accuracy
AI minimizes human intervention, reducing the chances of errors in data analysis. This leads to more accurate data and better strategies.Predictive Analytics
AI can predict future trends and outcomes based on historical data, which is invaluable for making informed decisions about product development and market strategies.Automation of Repetitive Tasks
AI automates tasks such as data cleaning, sorting, and prototyping, allowing professionals to focus on more creative and strategic aspects of product development.Enhanced Creativity
Generative design powered by AI can suggest hundreds or thousands of design iterations, sparking new ideas that might not occur to human designers. This enhances creativity and innovation in product design.Personalization
AI enables the creation of products that are highly personalized to individual customer preferences, improving customer satisfaction and loyalty.Cost Reduction
AI-driven prototyping and simulation reduce the need for physical prototypes, significantly cutting costs and development time.Disadvantages
Hardware Dependency
AI models, especially deep learning models, require heavy machinery and hardware equipment to operate effectively. This can be a significant investment, particularly for beginners or those on a tight budget.Data Dependency
The efficiency of AI models is highly dependent on the quality and quantity of data available. If the data is insufficient or not suitable, the results can be faulty or misleading.Incomplete Results
AI models, particularly neural networks, can sometimes produce incomplete or inaccurate results if they are not fully trained or if the training data is inadequate.Overfitting
Deep learning models can suffer from overfitting, where they perform well on training data but generalize poorly on new, unseen data. This requires careful hyper-parameter tuning, which can be tedious and time-consuming.Lack of Transparency
AI models, especially deep learning models, can be difficult to interpret due to their complex nature. This makes it challenging to understand what is happening in the hidden layers of the network.Training Requirements
AI models often require large amounts of data for training, which can be costly and time-consuming to obtain. The training process itself can also be computationally intensive. Given the absence of specific information about ADLN, these points reflect the general advantages and disadvantages associated with AI-driven product development and analytics tools.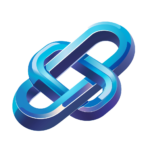
ADLN - Comparison with Competitors
To Compare ADLN.io with Other AI-Driven Analytics Tools
We need to look at the key features, unique selling points, and potential alternatives in the market.Unique Features of ADLN.io
Since the provided link does not offer detailed information about ADLN.io’s specific features, it’s challenging to highlight its unique aspects directly. However, in general, AI-driven analytics tools typically focus on advanced data integration, visualization, machine learning, and predictive analytics.Competitors and Alternatives
Adobe Analytics
Adobe Analytics offers a comprehensive suite of tools that include Customer Journey Analytics, Web & Mobile Analytics, Product Analytics, and Content Analytics. It stands out with its ability to connect customer identity and interactions across channels, devices, and time, providing a cohesive view of the customer journey. Adobe Analytics also uses generative AI to accelerate everyday tasks and deliver insights quickly.Google Analytics
Google Analytics is another powerful tool that uses machine learning to identify patterns and trends in data. It can predict future user actions and surface actionable insights, helping marketers improve their marketing campaigns. Google Analytics also automates the identification of significant trends and anomalies in data.Tableau
Tableau is renowned for its advanced visualization capabilities and interactive dashboards. It offers AI-powered recommendations, predictive modeling, and natural language processing features like Ask Data and Explain Data. Tableau enables users to explore data easily and identify trends, patterns, and outliers.Microsoft Power BI
Microsoft Power BI is a cloud-based business intelligence platform that integrates with Microsoft Azure for advanced analytics and machine learning. It provides interactive visualizations, data modeling, and pre-built connectors for various data sources. Power BI is known for its ability to visualize and analyze data from multiple sources and create interactive dashboards and reports.Pecan AI
Pecan AI is an automated machine learning and predictive analytics platform. It automates the data science cycle, including data ingestion, cleaning, feature selection, model building, and validation. This tool is particularly useful for non-technical users who can create predictive models with ease.Key Differences and Considerations
Data Integration and Preparation
Tools like Adobe Analytics and Pecan AI offer advanced data integration and preparation features, which are crucial for handling data from various sources and ensuring it is ready for analysis.Visualization Capabilities
Tableau and Microsoft Power BI are strong in data visualization, providing interactive dashboards and a range of visualization options that make complex data more interpretable.Machine Learning and Predictive Analytics
Google Analytics, Pecan AI, and Salesforce Einstein Analytics use machine learning to predict future trends and outcomes, giving businesses a strategic edge in decision-making.User-Friendly Interfaces
Tableau, Power BI, and Pecan AI are known for their user-friendly interfaces, making advanced analytics accessible to a broader range of users, including those without extensive technical expertise. If specific details about ADLN.io’s features are not available, it would be beneficial to compare it directly against these competitors based on the general categories of data integration, visualization, machine learning, and user interface. However, without more detailed information on ADLN.io, it’s challenging to pinpoint its unique features or how it stands out in the market.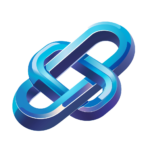
ADLN - Frequently Asked Questions
Frequently Asked Questions About AI-Driven Analytics Tools
1. What is AI Analytics?
AI analytics refers to the application of artificial intelligence technologies to enhance and automate data analysis. It uses machine learning algorithms, natural language processing (NLP), and other features to process large volumes of data, identify patterns, and extract insights that companies can use to improve business performance.2. How Does AI Analytics Work?
AI analytics works by automating the data analysis process using machine learning algorithms. These algorithms can build predictive models, detect anomalies, and identify trends. The process involves data collection, processing, analysis, and reporting, often using tools like generative AI to generate human-readable insights and visualizations.3. What Are the Key Capabilities of AI Analytics Tools?
Key capabilities include machine learning and automated analysis, natural language search, predictive analytics, automated data storytelling, metrics monitoring, and automated data visualization and reporting. These tools can handle large-scale data from multiple sources, integrate and prepare data, and provide intuitive interfaces for non-technical users.4. How Do AI Analytics Tools Handle Data Integration and Preparation?
AI analytics tools can import data from various sources, including spreadsheets, databases, and cloud applications. They can cleanse, transform, and integrate these datasets into a unified format ready for analysis. Automated features like data cleaning and preparation save time and ensure accuracy.5. What Role Does Generative AI Play in AI Analytics?
Generative AI, such as GPT and LLMs, enhances AI analytics by generating human-like text and language-based outputs. It helps in advanced embedding techniques, pre-processing tasks like correcting misspellings and standardizing date formats, and post-processing tasks like generating summaries and visualizations. This makes insights more accessible and interpretable for non-technical users.6. Can AI Analytics Tools Be Used by Non-Technical Users?
Yes, AI analytics tools are designed to be user-friendly and accessible to a broader range of business users. They offer intuitive interfaces, natural language search capabilities, and automated data visualization, making it easier for non-technical users to create predictive models, analyze data, and generate insights.7. How Do AI Analytics Tools Support Predictive Analytics?
AI analytics tools employ advanced machine learning algorithms to build predictive models. These models can forecast future outcomes, identify trends, and detect anomalies, enabling organizations to make data-driven decisions. Predictive analytics enhances the ability to anticipate and prepare for future events.8. What Are Some Examples of Advanced Analytics Tools?
Examples include Pecan AI, Tableau, and Power BI. These tools offer a combination of advanced analytics capabilities, such as automated machine learning, advanced visualization, and user-friendly interfaces, making them suitable for various business needs.9. How Do AI Analytics Tools Facilitate Collaboration and Reporting?
AI analytics tools provide features to create interactive data visualizations and reports that can be exported in various formats (e.g., CSV, PDF, HTML). They also enable sharing insights across multiple platforms, facilitating seamless collaboration and increased productivity.10. What Should I Consider When Choosing an AI Analytics Tool?
When choosing an AI analytics tool, consider the type of data you need to analyze, your budget, and the level of data analysis experience your team has. Look for tools that can import data from multiple sources, offer advanced visualization capabilities, and provide user-friendly interfaces. Also, consider scalability and the ability to convert unstructured data into meaningful insights.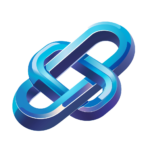
ADLN - Conclusion and Recommendation
General Assessment of AI-Driven Analytics Tools
AI-driven analytics tools, like those described in the context of augmented analytics, offer significant benefits for organizations. Here are some key points:Key Benefits
- Simplification and Automation: These tools simplify the process of cleaning, organizing, and understanding data, making data analysis faster and more accessible to users of all skill levels.
- Insight Generation: They enable businesses to find patterns, predict trends, and make smarter decisions that drive growth. This is achieved through advanced technologies like AI, ML, and NLP.
- Real-Time Analysis: Real-time analysis allows businesses to adapt quickly to market changes or operational challenges, which is crucial in industries where timing is critical.
- Collaboration and Accessibility: These tools encourage teamwork by enabling multiple departments to share, analyze, and discuss insights in a collaborative environment.
Who Would Benefit Most
- Business Analysts and Decision Makers: Individuals responsible for data-driven decision-making would greatly benefit from these tools as they provide actionable insights and automate complex analytics tasks.
- Marketing and Product Teams: Teams involved in marketing, product development, and customer journey optimization can leverage these tools to gain deeper insights into customer behavior and preferences.
- Cross-Functional Teams: Any department that relies on data to make informed decisions can benefit, as these tools facilitate collaboration and provide user-friendly interfaces for diverse users.
Overall Recommendation
Given the general benefits of AI-driven analytics tools, if ADLN aligns with these principles, it would likely be a valuable asset for any organization seeking to enhance their data analysis capabilities. Here are some recommendations:- Evaluate Data Quality: Ensure that the tool can handle and improve data quality, as poor data quality can lead to unreliable insights.
- Assess Integration Capabilities: Check if the tool can integrate with existing systems and data sources to provide a unified view of customer and business data.
- Consider User Adoption: Ensure the tool is user-friendly and accessible to a wide range of users, regardless of their technical expertise.
- Monitor and Optimize: Continuously monitor the performance of the tool and optimize its use based on feedback and insights generated.