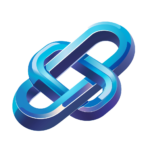
Verta - Detailed Review
Analytics Tools
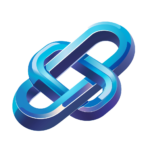
Verta - Product Overview
Overview
Verta is an Operational AI company that specializes in helping enterprises deploy, manage, and govern artificial intelligence and machine learning models effectively. Here’s a brief overview of its primary function, target audience, and key features:Primary Function
Verta’s main goal is to enable companies to safely and efficiently deploy, monitor, and manage machine-learning models. This involves ensuring the models are reliable, scalable, and compliant with regulatory requirements, particularly in highly regulated industries such as healthcare, finance, and insurance.Target Audience
Verta’s tools are primarily aimed at data scientists, ML engineers, DevOps teams, and other stakeholders involved in the machine learning operations (MLOps) cycle within enterprises. The platform is particularly beneficial for large companies and high-growth tech firms looking to accelerate the deployment of AI-enabled solutions while maintaining governance and compliance.Key Features
Model Management and Deployment
Verta’s platform allows users to package and deliver any ML model using best-in-class DevOps support for continuous integration and deployment (CI/CD), operations, and monitoring. This ensures safe, reliable, and scalable real-time AI deployments.Version Control and Collaboration
The Verta Model Catalog provides version control, enabling teams to track changes, compare different versions, and revert to previous states if needed. It also facilitates collaboration by allowing teams to organize and document models in a centralized repository.Model Governance and Compliance
The platform supports model governance by providing features such as access controls, comprehensive documentation, and auditing capabilities. This helps organizations ensure regulatory compliance and maintain transparency throughout the model lifecycle.Performance Optimization and Monitoring
Verta’s tools help in optimizing model performance, scaling, and cycle time, which is how quickly a model can be turned into a valuable product. The platform also includes deployment checklists and security scans to vet models before deployment.Generative AI Support
Verta recently launched the GenAI Workbench, an all-in-one platform that accelerates the development of Generative AI applications. It supports various large language models, prompting techniques, and a leaderboard to rank model and prompt configurations based on different dimensions like quality, cost, and latency.Conclusion
Overall, Verta’s solutions are geared towards streamlining the entire ML workflow, ensuring that AI models are deployed efficiently, safely, and in compliance with regulatory standards.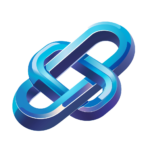
Verta - User Interface and Experience
User Interface Overview
The user interface of Verta AI, particularly in the analytics tools and AI-driven product category, is designed to be user-friendly and efficient for data scientists and machine learning practitioners.Logging In and Home Page
When users log into the Verta AI platform, they are greeted with a home page that serves as a welcome and instruction hub for first-time users. This page includes quick links to onboarding videos and essential resources to help users get started quickly. For returning users, the home page provides a snapshot of activities and updates in their workspace since their last login, including the status of running models, predictions made, and monitoring details.Data Registration
The platform allows data scientists to register and manage datasets used for model training and testing. This feature enables data provenance tracking, ensuring reproducibility and traceability throughout the model development process. Users can register versions of datasets and connect them to experiments and models, providing complete visibility into the data used.Running Experiments and Model Selection
Verta AI facilitates running experiments to compare and select the best-performing models. The model catalog acts as a central hub for managing models throughout their lifecycle, making it easier to evaluate, select, and deploy models.Model Deployment and Monitoring
Model deployment is streamlined, with options for creating endpoints and downloading containerized versions of the models. Once deployed, the platform offers monitoring capabilities to track the performance of the models in real-time. This includes tracking accuracy metrics such as precision, recall, and F1-score, as well as automatic alerting for drift detection and data outliers.Automation Capabilities
Verta AI also provides automation capabilities through web hook systems, enabling seamless integration and automation based on model catalog or monitoring activities. This feature helps in automating various tasks and integrating the platform with external systems.Ease of Use and User Experience
The interface is designed to be intuitive, allowing data scientists to efficiently manage their machine learning projects from data registration to model deployment. The platform offers a seamless workflow for the entire model lifecycle, making it easier for users to get started and manage their projects effectively. The availability of onboarding resources, quick links, and a centralized view of important information on the home page further enhances the ease of use.Collaboration and Support
Verta AI enhances collaboration among data science teams by providing a centralized platform where team members can manage and monitor models together. The platform also offers comprehensive support and assistance through various resources, including help icons and support emails, ensuring that users can get the help they need quickly.Conclusion
Overall, Verta AI’s user interface is structured to be user-friendly, efficient, and supportive, making it an effective tool for data scientists and machine learning practitioners to build, deploy, and manage AI models.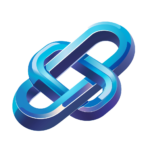
Verta - Key Features and Functionality
Verta Overview
Verta, an AI model management and operations company, offers a suite of tools and features that are integral to the development, deployment, and management of artificial intelligence and machine learning models. Here are the main features and functionalities of Verta’s products:Verta GenAI Workbench
The Verta GenAI Workbench is a comprehensive platform aimed at accelerating the development of Generative AI (GenAI) applications. It combines automated machine learning and human feedback to help builders quickly experiment with and refine custom Large Language Model (LLM) solutions. Key features include:Support for Various LLMs
The Workbench supports a range of open-source and proprietary LLMs, such as Llama 2, Mistral, Falcon, OpenAI’s GPT models, and Google’s PaLM.Starter Kits
It provides pre-built baseline prompts for various use cases, leveraging state-of-the-art prompting techniques and GenAI itself.GenAI Leaderboard
This feature ranks model and prompt configurations based on application-specific quality, cost, and latency, helping users select the best solution for their needs.Verta Model Catalog
The Verta Model Catalog is a central repository for managing, organizing, and documenting AI and ML models. Its key features include:Model Organization and Documentation
It allows teams to organize and document models in a structured and searchable inventory, making it easy to find and track relevant information.Version Control and Collaboration
The catalog supports version control, enabling teams to manage multiple versions of models, track changes, and collaborate effectively.Model Metadata and Documentation
Teams can store and manage essential metadata and documentation for each model, including model description, input/output specifications, and performance metrics.Model Permissions and Access Control
The catalog provides granular controls for managing permissions and access to models, ensuring secure and controlled access.Model Search and Discovery
It facilitates efficient model discovery through search capabilities based on various criteria such as model name, tags, metadata, or performance metrics.MLOps Capabilities
Verta’s platform is focused on ML Operations (MLOps), integrating ML development and delivery into regular operations. Key features include:Model Versioning and Metadata
Verta allows for the management of model versions and associated metadata, ensuring transparency and traceability.Model Deployment and Release
The platform supports the deployment and release of models, making it easier to operationalize ML models.Real-time Model Monitoring
Verta provides real-time monitoring of deployed models, enabling continuous model improvement and maintenance.Experiment Management
It includes features for logging, tracking, and visualizing experiments, which helps in organizing, filtering, and comparing different experiments.Collaboration and Security
The platform offers role-based access controls, deep links into assets, and customizable experiment reporting, ensuring secure and collaborative workflows.Integration and Deployment
Verta’s solutions are designed to be versatile and can be deployed across various environments:Multi-Cloud Support
The Verta GenAI Workbench and other tools are available on AWS, Azure, GCP, as well as on-premise deployments.Hybrid Data Lakehouse
With the acquisition by Cloudera, Verta’s technology integrates with Cloudera’s hybrid open data lakehouse, enabling unified governance and security across on-premises and multiple cloud environments.AI Integration
Verta’s products heavily integrate AI to streamline the development and deployment of AI models:Automated Machine Learning
The GenAI Workbench uses automated machine learning to help builders rapidly experiment with and refine custom LLM solutions.AI-Driven Model Management
Verta’s Model Catalog and MLOps platform leverage AI to manage the lifecycle of AI models, from development to deployment and monitoring. These features collectively enable data science and IT operations teams to focus on their strengths, rapidly bring AI/ML advances to market, and ensure the efficient management and operationalization of AI models.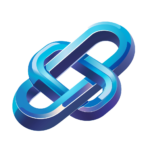
Verta - Performance and Accuracy
Performance Monitoring
Verta is highly effective in monitoring the performance of machine-learning models. It automatically tracks key metrics such as accuracy, precision, recall, and F1 score. The platform also monitors operational metrics like response time, latency, error rate, and throughput, ensuring that models are performing as intended.
Model Validation and Testing
Verta enables thorough model validation to identify any discrepancies or edge cases that may require manual processing or further review. This includes running models through deployment checklists and security scans to ensure they are functioning correctly and securely.
Data Quality and Drift
The platform is capable of monitoring data quality and drift, which is crucial for maintaining model accuracy over time. Alerts can be set up to notify teams of any performance degradation or data drift, allowing for prompt corrective actions.
Governance and Compliance
Verta supports strong governance and compliance practices by enabling companies to set up explainability and bias checks as part of the model release process. This ensures compliance with Ethical AI standards and upcoming regulations such as the EU AI Act and the American Data Privacy and Protection Act.
Automation of Governance Processes
While Verta offers significant automation in AI governance processes, such as bias detection and mitigation, model explainability, and transparency, the current state of many companies using Verta shows a gap. According to Verta’s own research, close to 90% of companies have little or no automation in place for these processes, and only 10% have fully or highly automated their model documentation process.
Limitations and Areas for Improvement
One of the main limitations is the lack of widespread automation in AI governance processes among companies using Verta. This indicates that while Verta provides the necessary tools, many organizations are not fully leveraging them to automate critical compliance tasks. Additionally, the high time and resource requirements for tasks like algorithm impact assessments (with a median time of 40 hours per model) highlight the need for further streamlining and automation to support scalability.
Industry-Specific Applications
Verta has been successful in helping companies across various industries, including healthcare, finance, and insurance, to deploy and manage AI models responsibly. For instance, healthcare companies can use Verta to improve AI-powered patient monitoring and treatment recommendations, ensuring these systems are thoroughly vetted for errors and biases.
Conclusion
In summary, Verta demonstrates strong capabilities in performance monitoring, model validation, and compliance, but there is room for improvement in terms of automating governance processes and reducing the time and resources required for regulatory compliance.
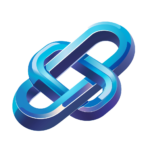
Verta - Pricing and Plans
Verta’s Token Pricing Model for Marketing Services
Verta’s marketing services, although not directly related to their AI analytics tools, provide insight into their innovative pricing approach. This model is based on a token system:
- Verta offers four-tier packages: Starter, Basic, Pro, and Custom.
- Each package includes a fixed number of tokens that clients can use to purchase various marketing services such as Social Media Marketing, Search Engine Optimization, Commercial Video Production, and Graphic Design.
- The valuation of each token is roughly equivalent to 1 hour of agency work.
- Clients have the flexibility to decide how many tokens to use each month, but tokens expire and cannot be carried forward.
Verta Model Catalog for AI and Machine Learning
For their AI and Machine Learning products, specifically the Verta Model Catalog:
- Verta has introduced a Free Tier Program that allows data scientists to manage their machine learning models at no cost.
- This free tier enables users to register and share an unlimited number of models, along with relevant metadata and documentation, in a secure central catalog.
- The free tier supports collaboration among data scientists and other stakeholders across the ML lifecycle, promoting operational excellence and AI governance.
Features and Plans
Free Tier
- Allows data scientists to manage all their ML models in a centralized hub.
- Includes features for organizing, managing, and monitoring ML assets.
- Supports collaboration and provides a 360-degree view of the ML model portfolio.
Paid Plans
While the free tier is well-documented, specific details about the features and pricing of the paid plans are not readily available in the provided sources. It is recommended to visit Verta’s official website or contact their sales team for detailed information on the paid tiers and their corresponding features.
In summary, Verta’s pricing structure for their AI analytics tools, such as the Model Catalog, includes a free tier with significant capabilities, but detailed pricing for their paid plans is not specified in the available resources.
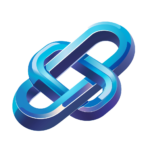
Verta - Integration and Compatibility
Integration and Compatibility of Verta
When discussing the integration and compatibility of Verta, particularly in the context of its AI-driven product category, several key points stand out:Integration with Cloudera
Verta’s Operational AI Platform has been acquired by Cloudera, a move that significantly enhances Cloudera’s AI capabilities. This integration allows Verta’s hybrid and multi-cloud end-to-end AI platform to work seamlessly with Cloudera’s data management infrastructure. Cloudera, which manages a substantial portion of the world’s data, provides an ideal environment for Verta’s tools to operate. This synergy enables the creation, operationalization, monitoring, security, and scaling of AI models across enterprises, leveraging Cloudera’s open data lakehouse approach that integrates analytics and AI with business data both on-premises and across multiple clouds.Platform Capabilities
Verta’s platform is built to simplify the deployment and management of AI models. It includes features such as a genAI workbench, model catalogue, and AI governance tools. These tools allow developers, regardless of their machine learning expertise, to turn datasets into custom retrieval-augmented generation applications. This integration ensures that AI models are deployed responsibly, with checks for performance, compliance, and governance.Cross-Platform Compatibility
Verta’s technology is designed to be versatile and compatible across various environments. It supports both predictive and generative AI (GenAI) models and can operate in hybrid and multi-cloud settings. This flexibility makes it suitable for a wide range of enterprise applications, from chatbots and document summarization to code generation, all while maintaining unified governance and security.Data Science Workflows
Verta’s platform integrates well with data science workflows, allowing data scientists to track different versions of models, understand how they were built, and run deployment checklists and security scans. This ensures that AI models are not only deployed quickly but also continue to work as intended over time.Conclusion
In summary, Verta’s integration with Cloudera and its inherent platform capabilities make it highly compatible and effective across various enterprise environments, supporting a wide range of AI applications and ensuring responsible model deployment and management.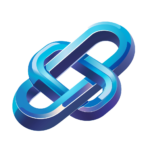
Verta - Customer Support and Resources
Customer Support
- Verta provides email support, which can be accessed through their contact email:
support@verta.ai
. - Users can also engage with Verta’s support team through online chat support, available via the Verta online portal.
Additional Resources
- Documentation and Guides: Verta offers detailed documentation and guides on its platform, including information on model versioning, deployment, and real-time monitoring. These resources help users manage their ML models effectively.
- Community and Forums: Verta has a community presence on platforms like LinkedIn, Twitter, and Slack, where users can connect with other users, ask questions, and share experiences.
- Blog and News: The company maintains a blog where they publish updates, insights, and news about their platform and the broader AI and ML landscape.
- GitHub: Verta’s open-core platform is supported by open-source technology, and users can find relevant code and contributions on GitHub.
Free Tier Program and Model Catalog
- Verta offers a Free Tier Program for its Model Catalog, allowing data scientists to manage all their ML models at no cost. This includes features like secure model registration, version tracking, and sharing models with colleagues. The Model Catalog also provides one-click scanning for vulnerabilities and configurable governance checklists to ensure compliance with AI regulations.
Enterprise Support
- For enterprise customers, Verta provides additional support features such as enterprise security, governance, and teamwork and collaboration tools. This includes customized checklists, audit logs, and centralized access to training data to ensure compliance and operational excellence.
By leveraging these support options and resources, users can effectively manage their machine learning operations and ensure their models are deployed safely and efficiently.
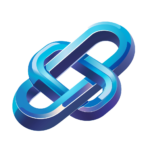
Verta - Pros and Cons
Advantages
Simplification of AI Deployments
Verta’s Operational AI Platform is designed to address significant hurdles in AI deployments by enabling organizations to effectively build, operationalize, monitor, secure, and scale models throughout the organization.
Acceleration of GenAI Application Development
The platform accelerates the development of General AI (GenAI) applications, making it easier for developers, regardless of their machine learning expertise, to create and optimize business-ready large language models (LLMs).
Streamlined Process
Verta simplifies the process of turning private datasets into custom retrieval-augmented generation applications, which can be a significant advantage for organizations looking to leverage AI efficiently.
Enhanced Enterprise AI Capabilities
The acquisition of Verta by Cloudera is expected to deepen Cloudera’s AI technology portfolio and expertise, providing customers with advanced tools for their data and AI initiatives.
Disadvantages
While the specific sources provided do not detail direct disadvantages of Verta’s platform, some general considerations can be inferred from the broader context of AI analytics tools:
Data Quality Issues
AI analytics tools, including those like Verta, are only as good as the data they are fed. Poor or incomplete data can lead to unreliable insights, which is a common challenge in AI-driven analytics.
Integration Challenges
Ensuring that the tool integrates seamlessly with other key data tools and systems is crucial. Any difficulties in integration could hinder the effectiveness of the platform.
Need for Human Oversight
While AI tools automate many tasks, they cannot replace the critical thinking capabilities of humans. There is a need for ongoing human interpretation and validation of AI-generated insights to ensure they align with organizational goals.
Given the limited specific information available directly about Verta’s platform, these points are derived from the broader context of AI analytics tools and may not be exclusively applicable to Verta. For the most accurate and detailed information, it would be best to consult Verta’s official resources or contact their support team.
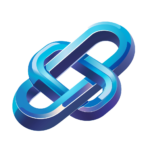
Verta - Comparison with Competitors
Unique Features of Verta
- Model Management and Lifecycle: Verta is specifically focused on the entire AI and ML model lifecycle, including experiment tracking, production registry, deployment, inference, and monitoring. This comprehensive approach sets it apart from tools that focus solely on data analytics or visualization.
- Integration and Agnosticity: Verta integrates with a wide range of ML frameworks and data preparation/training platforms such as TensorFlow, PyTorch, Scikit-learn, XGBoost, and more. It also integrates with standard DevOps tools like GitHub, Jenkins, and Kafka. This agnosticity makes it highly versatile and adaptable to various enterprise ecosystems.
- Security and Access Control: Verta features a robust Role-Based Access Control (RBAC) system and integrates with enterprise identity systems like Okta and Active Directory. It also supports Single Sign-On (SSO) and automated user provisioning, ensuring secure and controlled access to the platform.
- Deployment Flexibility: Verta offers multiple deployment options, including a fully managed SaaS service, on-premise deployment, and a managed service option where updates and operational metrics are managed without access to the models.
Potential Alternatives and Comparisons
Google Cloud AI Platform
- Unlike Verta, Google Cloud AI Platform is more broadly focused on data science and machine learning, with a larger customer base and market share. It integrates well with other Google Cloud services but may not offer the same level of model lifecycle management as Verta.
Tableau and Microsoft Power BI
- These tools are more focused on data visualization and business intelligence. Tableau and Power BI provide interactive dashboards and predictive modeling capabilities but do not manage the ML model lifecycle in the same way Verta does. They are better suited for data analysis and visualization rather than end-to-end ML model management.
Salesforce Einstein Analytics
- This platform is integrated within the Salesforce ecosystem and focuses on analyzing customer data to predict sales outcomes and personalize marketing campaigns. While it uses AI and machine learning, it is more specialized towards CRM and customer data analysis, unlike Verta’s broader ML model management capabilities.
Qlik and IBM Cognos Analytics
- Qlik and IBM Cognos Analytics are data analytics platforms that use AI for associative analysis and automated pattern detection. They are more geared towards general data analysis and business intelligence rather than the specific needs of ML model management and lifecycle tracking that Verta addresses.
Verta GenAI Workbench
- Verta also offers the GenAI Workbench, which is specifically designed for generative AI development. This tool simplifies the process of building GenAI applications by supporting various open-source and proprietary models and providing features like the GenAI Leaderboard to rank model and prompt configurations. This is a unique offering within the Verta suite that sets it apart from more general analytics tools.
Conclusion
In summary, Verta stands out due to its comprehensive approach to ML model management, its integration capabilities, and its flexible deployment options. While other tools excel in data visualization, business intelligence, and specific analytics tasks, Verta is uniquely positioned to manage the entire lifecycle of AI and ML models.
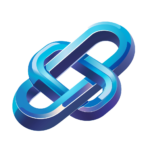
Verta - Frequently Asked Questions
Frequently Asked Questions about Verta
What is Verta and what does it do?
Verta is a platform that provides model management and operations solutions for the entire AI and ML model lifecycle. It helps companies deploy, monitor, and manage machine-learning models safely and at scale. Verta’s platform includes features such as experiment tracking, production registry, deployment, inference, serving, and monitoring.How does Verta support the AI model lifecycle?
Verta supports the entire AI model lifecycle, from development to production. It allows data scientists to track different versions of models, understand how they were built, and run them through deployment checklists and security scans. The platform also includes performance optimizations, scaling, and governance to ensure models continue working as intended over time.Is Verta compatible with various ML frameworks and tools?
Yes, Verta is agnostic to any ML framework and integrates with a wide range of ML frameworks and data preparation/training platforms, including TensorFlow, PyTorch, Scikit-learn, XGBoost, R, Spark, ONNX, PMML, Kubeflow, Anaconda, and more. It also integrates with standard DevOps tools like GitHub, PyPI, Jenkins, Kafka, and Datadog.What deployment options are available for Verta?
Verta offers several deployment options, including a fully managed SaaS service, on-premise deployment, and a managed service option where Verta manages the deployment and provides 24/7 support without access to the models. There is also an option for fully air-gapped deployments.How does Verta handle security and access control?
Verta supports a robust Role-Based Access Control (RBAC) system and integrates into enterprise identity systems such as Okta and Active Directory. This allows for the definition of roles and permissions and the ability to audit activity on the platform. Single Sign-On (SSO) and automated user provisioning are also supported.What is the Verta GenAI Workbench?
The Verta GenAI Workbench is an all-in-one platform designed to accelerate the development of Generative AI (GenAI) applications. It uses a combination of automated machine learning and human feedback to help builders quickly experiment with and refine custom Large Language Model (LLM) solutions. The Workbench supports various open-source and proprietary LLMs and provides starter kits with strong baseline prompts for different use cases.How does Verta ensure compliance and governance?
Verta’s platform includes features to ensure compliance and governance, such as the ability to vet models by running them through deployment checklists and security scans. It also provides tools for managing machine learning assets throughout the ML lifecycle, ensuring safe, reliable, and scalable real-time AI deployments.Can Verta be deployed on different cloud platforms?
Yes, Verta can be deployed on various cloud platforms, including AWS, Azure, and Google Cloud, as well as on-premise deployments. The platform is Kubernetes-based and can be deployed on any Kubernetes cluster, whether in the cloud or on-premise.What kind of support does Verta offer?
Verta offers 24/7 support for its managed service options, where the team can manage deployments, push updates, and provide operational metrics without accessing the models. This ensures continuous support and maintenance of the AI models.How does Verta integrate with enterprise ecosystems?
Verta integrates with all the standard DevOps tools within the enterprise ecosystem, such as GitHub, PyPI, Jenkins, Kafka, and Datadog. It also supports integration with enterprise identity systems like Okta and Active Directory, enabling seamless integration into existing workflows.What is the current status of Verta in terms of its ownership?
As of recent updates, Cloudera has acquired Verta, aiming to enhance its platform capabilities by integrating Verta’s Operational AI technology, including its model catalog, GenAI workbench, and AI governance tools.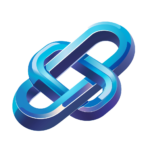
Verta - Conclusion and Recommendation
Final Assessment of Verta in the Analytics Tools AI-Driven Product Category
Verta is a formidable player in the AI-driven analytics tools market, particularly for organizations looking to manage, deploy, and monitor machine learning (ML) models efficiently and securely. Here’s a breakdown of its key benefits and who would most benefit from using it:Key Benefits
Speed and Efficiency
Verta significantly reduces the time it takes to deploy ML models into production. For instance, it can decrease deployment time by orders of magnitude, allowing companies to deploy models multiple times a month instead of every six months.
Security and Compliance
Verta enhances enterprise security, privacy, and access controls. It includes standardized vulnerability scanning at multiple points of the ML lifecycle, ensuring models are secure from the outset.
Governance and Fairness
The platform helps companies audit models for bias and ensures they are explainable and fair, which is crucial for highly regulated industries like healthcare and finance.
Scalability and Integration
Verta provides simplified and scalable model deployment and inference serving for batch, real-time, and streaming ML applications. It integrates well with popular data stream systems like Apache Kafka.
Model Management
Verta’s platform allows data scientists to track different versions of models, monitor their performance, and manage them for compliance and governance.
Who Would Benefit Most
Enterprise Data Science Teams
Teams in large organizations, especially in sectors like healthcare, finance, and insurance, would greatly benefit from Verta. It helps them manage the operational burdens associated with ML model deployment, allowing them to focus more on strategic data science tasks.
High-Growth Tech Companies
Companies looking to quickly deploy new AI-enabled solutions while ensuring they are used appropriately will find Verta’s tools invaluable. It helps in speeding up the deployment process while maintaining the integrity and safety of the models.
Regulated Industries
Organizations in highly regulated industries will appreciate Verta’s emphasis on governance, compliance, and fairness in ML models. This ensures their AI systems meet the necessary standards and regulations.
Overall Recommendation
Verta is highly recommended for any organization seeking to streamline and secure their ML model deployment and management processes. Its ability to reduce deployment time, enhance security, and ensure compliance makes it an essential tool for data science teams and companies looking to leverage AI effectively. With its strong focus on responsible AI practices, Verta helps companies build and deploy AI models safely and at scale, which is crucial for maintaining trust and integrity in AI-driven solutions.