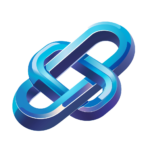
Whisper (OpenAI) - Detailed Review
Audio Tools
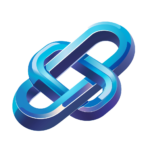
Whisper (OpenAI) - Product Overview
Introduction to OpenAI Whisper
OpenAI Whisper is an advanced Automatic Speech Recognition (ASR) system developed by OpenAI, released in September 2022. Here’s a brief overview of its primary function, target audience, and key features:Primary Function
Whisper’s primary function is to transcribe spoken language into written text with high accuracy. It can handle a wide range of audio inputs, including various languages, accents, and noisy environments. Additionally, Whisper can translate speech from its supported languages into English text.Target Audience
The primary intended users of Whisper are AI researchers, developers, and organizations looking for a reliable ASR solution. It is particularly useful for those studying robustness, generalization, capabilities, biases, and constraints of ASR models. Developers can also leverage Whisper for various applications, including meeting transcriptions, voice assistants, and automatic captioning.Key Features
Training Dataset
Whisper was trained on a massive dataset of 680,000 hours of multilingual and multitask supervised data collected from the internet. This includes a diverse range of audio types such as conversational speech, news broadcasts, podcasts, and more. About 117,000 hours of this data are multilingual, enabling support for 99 languages.Architecture
Whisper uses an end-to-end deep learning model based on an encoder-decoder Transformer architecture. This architecture allows the model to process audio input in 30-second chunks, converting them into log-Mel spectrograms and generating accurate text transcriptions. The model can also handle tasks like language identification, phrase-level timestamps, and speech translation.Multilingual Support
One of Whisper’s standout features is its ability to recognize and transcribe speech in multiple languages, including many low-resource languages. This makes it highly versatile for global applications.Customizability
Whisper can be fine-tuned for specific tasks and domains, such as recognizing industry-specific jargon, handling new languages or dialects, and improving performance in particular environments. This adaptability makes it suitable for a wide range of industries, including healthcare, media and entertainment, customer service, and education.Performance Metrics
Whisper boasts a low Word Error Rate (WER), indicating high accuracy in transcription. It performs well across various benchmarks, such as the Common Voice and LibriSpeech datasets, especially in noisy conditions.Practical Applications
Whisper’s applications are diverse, including transcribing meetings, converting educational materials into text, enabling voice assistants, and providing automatic captioning. It can streamline operations by automating transcription tasks and improve customer experiences through more accurate voice-controlled systems. In summary, OpenAI Whisper is a powerful ASR system that offers exceptional accuracy, multilingual support, and adaptability, making it a valuable tool for various applications across different industries.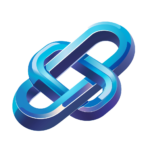
Whisper (OpenAI) - User Interface and Experience
User Interface and Experience of OpenAI Whisper
The user interface of OpenAI’s Whisper, while highly functional, is not typically user-friendly for non-technical users. Here are some key points to consider:Installation and Setup
Whisper is an open-source project, and its files are hosted on GitHub. To use Whisper, users need to download the necessary files and run some code to install it on their system. This process can be technical and may require some developer tools, which can be a barrier for those without programming experience.Usage
Once installed, using Whisper involves running commands in a terminal or command prompt. For example, to transcribe an audio file, users need to type a command followed by the file name. If the file name has spaces, it must be enclosed in apostrophes. This command-line interface can be intimidating for users who are not familiar with terminal commands.Transcription Process
The transcription process itself is relatively straightforward once the initial setup is complete. Whisper splits the input audio into 30-second chunks, converts them into log-Mel spectrograms, and then processes them through its encoder-decoder architecture to generate text output. However, users need to be aware of the dependencies on their system’s GPU or CPU speed, as this affects the transcription time.Features and Accuracy
Despite the technical setup, Whisper offers exceptional accuracy and features. It can transcribe speech in 99 languages and translate them into English, with high accuracy in many languages, especially Spanish, Italian, English, and Portuguese. Whisper also handles noisy environments and overlapping voices effectively, making it versatile for various use cases such as meetings, lectures, and video editing.User Experience
The overall user experience is heavily dependent on the user’s technical proficiency. For developers and those comfortable with command-line interfaces, Whisper can be a powerful tool that integrates seamlessly into their workflows. However, for non-technical users, the lack of a user-friendly interface and the need to navigate through developer notes can be a significant hurdle.Real-World Applications
Despite these challenges, Whisper’s capabilities make it highly useful in various real-world scenarios. It can be used by students to transcribe class notes, by meeting organizers to derive context from recorded meetings, and by podcasters and video editors to repurpose audio content. Its integration with other tools, such as the ChatGPT app, also enhances its usability in everyday tasks.Ease of Use
The ease of use for Whisper is generally low for non-technical users due to the technical setup and command-line interface. However, for those with some programming knowledge, the process can be manageable, and the benefits of using Whisper can outweigh the initial difficulties.Engagement and Factual Accuracy
Whisper’s engagement is high in terms of its accuracy and the quality of the transcriptions it produces. The model’s ability to handle diverse languages, accents, and noisy environments makes it a reliable choice for those who need accurate speech-to-text transcription. However, the need for technical expertise to set it up and use it effectively can limit its broader adoption. In summary, while Whisper offers exceptional transcription accuracy and versatility, its user interface and experience are more suited to technically inclined users. For a more user-friendly experience, users might need to rely on third-party applications or services that integrate Whisper’s capabilities into a more accessible interface.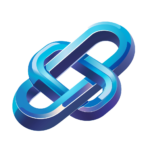
Whisper (OpenAI) - Key Features and Functionality
OpenAI’s Whisper Model
OpenAI’s Whisper model is a sophisticated AI system primarily focused on automatic speech recognition (ASR), which involves transcribing spoken language into text. Here are the main features and functionalities of Whisper:
Automatic Speech Recognition (ASR)
Whisper is trained on a massive dataset of 680,000 hours of multilingual, supervised data from the internet. This extensive training enables it to handle a wide variety of accents, vocabularies, and topics with high accuracy.
Multilingual Support
Whisper supports transcription and translation of speech from multiple languages into English. Approximately 117,000 hours of its training data are multilingual, allowing it to transcribe speech in 99 languages, many of which are considered low-resource languages.
Encoder-Decoder Architecture
Whisper uses an encoder-decoder Transformer architecture. The input audio is split into 30-second chunks, converted into a log-Mel spectrogram, and passed through an encoder to generate a mathematical representation. This representation is then decoded using a language model to predict the most likely sequence of text tokens.
Contextual Transcription
The Transformer architecture allows Whisper to keep track of long-range dependencies in speech, enabling it to contextualize words and improve transcription accuracy. This means it can “remember” what was said previously to fill in gaps and ensure coherent transcripts.
Additional Features
- Language Identification and Translation: Whisper can identify the language of the input speech and translate it into English text.
- Phrase-Level Timestamps: It can provide timestamps for specific phrases or segments within the transcribed text.
- Speaker Diarization: Although not supported in all integrations (e.g., Azure OpenAI Service), Whisper can be configured to distinguish between different speakers in a conversation, especially when used through services like Azure AI Speech.
Applications
- Transcribing Meetings and Calls: It can automate transcription tasks for meetings, customer service calls, and other business communications.
- Educational Materials: Converting educational audio content into text enhances accessibility and learning.
- Voice Assistants: Whisper can improve the accuracy of voice assistants and voice-controlled systems, enhancing user engagement and satisfaction.
- Automatic Captioning: It can generate captions for videos and live streams, making multimedia content more accessible.
Integration and Usage
Whisper can be integrated into various platforms, such as XCALLY for contact centers, to automate complex processes and improve customer interaction experiences. It is also available through Azure AI services, offering different features depending on whether it is used via Azure OpenAI or Azure AI Speech.
Benefits
- Enhanced Accessibility: Whisper improves communication and accessibility by converting spoken language into text, making it easier for people to engage with audio content.
- Operational Efficiency: It streamlines operations by automating transcription tasks, saving time and resources.
- Improved Customer Experience: Whisper enables more accurate and personalized responses in real-time, enhancing customer engagement and satisfaction.
Overall, Whisper represents a significant advancement in ASR technology, leveraging massive datasets and advanced machine learning to provide highly accurate and versatile speech transcription capabilities.
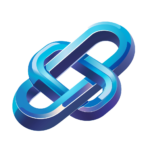
Whisper (OpenAI) - Performance and Accuracy
Performance Evaluation of OpenAI’s Whisper
When evaluating the performance and accuracy of OpenAI’s Whisper in the audio tools AI-driven product category, several key points and limitations emerge:Accuracy Metrics
Whisper’s performance is often measured using the Word Error Rate (WER), which indicates the percentage of words misrecognized in a transcription. According to various benchmarks:- Whisper’s WER ranges from around 7.75% to 10.13% depending on the dataset and model variant.
- For example, in a comparison with Universal-2, Whisper large-v3 and Whisper turbo had WERs of 7.88% and 7.75%, respectively, which is slightly higher than Universal-2’s 6.68% WER.
- In another benchmark, Soniox outperformed Whisper with an average WER of 6.82% compared to Whisper’s 10.13%, highlighting a significant gap in accuracy across different datasets.
Alphanumeric Recognition
Whisper performs well in recognizing alphanumerics, which is crucial for transcribing phone numbers, ticket numbers, and other such sequences. However, it is not the best in this category. For instance, Whisper large-v3 had an Alphanumeric WER of 3.84%, which is slightly better than Universal-2’s 4.00% but still indicates room for improvement.Hallucinations and Errors
One of the significant limitations of Whisper is its tendency to generate hallucinations – recognizing or inserting words that were not spoken in the audio. This issue is particularly problematic in high-stakes applications such as clinical documentation, where it can lead to erroneous patient diagnoses or poor medical decision-making.- Whisper sometimes recognizes extra words or fails to recognize clearly spoken words, leading to high insertion and deletion error rates. This is evident in datasets like news reporting and telephony, where Whisper’s errors were more pronounced.
Performance on Disfluent Speech
Whisper’s performance drops significantly when dealing with disfluent speech, such as stuttered speech. A study using the SEP-28k dataset showed a 20% drop in Word Error Rate for stuttered speech compared to fluent speech, indicating that Whisper struggles with shorter audio clips and disfluent speech patterns.Practical Considerations
OpenAI itself warns against using Whisper in “high-risk domains” or “decision-making contexts” due to its accuracy flaws. This caution is crucial for users considering integrating Whisper into critical systems where accuracy is paramount.Conclusion
In summary, while Whisper is a capable speech recognition model, it has notable limitations, particularly in handling disfluent speech, avoiding hallucinations, and maintaining high accuracy across various datasets. These issues highlight areas where improvements are necessary to enhance its reliability and accuracy.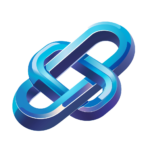
Whisper (OpenAI) - Pricing and Plans
Pricing Structure for OpenAI’s Whisper Audio Transcription Service
The pricing structure for OpenAI’s Whisper audio transcription service is relatively straightforward, though it is primarily centered around API usage. Here are the key points:
API Pricing
- The Whisper API is charged based on the duration of the audio transcribed. As of the latest updates, the cost is $0.006 per transcribed minute.
File Size and Format Limitations
- The Whisper API has a file size limit of 25 MB. Supported file formats include m4a, mp3, webm, mp4, mpga, wav, and mpeg. Files cannot be sent as links; they must be uploaded directly.
No Free Tier in Production
- Since March 1, 2023, the Whisper API is no longer free in the playground or for production use. Users must pay for the transcription services based on the per-minute rate.
No Tiered Plans
- Unlike other OpenAI products like ChatGPT, Whisper does not have tiered plans (e.g., Plus, Pro, Team, Enterprise). The pricing is uniform for all users based on the per-minute transcription rate.
Usage and Billing
- The cost is calculated based on the actual transcription time, and users are billed separately for API usage. This is distinct from any subscription plans for other OpenAI services like ChatGPT.
In summary, the Whisper API from OpenAI is priced at $0.006 per transcribed minute, with specific file size and format limitations, and there are no free or tiered plans available for this service.
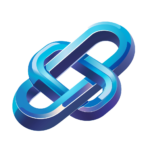
Whisper (OpenAI) - Integration and Compatibility
Whisper Overview
Whisper, the AI-driven speech-to-text model developed by OpenAI, integrates with various tools and platforms in several ways, ensuring broad compatibility and utility.
API Integration
Whisper is accessible through OpenAI’s Audio API, which allows developers to integrate the speech-to-text capabilities into their applications. This API supports both transcription and translation of audio files and can handle a wide range of languages, with support for 98 languages, although the quality varies based on the word error rate (WER).
Platform Compatibility
Intuiface
Whisper can be integrated into Intuiface through the OpenAI Audio API, allowing users to leverage Whisper’s speech-to-text functionality within their interface assets. This integration is made user-friendly by hiding the underlying API complexity, presenting users with simple properties, triggers, and actions.
Android and iOS
While Whisper is primarily known for its integration in AI-powered hearing aids, the Whisper Hearing System, which uses Whisper’s AI, has expanded its support to include several Android devices, in addition to its existing support for iOS devices. This system includes a small AI device (Whisper Brain) and earpieces, and it receives regular software updates to improve performance.
Developer Tools
Offline Voice Typing
There is a community interest in integrating Whisper into keyboards like SwiftKey for offline voice typing and dictation. While this is not yet a standard feature, it highlights the potential for Whisper to be integrated into various keyboard applications to enhance user experience.
File Handling and Technical Details
Audio File Handling
The Whisper API supports audio files up to 25 MB. For larger files, developers need to break them into chunks or use compressed audio formats to avoid losing context mid-sentence.
Home Automation
Home Assistant
Although this is not directly related to the OpenAI Whisper model, there is a different integration named “Whisper” in Home Assistant, which uses the Wyoming Protocol. This integration allows for the control of certain devices that share a common communication protocol, but it is not related to the OpenAI Whisper AI model.
Conclusion
In summary, Whisper’s integration is primarily through API access, making it versatile for various applications, including speech-to-text transcription, translation, and even innovative medical devices like AI-powered hearing aids. Its compatibility spans multiple platforms, including Android and iOS, and it has the potential for further integration into developer tools and other software applications.
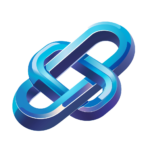
Whisper (OpenAI) - Customer Support and Resources
Customer Support
If you need support for Whisper or any other OpenAI services, there are a couple of ways to get in touch with the support team:
- If you have an account with OpenAI, you can log in and use the “Help” button to start a conversation with the support team.
- If you don’t have an account or can’t log in, you can reach out by selecting the chat bubble icon in the bottom right of the help.openai.com page.
Additional Resources
Documentation and Tutorials
OpenAI provides various resources to help you get started with Whisper:
- There are detailed tutorials and guides available on how to use the Whisper API, such as converting podcasts to text, creating speech-to-text applications with Flask, and running the Whisper speech recognition model.
- The DataCamp tutorial offers a comprehensive guide on using the Whisper API for speech-to-text conversion, including information on supported file formats and integration with Python.
Community and GitHub Projects
The community around Whisper is active, with numerous projects and resources available on GitHub:
- You can find a curated list of awesome OpenAI Whisper projects, including various model variants, applications, and tutorials. This includes projects like live-streaming transcription, speaker diarization, and automatic YouTube subtitle generation.
- There are also videos and tutorials available that demonstrate how to use Whisper for different tasks, such as multilingual speech recognition and speech translation.
Technical Details and Capabilities
For those interested in the technical aspects of Whisper:
- Whisper is an end-to-end deep learning model based on an encoder-decoder Transformer architecture. It can transcribe speech in multiple languages and translate speech to English. The model is trained on a vast dataset of 680,000 hours of supervised data.
- The model supports various audio formats like `mp3`, `mp4`, `mpeg`, `mpga`, `m4a`, `wav`, and `webm`, with a file size limit of 25MB.
Integration and Deployment
For integrating Whisper into your applications or deploying it on cloud services:
- Azure provides a quickstart guide on how to use the Azure OpenAI Whisper model for speech-to-text conversion. This includes setting up environment variables, retrieving API keys, and making REST API requests.
These resources should help you get started with using Whisper effectively and address any questions or issues you might have.
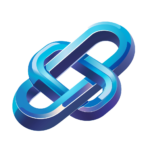
Whisper (OpenAI) - Pros and Cons
Advantages of OpenAI Whisper
Accuracy and Versatility
- OpenAI Whisper is renowned for its exceptional accuracy in transcribing spoken language into written text. It boasts a word error rate of 8.06%, making it 92% accurate by default.
- Whisper can handle a wide range of languages, with support for 99 languages, many of which are considered low-resource. This makes it highly versatile for multilingual applications.
Performance in Challenging Conditions
- Whisper performs well in challenging acoustic conditions such as noisy audio and heavily accented speech, although its performance can be affected by these factors.
Customization and Flexibility
- Being an open-source model, Whisper offers significant flexibility and customizability. Developers can fine-tune it for specific tasks, recognize industry-specific jargon, and adapt it to new languages, dialects, and accents.
Efficiency and Automation
- Whisper can significantly reduce manual labor by automating transcription tasks, making it useful for applications like transcribing interviews, podcasts, and live-streams.
Accessibility
- Whisper enhances accessibility by converting spoken language into written text, which is particularly beneficial for individuals who are hard-of-hearing.
Community and Transparency
- OpenAI provides clear documentation and actively engages with the community to improve and address issues with the model. This transparency is a significant advantage over some proprietary alternatives.
Disadvantages of OpenAI Whisper
Hallucinations and Fabrications
- One of the major flaws of Whisper is its tendency to “hallucinate” or invent text that was not spoken. This can include harmful content such as racial commentary or violent rhetoric, and it occurs at a rate of about 1-2% depending on the speech type.
Resource Intensity
- Whisper is resource-intensive and requires significant computational power, which can be a challenge for users with limited hardware capabilities. This can lead to slower processing times if not adequately resourced.
Audio Quality Dependence
- The accuracy of Whisper’s transcriptions can be affected by the quality of the audio input. Background noise, poor audio quality, or heavily accented speech can lead to less accurate transcriptions.
Limitations in Specific Domains
- While Whisper is highly accurate in general, it may require fine-tuning to perform optimally in specific professional or business environments. It is not designed as a production-ready enterprise tool and can face practical issues with large volumes of transcription.
File Size Limitations
- Whisper has a file size limit of 25 MB for audio inputs, which can be restrictive for longer recordings or larger files.
Need for Fine-Tuning
- To achieve optimal results, Whisper often needs to be fine-tuned for specific tasks or domains. Without this fine-tuning, the model may produce mediocre results and make mistakes during transcription.
By considering these points, users can better evaluate whether OpenAI Whisper meets their specific needs and how to optimize its use.
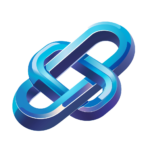
Whisper (OpenAI) - Comparison with Competitors
Unique Features of Whisper
Multilingual Support
High Accuracy
Adaptability
Transformer Architecture
Comparison with Google’s Chirp
Accuracy and Punctuation
Cost
Other Alternatives
Microsoft Azure Speech Services
Google Cloud Speech-to-Text
Practical Considerations
Deployment Requirements
Customization and Industry Use
Conclusion
In summary, Whisper stands out due to its high accuracy, extensive multilingual support, and adaptability, making it a strong contender in the AI-driven audio tools category. However, other services like Google’s Chirp and Microsoft Azure Speech Services offer competitive features and pricing, and the choice ultimately depends on the specific needs and use cases of the user.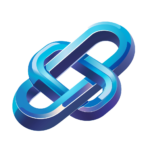
Whisper (OpenAI) - Frequently Asked Questions
What is OpenAI Whisper?
OpenAI Whisper is an Automatic Speech Recognition (ASR) system that transcribes spoken language into written text using deep learning techniques. It was released in September 2022 and is known for its high accuracy and versatility in handling diverse languages and acoustic conditions.
How does Whisper work?
Whisper operates using an encoder-decoder Transformer architecture. The process involves splitting the input audio into 30-second chunks, converting them into log-Mel spectrograms, and then passing these through an encoder to generate a mathematical representation. This representation is then decoded using a language model to predict the most likely sequence of text tokens.
What are the key features of Whisper?
Whisper can transcribe speech into text, translate speech from various languages to English, and perform tasks like language identification, phrase-level timestamps, and multilingual speech transcription. It can also be fine-tuned for specific domains, such as recognizing industry-specific jargon and handling new languages, dialects, and accents.
How accurate is Whisper?
Whisper has an average word error rate (WER) of 8.06%, meaning it is approximately 92% accurate by default. Its accuracy is superior to many other open-source ASR models, especially in handling diverse languages and noisy audio conditions.
What are the different sizes of Whisper models available?
Whisper models come in various sizes, ranging from 39 million to 1.55 billion parameters. Larger models offer better accuracy but at the cost of longer processing times and higher computational costs. Smaller models can be optimized for speed.
How is Whisper trained?
Whisper is trained on a vast dataset of 680,000 hours of supervised data, with 117,000 hours being multilingual. This extensive training data allows Whisper to generalize well and perform effectively across various applications.
Can Whisper be used for real-time transcription?
While Whisper can be used for live-streaming transcription, it is not inherently designed as a real-time tool. For real-time applications, additional optimizations and infrastructure may be necessary. It is more suited for product demos, academic projects, and indie projects with relatively low volumes of audio.
How does Whisper compare to other ASR models like Azure Cognitive Services Speech Services?
Whisper is optimized for transcribing audio files, especially in English, and is recommended for fast processing of individual audio files. In contrast, Azure Cognitive Services Speech Services support over 100 languages and are easier to use for tasks like speech-to-text, text-to-speech, and speaker recognition. Azure services are better for batch processing and more comprehensive speech-related tasks.
What are the pricing details for using Whisper?
When using Whisper through Azure services, the pricing is $0.36 per hour, with discounts available for larger volumes (20% for 2000 hours, 35% for 10,000 hours, and 50% for 50,000 hours).
Can Whisper be fine-tuned for specific tasks?
Yes, Whisper can be fine-tuned to recognize new languages, dialects, and accents, as well as to be more sensitive to specific domains. This allows developers to adapt the model to their particular use cases.
How can prompts be used with Whisper?
Prompts can be used to help Whisper maintain context and consistency across multiple audio segments. You can submit prior segment transcripts or use fictitious prompts to steer the model towards specific styles or spellings. However, prompts are limited to 224 tokens, and any tokens beyond this limit are ignored.
Is Whisper suitable for large-scale enterprise use?
Whisper is not designed as a production-ready enterprise tool and can be challenging to run at scale. For professional projects requiring over 100 hours of recurrent transcription per month, practical issues may arise, such as insufficient speed or accuracy.
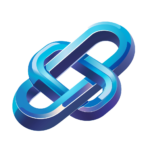
Whisper (OpenAI) - Conclusion and Recommendation
Final Assessment of OpenAI Whisper
OpenAI Whisper is a revolutionary speech recognition system that stands out in the audio tools AI-driven product category due to its advanced capabilities and versatility.Key Strengths
- Multilingual Support: Whisper supports transcription in up to 99 languages, including many low-resource languages, making it a powerful tool for breaking down language barriers.
- Accuracy and Real-Time Transcription: Whisper’s transformer-based architecture and extensive training on 680,000 hours of multilingual data enable it to transcribe speech with high accuracy, even in noisy environments and with various accents.
- Translation Capabilities: It can translate speech from multiple languages into English, enhancing its utility in global communication.
- Customizability: Whisper can be fine-tuned for specific domains, languages, and accents, making it adaptable to various industries and applications.
Applications and Benefits
- Accessibility: Whisper significantly improves accessibility for hearing-impaired individuals by providing real-time transcriptions, and it aids non-native speakers by offering multilingual content in their preferred language.
- Healthcare: It accurately transcribes medical dictations and patient interactions, reducing administrative workload and improving documentation accuracy.
- Customer Service: Whisper enhances call center operations with real-time transcription of multilingual customer interactions, improving response times.
- Education: It assists in language learning and accessibility by providing accurate transcriptions and translations of lectures or course materials.
- Media and Entertainment: Whisper generates multilingual subtitles for videos and podcasts, enabling content accessibility across different languages.
Who Would Benefit Most
- Researchers: The primary target audience for Whisper is AI researchers studying speech recognition, robustness, and generalization. Researchers can leverage Whisper to advance their studies and develop practical applications.
- Businesses and Organizations: Companies in healthcare, customer service, education, and media can benefit from Whisper’s accurate and real-time transcription capabilities, improving efficiency and accessibility.
- Individuals with Hearing Impairments: Whisper’s real-time transcription feature makes it an invaluable tool for hearing-impaired individuals, enabling them to follow conversations and content more easily.
Overall Recommendation
OpenAI Whisper is highly recommended for anyone seeking a reliable and accurate speech recognition system, especially those needing multilingual support and real-time transcription. Its adaptability and customizability make it suitable for a wide range of applications across various industries.For users deciding between using Whisper via Azure OpenAI or Azure AI Speech, the choice depends on the specific needs:
- Use Whisper via Azure OpenAI for quick transcription of individual audio files and translation from other languages into English.
- Use Whisper via Azure AI Speech for batch processing of large files, diarization, and word-level timestamps.