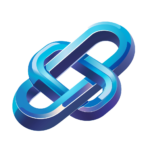
Google Recommendations AI - Detailed Review
Analytics Tools
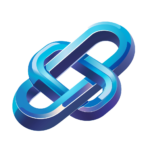
Google Recommendations AI - Product Overview
Introduction to Google Recommendations AI
Google Recommendations AI is a personalized product recommendation system developed by Google, integrated into the Google Cloud Platform (GCP). This AI-driven tool is part of the Retail console in GCP and is designed to help businesses deliver highly accurate and personalized product recommendations to their customers.
Primary Function
The primary function of Google Recommendations AI is to analyze user behavior and product data to generate relevant and personalized recommendations. It uses machine learning models to suggest products based on user interactions such as purchases, adds to cart, and page views. This helps in increasing customer engagement, basket size, and average order value.
Target Audience
The target audience for Google Recommendations AI includes e-commerce businesses, retailers, and wholesalers who aim to enhance their customer experience and increase sales. It is particularly beneficial for organizations that want to implement advanced recommendation systems without requiring extensive machine learning expertise.
Key Features
Data Ingestion and Integration
Recommendations AI allows you to ingest data from your product catalog and customer interactions. You can import historical data from sources like Google Analytics, BigQuery, or Google Cloud Storage, or collect real-time data using Google Tag Manager or JavaScript pixels.
Customizable Recommendations
The system offers various recommendation types, such as “Recommended for you,” “Other items you may like,” and “Frequently bought together.” You can select objectives like click-through rate, conversion rate, or revenue per session, and apply business rules to filter recommendations (e.g., excluding out-of-stock items or duplicate items).
Real-Time Adaptation
Recommendations AI adapts to user behavior in real-time, ensuring that the recommendations are always relevant and up-to-date. This is achieved through the use of state-of-the-art deep learning models, such as Transformers.
Fully Managed Service
The system is fully managed, meaning you do not need machine learning expertise to implement and maintain it. It retrains models daily to ensure they remain optimized for the selected objectives.
Cross-Channel Personalization
Recommendations can be delivered across multiple channels, including websites, mobile apps, email, and contact centers, ensuring a consistent and personalized customer experience.
Handling Cold-Start Challenges
Recommendations AI can handle scenarios where there is limited customer or product data, leveraging Google Cloud’s extensive experience in recommendations and search.
By leveraging these features, Google Recommendations AI helps businesses deliver highly personalized and effective product recommendations, leading to increased customer satisfaction and revenue.
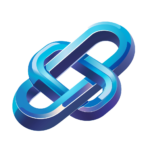
Google Recommendations AI - User Interface and Experience
User Interface Overview
The user interface of Google Cloud Recommendations AI is designed to be user-friendly and accessible, even for those without extensive machine learning (ML) expertise.Ease of Use
Google Cloud Recommendations AI offers an easy-to-use graphical interface that simplifies the process of creating and managing recommendation models. Users can create models, define optimization objectives, and customize business rules with just a few clicks. This interface is intuitive, allowing users to set up and train models without needing deep ML knowledge.Key Steps and Interface Components
To get started, users need to:- Create a project in Google Cloud Platform.
- Import their product catalog and record key user events such as purchases, adds to cart, and page views.
- Determine the recommendation types and placements on their website or app.
- Create and train the model, which typically takes 2-5 days.
- Choose the model type and assign a business objective.
- Create placements for each area on the website or app where recommendations will be displayed.
- Preview recommendations to ensure they meet the desired criteria before deploying them to production.
User Experience
The overall user experience is streamlined and efficient. Users can easily integrate Recommendations AI with existing Google tools like Google Analytics and Google Tag Manager, making the setup process smoother. The system provides clear documentation and examples, which users have praised for their clarity and helpfulness.Personalization and Real-Time Recommendations
The interface enables real-time personalization, allowing the AI to predict the next best item to recommend based on current user activities. This is achieved through advanced ML models, such as Transformers, which capture the interconnection between a user’s history, current landing page, and next action.Feedback and Optimization
Users can set up A/B tests to objectively measure the impact of Recommendations AI, using optimization platforms like Google Optimize or Optimizely. This feature helps in evaluating the model’s performance and making necessary adjustments.Conclusion
In summary, Google Cloud Recommendations AI provides a straightforward, user-friendly interface that makes it easy to implement and manage personalized recommendation systems, even for users without extensive technical expertise.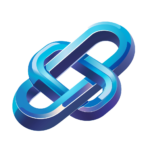
Google Recommendations AI - Key Features and Functionality
Google Cloud Recommendations AI
Google Cloud Recommendations AI is a sophisticated tool designed to deliver highly personalized recommendations, enhancing user engagement and driving business revenue. Here are the main features and how they work:
Personalization
Google Cloud Recommendations AI uses advanced machine learning models to provide personalized suggestions based on user interactions, such as purchase history, search queries, and other behavioral data. This personalization ensures that the recommendations are relevant and timely, leading to a better user experience and higher engagement rates.
Scalability
The service is built on Google Cloud’s infrastructure, allowing it to handle large volumes of data and traffic. This scalability makes it suitable for businesses of all sizes, ensuring that the recommendation system can grow with the business without compromising performance.
Integration
Recommendations AI integrates seamlessly with existing systems and workflows. It can import data from various sources such as Google Analytics, BigQuery, Google Cloud Storage, and more, using tools like Google Tag Manager or JavaScript snippets. This easy integration enables businesses to implement the service without significant disruptions.
Real-time Insights
The service provides real-time analytics and insights that help businesses understand user behavior and optimize their recommendation strategies. This includes monitoring the performance of recommendations and making necessary adjustments to improve accuracy and engagement.
Data Collection and Model Training
Recommendations AI collects data from various sources, including user interactions, product catalogs, and sales history. It then uses AutoML (Automated Machine Learning) to train custom models that can learn from the data and improve over time. This automated process eliminates the need for manual model training and hyperparameter tuning.
Deployment and Monitoring
Once the models are trained, they are deployed into the production environment to start generating recommendations. The system continuously monitors the performance of these recommendations and makes adjustments as necessary to maintain or improve accuracy.
Customization and Business Rules
Businesses can customize the recommendations to fit their specific needs. This includes selecting recommendation types (e.g., “Recommended for you,” “Other items you may like,” “Frequently bought together”), setting objectives (e.g., click-through rate, conversion rate, revenue per session), and applying business rules such as filtering out-of-stock items or duplicate items.
AI Integration
Google Cloud Recommendations AI leverages state-of-the-art machine learning models, including deep learning models like Transformers. These models predict user behavior in real-time, taking into account the user’s current activities and preferences. This integration of AI ensures that the recommendations are highly accurate and relevant, enhancing user engagement and retention.
Federated Learning and Active Learning
The service also supports techniques like Federated Learning, which allows for collaborative model training while maintaining user privacy, and Active Learning, which enables models to query users for feedback on uncertain predictions. These techniques help refine the model’s accuracy over time and adapt to changing user preferences.
By combining these features, Google Cloud Recommendations AI provides a comprehensive solution for delivering personalized recommendations, driving engagement, and increasing revenue, all while ensuring ease of implementation and maintenance.
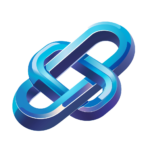
Google Recommendations AI - Performance and Accuracy
Google Cloud Recommendations AI
Google Cloud Recommendations AI is a sophisticated tool within the Google Cloud Platform (GCP) that leverages advanced machine learning techniques to provide personalized product recommendations. Here’s an evaluation of its performance and accuracy, along with some limitations and areas for improvement.
Performance
- Scalability: Recommendations AI is built on Google Cloud’s infrastructure, which allows it to handle large volumes of data and traffic, making it suitable for businesses of all sizes.
- Real-time Insights: The service provides real-time analytics and insights, enabling businesses to understand user behavior and optimize their recommendation strategies promptly.
- Automated Processes: It automates the process of data ingestion, model training, and deployment, reducing the need for manual intervention and ML expertise.
- Integration: Recommendations AI integrates seamlessly with existing systems and workflows, facilitating easy implementation.
Accuracy
- Personalization: The service uses historical data and user interactions to train custom models, providing highly accurate and personalized recommendations. This personalization is based on user behavior and preferences, ensuring that the suggestions are relevant and timely.
- Model Training and Optimization: The models are trained using AutoML and are re-trained daily to incorporate insights from changing catalogs, user behavior, and shopping trends. This continuous optimization helps in maintaining high accuracy.
- Handling Bias and Sparse Data: Recommendations AI corrects for bias with extremely popular or on-sale items and better handles long-tail items with sparse data, as well as seasonal items, to drive better click-through rates (CTR), conversion rates (CVR), and revenue lift.
Limitations and Areas for Improvement
- Data Quality: The accuracy of the recommendations heavily depends on the quality of the product catalog and user event data. High-quality, clean, complete, and up-to-date data are crucial for optimal performance.
- Daily Updates: While the models are re-trained daily, ensuring the product catalog is updated daily is essential for maintaining relevance and accuracy.
- A/B Testing: To fully evaluate the performance of Recommendations AI, setting up A/B tests is recommended. This helps in objectively measuring the impact of the recommendations and making data-driven decisions.
- Limited Context: The models may not capture long-term trends or seasonal fluctuations as effectively as they handle short-term data. This can be a limitation in scenarios where long-term trends are significant.
Additional Considerations
- Business Rules: Recommendations AI allows businesses to apply custom business rules to fine-tune what customers see, such as diversifying product displays and filtering by product availability. This flexibility helps in aligning the recommendations with specific business goals.
- Data Ownership: The data and models used in Recommendations AI are owned by the user and are not used for any other Google product or shared with other customers, ensuring data privacy and control.
In summary, Google Cloud Recommendations AI offers high performance and accuracy through its advanced machine learning models and real-time insights. However, it requires high-quality data and regular updates to maintain its effectiveness. Additionally, while it handles short-term data well, it may have limitations in capturing long-term trends.
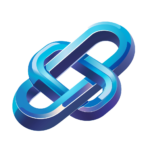
Google Recommendations AI - Pricing and Plans
Pricing Structure for Google’s Recommendations AI
The pricing structure for Google’s Recommendations AI, which is part of the Analytics Tools and AI-driven product category, is outlined as follows:
Volume-Based Pricing Tiers
Google Recommendations AI operates on a volume-based pricing model, which includes several tiers for predictions, as well as separate charges for model training and tuning.
Predictions
The service offers three volume-based pricing tiers for generating recommendations. The exact pricing details for these tiers are not specified in the available sources, but they are based on the volume of predictions made.
Model Training and Tuning
There are additional charges for training and tuning the recommendation models. The training process typically takes between two to five days, depending on the model’s complexity.
Features Available in Each Plan
Basic Recommendations
Most customers can access basic recommendations and insights for free through various exposure channels, such as the UI and API. This includes access to recommendations via the UI and API, with different API quotas depending on the customer’s support package (e.g., Basic, Standard, Enhanced, Premium).
Premium Recommendations
For more advanced insights, such as Firewall Insights, customers need to purchase a specific SKU. This premium tier provides full UI and API access, as well as the ability to export insights to BigQuery.
Free Options
Free Recommendations
Google Cloud generates most recommendations and insights for free to all customers. This includes access to the UI and API, although the API quotas vary based on the customer’s support level.
Free Trial and Limited Free Usage
While there is no specific free trial mentioned for Recommendations AI, the service does offer free access to basic recommendations. For other AI tools within Google Cloud, new customers often receive free credits and limited free usage up to certain monthly limits.
Additional Considerations
Support Packages
The availability and quotas of recommendations via the UI and API depend on the customer’s support package. Customers with Standard, Enhanced, or Premium support packages have higher API quotas and the ability to export recommendations to BigQuery.
In summary, Google Recommendations AI offers a mix of free and paid options, with volume-based pricing for predictions and additional charges for model training and tuning. The free tier provides basic recommendations, while premium features require a purchase.
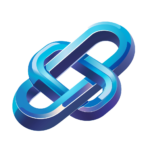
Google Recommendations AI - Integration and Compatibility
Google Recommendations AI Overview
Google Recommendations AI is a sophisticated tool that integrates seamlessly with various Google and external tools, enhancing its compatibility and utility across different platforms and devices.
Integration with Google Tools
- Google Cloud Platform (GCP): Recommendations AI is part of the Retail console in GCP, making it easy to set up and manage within the Google Cloud ecosystem. You need to create a project in GCP, link it to a billing account, and set up authentication to get started.
- Google Analytics: To leverage Recommendations AI effectively, you need to capture Enhanced Ecommerce metrics with Google Analytics. This data is crucial for training the recommendation models and ensuring they provide relevant suggestions.
- Google Merchant Center: You can import your product catalog data from Google Merchant Center, Google BigQuery, or Google Cloud Storage, making the integration with existing Google services straightforward.
Integration with Websites and Apps
- API Integration: Recommendations AI uses API calls to retrieve recommendations based on user queries. You can integrate the Recommendations AI API into your website or app by making POST requests that include the necessary user event data and other parameters. This allows you to populate content slots with personalized recommendations.
- Placement and Display: You can determine various recommendation types and placements on your website or app. For example, you can create placements for product detail pages, home pages, or any other area where you want to display recommendations. Each placement is associated with a specific model, and you can preview the recommendations before deployment.
Compatibility Across Platforms
- Web and Mobile: Recommendations AI can be integrated into both web and mobile applications. The API-based approach ensures that recommendations can be generated and displayed across different devices, enhancing user engagement and personalization.
- Adobe Commerce: For users of Adobe Commerce (formerly Magento), there is an extension available that allows easy integration of Google Recommendations AI. This extension supports Adobe Commerce 2.4.x and requires PHP 7.3 or 8.0 , making it compatible with a range of e-commerce platforms.
Additional Requirements and Considerations
- Data Quality: High-quality product catalog data is essential for the effective functioning of Recommendations AI. Ensuring that your product data is accurate, clean, complete, and up-to-date is crucial for training the models and generating relevant recommendations.
- Team Roles: Implementing Recommendations AI requires a team with various skills, including strategy, configuration setup in GCP, automated data processes, and code changes to display recommendations on the website or app.
By integrating Google Recommendations AI with these tools and ensuring compatibility across different platforms, businesses can significantly enhance user engagement, conversions, and overall revenue.
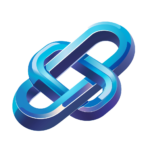
Google Recommendations AI - Customer Support and Resources
Support and Resources
Accelerate with Google
Google offers a marketing resource hub called Accelerate with Google, which is part of their “Summer of AI Essentials” campaign. This hub provides a central destination for marketers to learn about AI-powered ad products, including Google Recommendations AI. It includes customized skill assessments, a library of video tutorials and articles, and upcoming digital live streams on AI marketing topics. This resource helps marketers stay updated with new AI functionalities and best practices.Google Cloud Documentation and Guides
Google Cloud provides comprehensive documentation and guides for setting up and using Recommendations AI. These resources include step-by-step instructions on importing product catalogs, setting up access tokens, adding tracking events, training the AI model, and placing recommendations on your webshop. For example, a live demo video walks users through the entire process using tools like React, Next.js, and Crystallize.Integration with Existing Tools
Recommendations AI integrates seamlessly with other Google tools such as Google Analytics and Google Tag Manager. This integration allows users to import existing user events easily, making the setup process more straightforward. Users can also choose from various methods like BigQuery, Google Cloud Storage, API, or JavaScript snippets to import their data.Customization and Configuration
Users can customize Recommendations AI to fit their specific needs by choosing from three recommendation types: “Recommended for you,” “More like this,” and “Others.” Additionally, users can apply various filtering rules and configurations to optimize for click-through rate, click and play rate, or watch time, and filter recommendations by item availability, price, or other criteria.AI Readiness Program
Google Cloud offers an AI Readiness Program, a 2-3 week engagement that helps businesses accelerate value realization from their AI efforts. Experts work with clients to benchmark their AI capabilities and provide recommendations for their specific needs.Community and Developer Resources
Google provides access to GitHub repositories and community forums where users can share code, ask questions, and get support from other developers who are using Recommendations AI. For instance, the live demo video shares a GitHub repository of the code used in the demonstration. By leveraging these resources, users can ensure they are getting the most out of Google Recommendations AI and optimizing their recommendation systems for better customer engagement and retention.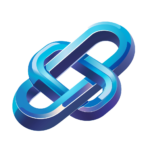
Google Recommendations AI - Pros and Cons
Advantages of Google Cloud Recommendations AI
Personalization and Engagement
Google Cloud Recommendations AI excels in providing highly personalized product recommendations, which can significantly enhance user engagement and conversion rates. It uses advanced machine learning models to analyze user behavior, such as shopping and viewing history, to suggest relevant products or content.
Ease of Use
The platform offers an easy-to-use graphical interface, allowing users to choose their model type and define optimization objectives without requiring deep machine learning expertise. This simplicity makes it accessible even for those who are not tech experts.
Scalability and Automation
Recommendations AI can handle large amounts of data and scale automatically to manage unpredictable traffic spikes, eliminating the need for manual data preprocessing, model training, or infrastructure provisioning.
Customization
Users can customize recommendations to achieve specific business goals, such as increasing engagement, revenue, or conversions. Business rules can be applied to fine-tune what customers see, including filters for product availability and custom tags.
Real-Time Recommendations
The AI provides real-time recommendations, which can be integrated across various touchpoints, including home pages, product detail pages, cart pages, email campaigns, and mobile apps. This ensures that recommendations are relevant and timely.
Success Stories
Several companies, such as IKEA Retail, Hanes Australasia, and 1-800-FLOWERS.COM, Inc., have seen significant improvements in sales, average order value, and customer lifetime value by using Recommendations AI.
Disadvantages of Google Cloud Recommendations AI
Complex Setup
While the interface is user-friendly, the initial setup process can be complex and time-consuming. It requires careful tagging of content and structuring of the product catalog to ensure the AI’s effectiveness.
Limited Recommendations
Some users have noted that the recommendations can sometimes be very limited, and the AI may not always align with user preferences, especially if it relies too heavily on machine learning models rather than user behavior.
Pricing
The pricing of Google Cloud Recommendations AI is considered to be on the higher side by some users, which may not be justified unless there is a significant improvement in revenues.
User Interface and Optimization
There is room for improvement in the user interface and automated optimization features. Some users suggest that these aspects could be made better to enhance the overall user experience.
Data Limitations
The AI’s effectiveness depends on the quality and completeness of the data provided. If the data is incomplete or not well-structured, the recommendations may not be as accurate or relevant.
By considering these points, you can make an informed decision about whether Google Cloud Recommendations AI aligns with your business needs and goals.
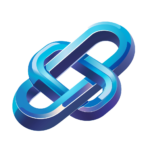
Google Recommendations AI - Comparison with Competitors
Google Cloud Recommendations AI
- This service is part of Vertex AI Search and is known for its advanced machine learning algorithms that analyze historical data to predict future user behavior. It offers personalization based on user interactions, ensuring relevant and timely recommendations.
- It is highly scalable, built on Google Cloud’s infrastructure, and can handle large volumes of data and traffic. This makes it suitable for businesses of all sizes.
- Google Cloud Recommendations AI integrates easily with existing systems and workflows, and it provides real-time insights to help businesses optimize their recommendation strategies.
- The service allows for customization of recommendations to deliver specific outcomes such as engagement, revenue, or conversions. Businesses can apply various filtering rules and configurations, such as optimizing for click-through rate, watch time, or item availability.
Unique Features
- Federated Learning: Google Cloud Recommendations AI uses Federated Learning, which allows for collaborative model training while maintaining user privacy by training models on local data.
- Integration with Google Tools: It seamlessly integrates with other Google tools like Google Analytics and Google Tag Manager, making it easy to implement without requiring AI expertise.
- Advanced Machine Learning Models: The service utilizes state-of-the-art machine learning models, including deep learning models like Transformers, to predict user interests in real-time.
Alternatives and Comparisons
BytePlus Recommend
- BytePlus Recommend is a fully managed service that provides dynamic and targeted recommendations. It leverages machine learning expertise and automates the machine-learning workflow, making it easy to use with existing infrastructure. However, it does not offer the same level of integration with other tools as Google Cloud Recommendations AI.
Qubit
- Qubit CommerceAI generates product recommendations and insights using deep learning technology. It creates a model for each shopper, making interactions more relevant. While Qubit offers powerful personalization, it may not match the scalability and integration capabilities of Google Cloud Recommendations AI.
Froomle
- Froomle provides AI-powered recommendations for digital publishing and eCommerce, focusing on specialized modules tailored to specific business needs. It does not have the broad scalability or the extensive integration options that Google Cloud Recommendations AI offers.
Constructor.io
- Constructor.io automates product discovery using machine learning to optimize search, autosuggest, and recommendation results. While it provides advanced tools for personalization, it is more focused on search optimization rather than the comprehensive recommendation capabilities of Google Cloud Recommendations AI.
AutoCommerce
- AutoCommerce is a free app for Shopify that provides Amazon-like product suggestions. It is simpler and more limited in scope compared to Google Cloud Recommendations AI, lacking the advanced machine learning and integration features.
Key Differences
- Scalability and Integration: Google Cloud Recommendations AI stands out for its ability to handle large volumes of data and its seamless integration with other Google tools, which is not always matched by its competitors.
- Advanced Machine Learning: The use of Federated Learning and deep learning models like Transformers sets Google Cloud Recommendations AI apart in terms of accuracy and real-time personalization.
- Customization and Flexibility: Google Cloud Recommendations AI offers extensive customization options, including various filtering rules and configurations, which may not be as comprehensive in some of the alternative solutions.
In summary, while alternatives like BytePlus Recommend, Qubit, Froomle, and others offer strong personalization capabilities, Google Cloud Recommendations AI’s unique features, such as its scalability, integration with Google tools, and advanced machine learning models, make it a compelling choice for businesses seeking robust and personalized recommendation solutions.
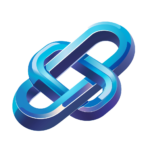
Google Recommendations AI - Frequently Asked Questions
Frequently Asked Questions about Google’s Recommendations AI
What is Google’s Recommendations AI and how does it work?
Google’s Recommendations AI is a personalized product recommendation system that uses advanced machine learning models to analyze user interactions and product catalog data. It trains models using your product catalog and analytics data to provide personalized recommendations. The process involves data collection, model training, and deployment, with continuous monitoring and optimization to improve accuracy.What are the key steps to get started with Recommendations AI?
To get started, you need to:- Create a project in Google Cloud Platform.
- Import your product catalog.
- Determine your recommendation types and placements.
- Record key user events such as purchases, adds to cart, and views.
- Create and train your model.
- Create placements and preview recommendations.
- Deploy the model, ideally as part of an A/B test.
- Evaluate the model’s performance.
Does Recommendations AI stop recommending a product after a user purchases it?
Recommendations AI typically adjusts its recommendations based on user behavior. While it may not immediately stop recommending a purchased product, it often lowers the priority of that item in subsequent recommendations. This behavior can be customized and fine-tuned based on your configuration.Can I create configurations and controls based on user event attributes?
Yes, Recommendations AI allows you to set up rules and controls that influence recommendations when certain events occur, such as purchases or adding items to the cart. These event-based rules can adjust user preferences or alter the recommendation logic for subsequent interactions.Does Recommendations AI use cookies in the end-to-end process?
Recommendations AI may use cookies as part of the data collection and tracking process. However, it is possible to perform some actions without using cookies, depending on the specific implementation and data sources used. For example, you can import data from Google Analytics or Google Tag Manager without relying on cookies.What personalization techniques does Recommendations AI use?
Recommendations AI employs several personalization techniques, including Federated Learning, Active Learning, and Knowledge Distillation. These methods help create accurate and relevant recommendations by considering user behavior and preferences while maintaining user privacy.How does Recommendations AI integrate with existing systems?
Recommendations AI integrates easily with existing systems and workflows, including Google Analytics and Google Tag Manager. This integration allows for seamless implementation and the use of existing user event data to train and optimize the recommendation models.What types of recommendation models can I create with Recommendations AI?
You can create several types of recommendation models, such as “Recommended for You” (suitable for category or home pages), “More Like This” (suitable for item detail pages), and others. Each model can be customized to fit specific needs and objectives, such as optimizing for click-through rate, click and play rate, or watch time.Do I need machine learning expertise to implement Recommendations AI?
No, you do not need machine learning expertise to implement Recommendations AI. The service is designed for straightforward implementation, allowing you to build end-to-end recommendation solutions without requiring ML expertise.How long does it take to train a model in Recommendations AI?
The model training process in Recommendations AI typically takes 2-5 days, assuming you have met the minimum data requirements by either importing historical data or recording events in real-time.Can I run A/B tests with Recommendations AI?
Yes, you can set up A/B tests to objectively measure the impact of Recommendations AI. This can be done using optimization platforms such as Google Optimize or Optimizely, and you can send the experiment ID to Recommendations AI to calculate the results.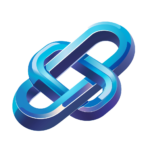
Google Recommendations AI - Conclusion and Recommendation
Final Assessment of Google Recommendations AI
Google Recommendations AI is a powerful tool in the Analytics Tools AI-driven product category, offering several key benefits that make it an attractive solution for businesses seeking to enhance user engagement and drive revenue.
Personalization and Accuracy
Google Recommendations AI excels in personalization, using advanced machine learning techniques such as Federated Learning, Active Learning, and Knowledge Distillation to provide highly accurate and relevant recommendations. These methods ensure that the recommendations are based on user behavior and preferences, leading to a better user experience and increased customer satisfaction.
Scalability and Integration
The service is built on Google Cloud’s infrastructure, making it highly scalable and capable of handling large volumes of data and traffic. It integrates seamlessly with existing systems and workflows, including tools like Google Analytics and Google Tag Manager, which simplifies implementation and reduces the need for extensive technical expertise.
Real-Time Insights and Optimization
Google Recommendations AI provides real-time insights and analytics, allowing businesses to monitor the performance of their recommendations and make necessary adjustments to optimize their strategies. This continuous monitoring and optimization ensure that the recommendations remain relevant and effective over time.
Business Outcomes
The use of Google Recommendations AI has led to significant business outcomes for various companies. For instance, IKEA Retail saw a 2% increase in global average order value for ecommerce, while Hanes Australasia experienced a double-digit uplift in revenue per session. These results highlight the potential of the service to increase sales, average order value, and customer lifetime value.
Who Would Benefit Most
Google Recommendations AI is particularly beneficial for retailers and media companies. Retailers can use it to recommend products based on user history and frequently bought together items, optimizing conversion rates and increasing average order value. Media companies can recommend personalized content and advertisements, leading to increased engagement, viewership, and ad revenue.
Overall Recommendation
Given its advanced personalization techniques, scalability, ease of integration, and the ability to provide real-time insights, Google Recommendations AI is highly recommended for businesses looking to enhance user engagement and drive revenue. It is especially suitable for those without extensive AI expertise, as it offers an out-of-the-box solution that can be customized to meet specific business objectives.
In summary, Google Recommendations AI is a versatile and effective tool that can significantly improve customer engagement, retention, and revenue, making it a valuable addition to any business’s analytics and recommendation strategy.