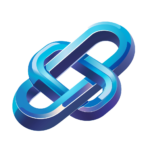
ImageBind by Meta - Detailed Review
Analytics Tools
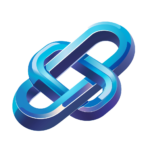
ImageBind by Meta - Product Overview
Introduction to ImageBind by Meta
ImageBind, developed by Meta Research, is a groundbreaking AI model that integrates data from six distinct modalities into a single embedding space. This innovative tool falls within the Analytics Tools AI-driven product category and is particularly significant for its multi-modal capabilities.
Primary Function
The primary function of ImageBind is to forecast relationships between data from various modalities, including text, images/videos, audio, 3D measurements, temperature data, and motion data (from sensors like accelerometers and orientation monitors).
Target Audience
ImageBind is aimed at a broad range of users, including researchers, developers, and individuals with specific needs such as those with vision or hearing impairments. It has the potential to be used in various fields, enhancing multimedia descriptions and providing a more immersive experience.
Key Features
Multi-Modal Integration
ImageBind combines data from six different modalities into a single embedding space, allowing for a holistic interpretation of diverse sensory inputs without explicit supervision.
Cross-Modal Retrieval
Users can provide data in one modality (e.g., audio) and retrieve related documents in different modalities (e.g., video).
Zero-Shot and Few-Shot Classification
ImageBind supports zero-shot classification, where data is embedded and labeled without prior examples, and few-shot classification, which improves performance with just a few examples. It has shown significant gains in accuracy, particularly in few-shot classification tasks.
Open-Source
ImageBind is an open-source project by Meta AI Research, making it accessible for various applications and further development.
Enhanced Applications
It can be used for advanced information retrieval, object detection, and generative AI by combining its embeddings with other models.
Real-Time Multimedia Descriptions
ImageBind has the potential to aid individuals with vision or hearing impairments by providing real-time multimedia descriptions of their environment.
By integrating multiple modalities into a single embedding space, ImageBind offers a comprehensive and innovative approach to handling diverse types of data, making it a valuable tool in the field of AI and multimodal learning.
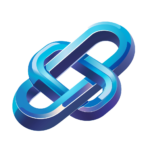
ImageBind by Meta - User Interface and Experience
User Interface
The user interface for ImageBind is likely structured around facilitating the integration and analysis of multiple data modalities, including text, images/videos, audio, 3D measurements, thermal data, and motion data. Here are some key aspects:
Data Input
Users can feed various types of data into the system, such as text, images, audio files, and sensor data. The interface would need to accommodate multiple input formats and possibly include tools for uploading or linking these data sources.
Embedding and Analysis
The interface might include visualizations or dashboards to display the embeddings and the relationships between different modalities. This could involve graphs, charts, or other visual tools to help users see how different types of data are connected.
Search and Retrieval
There would likely be a search function that allows users to find related content across different modalities. For example, a user could input an audio clip and find matching images or videos.
Ease of Use
The ease of use of ImageBind can be assessed based on its open-source nature and the availability of documentation:
Open-Source and Documentation
ImageBind is an open-source project, which means there is likely extensive documentation and community support. This includes a playground published by Meta research with pre-made examples, making it easier for users to get started.
Pre-Made Examples
The presence of pre-made examples and a README file with instructions on how to feed different types of data into ImageBind suggests that the tool is designed to be relatively user-friendly, especially for those familiar with AI and data analysis.
Overall User Experience
The overall user experience is expected to be engaging and informative due to the following reasons:
Multimodal Interactions
By allowing users to interact with and analyze data from multiple modalities in a single embedding space, ImageBind provides a comprehensive and immersive experience. This can help users gain a deeper insight into their data by identifying connections that might not be apparent when analyzing each modality separately.
Practical Applications
The tool enables various practical applications such as cross-modal retrieval, combining modalities with arithmetic, and cross-modal production. This versatility makes it useful for a wide range of tasks, enhancing the user’s ability to generate and enhance content.
Accessibility
The potential for ImageBind to assist users with vision or hearing impairments by providing real-time multimedia descriptions adds a layer of accessibility, making the tool more inclusive and beneficial for a broader audience.
In summary, while specific details about the user interface are not provided, ImageBind by Meta is likely designed to be user-friendly, with a focus on facilitating the integration and analysis of multiple data modalities, and offering a range of practical applications that enhance the user experience.
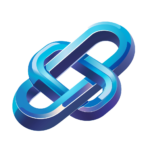
ImageBind by Meta - Key Features and Functionality
Key Features of ImageBind by Meta
Single Embedding Space Across Multiple Modalities
ImageBind is the first AI model to learn a single embedding space that connects six distinct modalities: text, images/videos, audio, 3D measurements, thermal data, and motion data from inertial measurement units (IMU). This unified representation allows the model to analyze and relate different types of data without the need for explicit supervision or paired data across all modalities.
Holistic Learning and Cross-Modal Alignment
ImageBind leverages recent large-scale vision-language models to extend their zero-shot capabilities to new modalities. It uses the natural pairing of images with other modalities (e.g., video-audio, image-depth) to learn a joint embedding space. This approach enables the model to align visual and non-visual modalities, such as audio and IMU readings, without requiring them to appear together in the training data.
Self-Supervised Learning
The model relies on naturally paired self-supervised data for the additional modalities (audio, depth, thermal, and IMU readings). This method allows ImageBind to learn features for different modalities using visual representations from large-scale web data, which act as targets for learning.
Cross-Modal Retrieval and Generation
ImageBind can retrieve data from different sources and generate new information by combining various modalities. For example, it can create images from audio inputs, such as generating visuals that match the sounds of a rainforest or a bustling market. This capability enhances content creation by allowing creators to search for and incorporate relevant content from different modalities.
Improved Performance in Few-Shot Learning
ImageBind outperforms prior specialized models in few-shot learning tasks, such as audio and depth classification. It achieves significant gains in accuracy, up to 40% in top-1 accuracy on ≤four-shot classification tasks, compared to other models trained specifically for those modalities.
Enhanced Creative and Analytical Capabilities
The model enables various creative applications, such as generating richer media content, enhancing videos with appropriate audio clips, and segmenting objects in images based on audio prompts. It also facilitates more accurate content recognition, moderation, and multimodal search functions.
Open-Source and Scalability
ImageBind is an open-source project by Meta AI Research, making it accessible for further development and integration into other AI systems. The model’s strong scaling behavior allows it to benefit from larger vision models, improving its performance in non-vision tasks as well.
Potential for Expanded Modalities
While ImageBind currently supports six modalities, it has the potential to accommodate additional data types by identifying and creating connections between different types of data. This flexibility makes it a versatile tool for future multimodal AI applications.
In summary, ImageBind by Meta integrates multiple sensory inputs into a single embedding space, enabling holistic learning and cross-modal alignment without explicit supervision. This approach opens up new possibilities for creative content generation, enhanced analytical capabilities, and improved performance in various AI tasks.
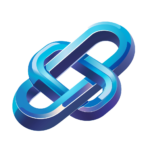
ImageBind by Meta - Performance and Accuracy
Performance and Accuracy of ImageBind
ImageBind, developed by Meta, has demonstrated impressive performance and accuracy in various multi-modal tasks, particularly in the integration of different data types such as text, audio, video, 3D, thermal, and motion.Accuracy in Multi-Modal Tasks
- ImageBind has achieved significant improvements in accuracy, especially in few-shot classification tasks. For instance, it outperformed specialist models for audio classification by approximately 40% in top-1 accuracy.
- In depth classification, ImageBind also showed improved performance on zero-shot recognition tasks compared to specialist models.
- The model’s ability to learn from naturally paired self-supervised data allows it to align and recognize content across different modalities effectively, even with limited training data.
Multi-Modal Integration
- ImageBind can bind data from six different modalities without the need for explicit supervision. It uses images as a bridge to connect these modalities, enabling the model to recognize and link content comprehensively.
- This integration allows ImageBind to perform tasks such as linking audio and text, or estimating the depth of a scene from a picture, with high accuracy.
Scalability and Adaptability
- As ImageBind grows larger, it gains new abilities that were not present in smaller models. This strong scaling behavior enables it to use other modalities to replace or improve various AI models.
- For example, ImageBind can redesign a text-to-image tool to create images using sound, such as laughter or rain.
Limitations and Areas for Improvement
- Despite its impressive performance, ImageBind is still primarily for research purposes. It faces challenges such as the need for extensive computational resources and large-scale data.
- The model’s performance can be limited by the quality and availability of the data used for training. Modalities that are not visually related, like sound and IMU readings, have a more vulnerable relationship with images, which can affect accuracy.
- While ImageBind can accommodate additional data modalities by identifying information present in the data, it may not always be able to handle new modalities seamlessly without further fine-tuning or additional data.
Conclusion
ImageBind by Meta AI has set new benchmarks in multi-modal AI by achieving high accuracy and performance across various tasks. However, it is important to acknowledge its current limitations, particularly in terms of computational resources and data quality. As the model continues to evolve, addressing these limitations will be crucial for its broader application and improvement.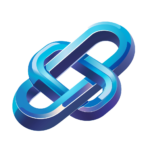
ImageBind by Meta - Pricing and Plans
Pricing
- The ImageBind demo on MetaDemoLab is priced at $0.50 per request. This pricing is part of a flexible plan that allows users to select options based on their specific needs and the volume of requests they intend to make.
Plans and Features
- There are no explicitly defined tiers or plans beyond the per-request pricing. Users pay based on the number of requests they make, which allows for a high degree of flexibility.
- The key features available include:
- Multimodal AI Model: Processes and analyzes data from multiple sensory inputs simultaneously.
- Single Embedding Space: Integrates various modalities into a unified representation.
- Zero-Shot and Few-Shot Learning: Recognizes patterns or objects it has not seen during training.
- Cross-Modal Search and Generation: Performs searches and generates content across different modalities.
- Interactive Demo: Allows users to experience the capabilities of ImageBind firsthand.
Free Options
- There is no mention of a free plan or free tier for using ImageBind. However, users can access an interactive demo to experience the capabilities of ImageBind before committing to paid requests.
Summary
In summary, the pricing for ImageBind by Meta is based on a pay-per-request model, with no free or tiered plans currently available beyond the demo.
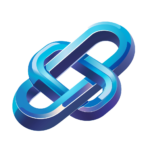
ImageBind by Meta - Integration and Compatibility
ImageBind by Meta
ImageBind, an advanced AI model developed by Meta AI, integrates with other tools and platforms in several key ways, enhancing its compatibility and utility across various applications.
Integration with Weaviate
ImageBind can be seamlessly integrated with Weaviate, a database that supports vector search. This integration allows users to leverage ImageBind’s embedding models directly from the Weaviate database. By spinning up the ImageBind model in a container, users can host their own models and use them with Weaviate for semantic and hybrid search operations without additional preprocessing or data transformation steps.
Multi-Modality Support
One of the standout features of ImageBind is its ability to combine data from six different modalities: images and video, text, audio, thermal imaging, depth sensor readings, and IMU (Inertial Measurement Unit) readings. This multi-modality support enables the model to create a single joint embedding space, allowing data from different modalities to be aligned and processed together. This integration facilitates advanced information retrieval across modalities and supports zero-shot and few-shot classification.
Open-Source and Accessibility
ImageBind is an open-source project, making it accessible for developers to build their own classifiers and information retrieval systems. The model and its accompanying weights are licensed under a CC-BY-NC 4.0 license, and there are examples provided in the project README on how to feed text, image, and audio data into ImageBind. This openness allows for widespread adoption and customization across different platforms and devices.
Compatibility with Other Models
ImageBind can be used in conjunction with other AI models to enhance their capabilities. For instance, it can be integrated with models like DINOv2 for zero-shot image segmentation or with generative AI models to create new types of immersive experiences. By using image-paired data as a bridge, ImageBind can connect modalities that do not typically co-occur, enabling other models to “understand” new modalities without extensive training.
Cross-Platform Usage
While specific details on device-level compatibility are not provided, the model’s open-source nature and its ability to be hosted in containers suggest that it can be deployed on various platforms that support containerization. This flexibility makes it possible to use ImageBind on cloud services, local servers, or edge devices, depending on the requirements of the application.
Conclusion
In summary, ImageBind’s integration capabilities, multi-modality support, and open-source nature make it highly compatible and versatile across different tools, platforms, and devices, enabling developers to build sophisticated AI-driven applications with ease.
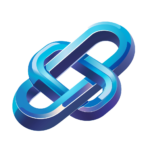
ImageBind by Meta - Customer Support and Resources
Support and Resources for ImageBind by Meta
Open-Source Availability
ImageBind is an open-source model, which means the code and model weights are freely available for use. This is licensed under a CC-BY-NC 4.0 license, allowing users to experiment and build upon the model.
Documentation and Code
The project includes a detailed README file that provides examples on how to feed text, image, and audio data into ImageBind. This documentation helps users get started with integrating the model into their own projects.
Interactive Playground
Meta Research has published an interactive playground for ImageBind, which offers pre-made examples to demonstrate information retrieval across different modalities. This playground is a valuable resource for testing and exploring the capabilities of the model.
Community and Support
While there may not be a dedicated customer support hotline or forum specifically for ImageBind, the open-source nature of the project encourages community involvement. Users can engage with the broader AI and machine learning community to share insights, ask questions, and collaborate on projects using ImageBind.
Practical Applications and Examples
The resources provided include several examples of how ImageBind can be used in practical applications, such as creating a search engine that retrieves images based on audio inputs, or generating images from text prompts and enhancing them with audio clips. These examples are detailed in the blog posts and the interactive playground.
Integration with Other Models
ImageBind can be integrated with other AI models, such as DINOv2 and Segment Anything (SAM), to enhance their capabilities. For instance, using ImageBind with Detic can improve audio and image classification tasks.
Conclusion
Overall, the support and resources for ImageBind are centered around its open-source availability, comprehensive documentation, and the interactive playground, which collectively facilitate a strong foundation for users to explore and utilize the model’s multimodal capabilities.
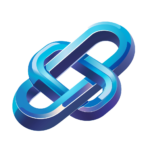
ImageBind by Meta - Pros and Cons
Advantages of ImageBind by Meta
Holistic Learning
ImageBind is the first AI model to learn a single embedding space that connects data from six distinct modalities, including text, images/videos, audio, 3D measurements, temperature data, and motion data. This holistic approach allows machines to analyze different kinds of data in a way that mimics human sensory integration.
Multimodal Capabilities
By binding multiple modalities, ImageBind enables various innovative applications such as cross-modal retrieval, multimodal arithmetic, cross-modal detection, and cross-modal production. For example, it can add the perfect audio clip to a video recording or generate depth models from images.
Improved Performance
ImageBind outperforms earlier specialized models in few-shot and zero-shot recognition tasks across modalities. It achieved significant gains, such as a 40% improvement in top-1 accuracy for few-shot audio and depth classification compared to Meta’s AudioMAE models.
Efficient Training
Unlike traditional multimodal learning methods, ImageBind does not require explicit supervision or paired data across all modalities. It leverages naturally paired self-supervised data and visual representations from large-scale web data to align different modalities.
Open-Source
ImageBind is an open-source project, allowing researchers and developers to experiment with their own data and explore its capabilities, which can foster further innovation and community engagement.
Enhanced Accessibility
ImageBind has the potential to aid people with vision or hearing impairments by providing real-time multimedia descriptions, enhancing their ability to understand their environment.
Disadvantages of ImageBind by Meta
Limitations in Real-World Applications
Currently, ImageBind is not recommended for real-world applications due to potential biases and unintended associations learned during training. It is still considered a research prototype.
Lack of Established Baselines
Given its novelty, there are no fair baselines to compare ImageBind’s performance against, making it challenging to evaluate its effectiveness comprehensively.
Training and Alignment Challenges
While ImageBind can align modalities that co-occur with images, modalities that are not visual (like audio and IMU data) have a weaker correlation, which can make alignment more challenging.
Data Restrictions
ImageBind is limited to the data it was trained on and cannot generate new images or data that were not present in the original dataset.
By considering these points, users can better understand the capabilities and limitations of ImageBind and how it can be utilized effectively within the analytics and AI-driven product category.
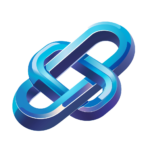
ImageBind by Meta - Comparison with Competitors
Unique Features of ImageBind
- Multimodal Integration: ImageBind is distinct in its ability to integrate six types of data: visual (images and videos), thermal (infrared), text, audio, depth information, and movement readings from inertial measuring units (IMU). This holistic approach allows the model to learn a single embedding space across multiple modalities, enabling machines to analyze and connect different forms of information more like humans do.
- Cross-Modal Alignment: Unlike traditional models, ImageBind does not require all modalities to appear concurrently within the same datasets. It leverages the natural pairing of images with other modalities to create a joint embedding space, allowing different modalities to “talk” to each other without needing explicit paired data.
- Zero-Shot and Few-Shot Learning: ImageBind excels in zero-shot and few-shot learning tasks, outperforming specialist models in tasks such as audio and depth classification. This capability is particularly useful for tasks where extensive labeled data is not available.
Potential Alternatives
AI Analytics Tools for Data Visualization and Analysis
- Tableau: While Tableau is primarily a data visualization and analytics platform, it does not integrate multiple modalities like ImageBind. However, it offers AI-powered recommendations, predictive modeling, and natural language processing, making it a strong tool for data analysis and visualization.
- Microsoft Power BI: Power BI is another powerful analytics tool that integrates with Microsoft Azure for advanced analytics and machine learning. It provides interactive visualizations and data modeling but does not handle multimodal data integration like ImageBind.
Specialized AI Models
- Google’s Cloud AI Platform: This platform offers a comprehensive suite of machine learning tools but is more focused on general machine learning tasks rather than multimodal integration. It is ideal for businesses already invested in the Google ecosystem but lacks the specific multimodal capabilities of ImageBind.
Multimodal AI Models
- GenAI by Meta: While GenAI is another AI model from Meta, it does not have the same multimodal integration capabilities as ImageBind. GenAI is more generalized and does not focus on binding multiple modalities into a single embedding space.
Key Differences
- Modalities Handled: ImageBind’s ability to handle six different modalities sets it apart from other AI analytics tools, which typically focus on text, images, or other single modalities.
- Training Requirements: ImageBind’s approach to using image-paired data to align different modalities reduces the need for extensive paired datasets, making it more feasible for multimodal learning.
- Applications: ImageBind’s multimodal capabilities open up new possibilities for applications such as generating images from audio, enhancing videos with appropriate audio clips, and creating immersive experiences by combining multiple modalities.
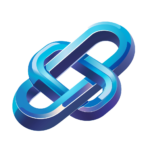
ImageBind by Meta - Frequently Asked Questions
What is ImageBind?
ImageBind is an embedding model developed by Meta Research that combines data from six different modalities: images and video, text, audio, thermal imaging, depth, and inertial measurement units (IMUs).
How does ImageBind work?
ImageBind works by training a large embedding model using pairs of data that map image data to other modalities. For example, it uses image-audio pairings and image-thermal pairings. This approach allows the model to create a joint embedding space where all modalities are represented in a single vector space, enabling direct comparison and combination of different modalities.
What modalities does ImageBind support?
ImageBind supports six modalities:
- Images and video
- Text
- Audio
- Thermal imaging (infrared images)
- Depth information (3D data)
- Inertial measurement units (IMUs) which record motion and position.
What are the practical applications of ImageBind?
ImageBind can be used for various applications, including:
- Information retrieval across different modalities: For instance, searching for audio materials associated with an image or finding images related to an audio clip.
- Zero-shot and few-shot classification: ImageBind can classify data from different modalities without or with very few examples.
- Generative AI: It can be used to generate images or videos based on audio input, and enhance object detection models by incorporating audio data.
How does ImageBind handle data from different modalities?
ImageBind does not require all modalities to appear concurrently within the same datasets. Instead, it leverages the natural pairing of images with other modalities to create a unified representation space. This allows the model to align and compare data from different modalities even if they were not observed together during training.
Is ImageBind open source?
Yes, ImageBind is open source. The model and its accompanying weights are available on GitHub, licensed under a CC-BY-NC 4.0 license. This allows researchers and developers to use and experiment with the model.
How can I get started with ImageBind?
To get started, you can use the ImageBind playground published by Meta Research, which provides pre-made examples for information retrieval. You can also refer to the project README on GitHub, which includes examples of how to feed text, image, and audio data into ImageBind.
What are the benefits of using ImageBind over traditional models?
ImageBind outperforms prior specialist models by enabling machines to analyze and combine multiple forms of information together. It does not require explicit supervision or paired data for all modalities, making it more feasible to train and use.
Can ImageBind be used with other AI models?
Yes, ImageBind can be combined with other AI models. For example, Meta used ImageBind embeddings with the object detection model Detic and the generative AI model DALLE-2 to enhance their capabilities.
How does ImageBind improve performance in classification tasks?
ImageBind achieves significant gains in zero-shot and few-shot classification. It realized approximately 40 percent accuracy improvement in top-1 accuracy on ≤four-shot classification compared to other models like AudioMAE.
What future possibilities does ImageBind open up?
ImageBind opens up possibilities for more accurate content recognition, moderation, and creative design. It can also be used to generate richer media content and create immersive virtual environments by combining data from multiple modalities.
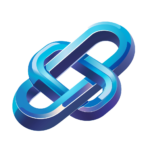