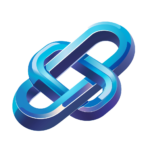
BioGPT - Detailed Review
Content Tools
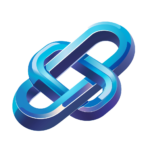
BioGPT - Product Overview
Introduction to BioGPT
BioGPT is a domain-specific generative pre-trained Transformer language model developed primarily for biomedical applications. Here’s a breakdown of its primary function, target audience, and key features:Primary Function
BioGPT is trained on a vast dataset of biomedical research articles, specifically 15 million PubMed abstracts. This training enables the model to generate and mine biomedical text effectively. It is particularly useful for tasks such as text generation, question answering, document classification, and data mining within the biomedical domain.Target Audience
The primary target audience for BioGPT includes researchers, scientists, and professionals in the biomedical field. This includes those involved in drug discovery, genetic research, precision medicine, bioengineering, and environmental monitoring. BioGPT is also beneficial for clinicians and medical professionals who need to analyze and generate biomedical text.Key Features
Training Data
BioGPT is pre-trained on a large-scale dataset of biomedical literature, including PubMed abstracts. This extensive training data allows the model to generate highly accurate and detailed descriptions of biological processes and structures.Performance
BioGPT performs at the level of human experts in various biomedical tasks and outperforms other general and scientific language models. It achieves high F1 scores in tasks such as end-to-end relation extraction and document classification.Applications
The model is effective in drug discovery, genetic research, precision medicine, bioengineering, and environmental monitoring. It can aid in identifying therapeutic targets, developing medical knowledge graphs, and assisting in medical dialogue systems.Technical Capabilities
BioGPT uses a causal language modeling objective, making it powerful at predicting the next token in a sequence and generating syntactically coherent text. It can also leverage past key-value attention pairs to optimize text generation. In summary, BioGPT is a specialized AI tool that leverages large-scale biomedical data to support advanced research and applications in the biomedical field, making it an invaluable resource for researchers and medical professionals.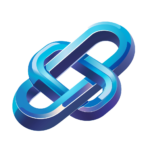
BioGPT - User Interface and Experience
User Interface of BioGPT
The user interface of BioGPT, a specialized generative Transformer language model developed by Microsoft, is designed to be intuitive and user-friendly, particularly for developers, data scientists, and researchers in the biomedical field.
Interface Design
BioGPT’s interface is characterized by its simplicity and ease of use. It is implemented using PyTorch and the Transformers library, which makes it accessible for those familiar with these tools.
- The model supports various decoding strategies, including beam-search decoding, which can be easily managed through the interface.
- It is designed to be integrated into existing NLP pipelines, making it straightforward for users to incorporate BioGPT into their workflows.
Ease of Use
The interface is user-friendly, allowing users to quickly and accurately generate natural language text related to biomedical literature.
- BioGPT can perform a wide range of tasks such as answering questions, extracting relevant data, and generating text, all of which can be initiated through a straightforward and intuitive process.
- The model’s ability to generate fluent descriptions for biomedical terms and assist in biomedical research and literature analysis is facilitated by an interface that does not require extensive technical knowledge beyond what is typical for NLP tasks.
Overall User Experience
The overall user experience is enhanced by BioGPT’s efficiency and speed. Here are some key points:
- BioGPT can generate high-quality text at an incredible speed, which is beneficial for tasks that require rapid data analysis and text generation.
- The model achieves high accuracy on various biomedical natural language processing tasks, such as PubMedQA and BC5CDR, which adds to the positive user experience by delivering reliable results.
- The ability to automate text summarization, extraction, and generation tasks reduces the time and resources needed for data analysis, allowing researchers to focus on more strategic areas of their work.
In summary, BioGPT’s user interface is designed to be easy to use, efficient, and highly effective for generating and processing biomedical text, making it an invaluable tool for researchers and professionals in the biomedical field.
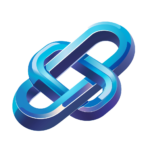
BioGPT - Key Features and Functionality
BioGPT Overview
BioGPT, developed by Microsoft, is a sophisticated language model specifically designed for the biomedical domain, boasting several key features and functionalities that make it a valuable tool in biomedical research and applications.Training Data and Architecture
BioGPT is trained on a vast dataset of 15 million biomedical articles from PubMed, which includes abstracts and titles of articles published in English before 2021. This model uses a transformer architecture, similar to other popular models like BERT and GPT, but with a focus on generation rather than classification or regression. It was pre-trained using eight Nvidia V100 GPUs for 200,000 steps and fine-tuned with a single Nvidia V100 GPU for 32 steps, resulting in a model with 357 million parameters.Text Generation
One of the primary features of BioGPT is its ability to generate highly accurate and detailed descriptions of biological processes and structures. This capability is particularly useful in biotechnology research, where researchers need to create content quickly and accurately. BioGPT can generate fluent descriptions for biomedical terms, making it an ideal tool for researchers and scientists.Biomedical Natural Language Processing
BioGPT has been trained on a wide range of biomedical tasks, including relation extraction, question answering, and document classification. It outperforms previous models on most biomedical tasks, such as achieving a 44.98% F1 score on BC5CDR and 78.2% accuracy on PubMedQA, a benchmark for biomedical question answering.Real-World Applications
BioGPT has several real-world applications:Drug Discovery
It can help in identifying potential drug targets and developing more effective treatments by analyzing large amounts of biomedical research.Genetic Research
BioGPT aids in generating descriptions of specific therapeutic classes or therapies.Precision Medicine
It can assist in personalized treatment plans by analyzing patient-specific data.Bioengineering and Environmental Monitoring
BioGPT’s capabilities extend to these fields by generating detailed descriptions and aiding in research and development.Efficiency and Customization
BioGPT is efficient and supports various decoding methods, including beam-search decoding, which allows for more control over the output. It can be easily integrated into existing pipelines and is implemented using PyTorch and the Transformers library, making it versatile for different tasks.Knowledge Extraction and Summarization
BioGPT can extract relevant data from medical literature and generate summaries, which is beneficial for researchers who need to quickly analyze large volumes of biomedical texts. It can also answer questions based on PubMed articles with high accuracy.Conclusion
In summary, BioGPT’s integration of AI in the biomedical domain enhances research capabilities through its text generation, biomedical natural language processing, and knowledge extraction features, making it a valuable tool for researchers and professionals in the field.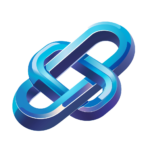
BioGPT - Performance and Accuracy
Performance Highlights
BioGPT has demonstrated impressive performance in various biomedical natural language processing (NLP) tasks. Here are some notable achievements:- BioGPT outperforms previous models on most biomedical NLP tasks, such as BC5CDR, KD-DTI, and DDI end-to-end relation extraction, with significant F1 scores.
- It achieves high accuracy on PubMedQA, reaching up to 81.0% with the larger BioGPT-Large model.
- In lay summarization tasks, fine-tuned BioGPT models show better performance than GPT-2 models, particularly in relevance, readability, and factuality metrics.
Accuracy and Limitations
Despite its strong performance, BioGPT faces several accuracy and reliability issues:- Inaccurate or Misleading Text: BioGPT can generate inaccurate or misleading text, which is a significant concern. It may produce nonsensical answers, pseudoscientific claims, and even fabricate citations and studies to support its claims.
- Hallucinations: Similar to other advanced AI models, BioGPT can “hallucinate” false information, which could be dangerous if relied upon by patients or medical professionals.
- Zero-Shot Performance: The zero-shot performance of BioGPT models is inferior to that of GPT-2 models, possibly due to the smaller dataset used for pre-training.
- Overreliance and Disclosure: There is a concern about users overrelying on AI tools like BioGPT without fully understanding their limitations. Proper disclosure of AI use and prompts is essential to maintain integrity in medical publishing.
Areas for Improvement
To improve BioGPT’s accuracy and reliability:- Further Training and Fine-Tuning: Additional research, evaluation, and fine-tuning are necessary for any downstream applications. This could help mitigate the issues of inaccurate or misleading text.
- Regulation and Guidance: The medical publishing industry needs to establish timely advice and regulation to ensure appropriate use of AI tools like BioGPT. This includes guidelines on disclosure and the responsible use of AI-generated content.
- Addressing Biases: Efforts should be made to address and reduce existing biases within the model to ensure the information generated is accurate and unbiased.
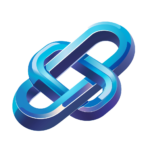
BioGPT - Pricing and Plans
The Pricing Structure of BioGPT
The pricing structure of BioGPT, specifically the AI-driven content tools product, is outlined in several plans, each with distinct features.
Free Plan (Basic)
- This plan is free and includes unlimited bio generation for Instagram, Facebook, Twitter, WhatsApp, LinkedIn, and TikTok.
Standard Plan
- Priced at $15 per month.
- Includes all features from the Basic plan.
- Additional features:
- Username creation: 100 suggestions
- Captions ideas: 100 suggestions
- Bio content: 100 suggestions
- Hashtags ideas: 100 suggestions.
Advance Plan
- Priced at $35 per month.
- Includes all features from the Standard plan.
- Additional features:
- Post suggestions: 250
- Video editing: 250
- New reel creation: 250
- API credits: 5,000.
Additional Features
- The Advance plan also includes other advanced functionalities such as content scheduling, analytics and reporting, social listening, engagement tracking, multi-platform management, automated posting, and more.
Free Trial
- BioGPT also offers a free trial for users to test the features before committing to a paid plan.
This structure allows users to choose a plan that best fits their needs and budget, whether they are individuals, influencers, or agencies.
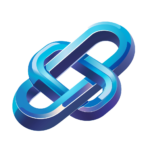
BioGPT - Integration and Compatibility
Integration and Compatibility of BioGPT
When considering the integration and compatibility of BioGPT, which is a domain-specific generative Transformer language model pre-trained on large-scale biomedical literature, several key points come into focus:
Integration with Other Tools
BioGPT is designed to be integrated with various tools and systems, particularly those involved in biomedical research and applications. For instance, it can be used in conjunction with other biomedical language models and tools to enhance tasks such as relation extraction, question answering, and the generation of biomedical content. The model’s ability to generate fluent descriptions for biomedical terms and answer questions about medical conditions makes it a valuable tool for building conversational agents, summarization systems, and other biomedical language processing solutions.
Compatibility Across Platforms and Devices
BioGPT is built using the Transformer architecture and is compatible with popular deep learning frameworks such as PyTorch. This compatibility allows developers to integrate BioGPT into their existing infrastructure with relative ease. Here are some specific points:
- PyTorch Integration: BioGPT can be loaded and used within PyTorch, leveraging its native functions and optimizations, such as the scaled dot-product attention (SDPA) operator.
- Hugging Face: The model is available on the Hugging Face platform, which provides a straightforward way to load, fine-tune, and deploy the model across different environments and devices.
- API and Code Integration: Developers can integrate BioGPT into their code using APIs and example playgrounds provided, allowing for customization and fine-tuning of the model’s behavior.
Data and Infrastructure Compatibility
For effective integration, ensuring access to reliable and relevant data is crucial. BioGPT relies on large-scale biomedical literature, and its performance can be enhanced by fine-tuning it on domain-specific datasets. However, data availability and connectivity can sometimes pose challenges, especially in regions with limited infrastructure.
Conclusion
In summary, BioGPT is designed to be highly integrable with various tools and platforms, particularly those within the biomedical domain. Its compatibility with popular deep learning frameworks and availability on platforms like Hugging Face make it accessible for a wide range of applications and devices.
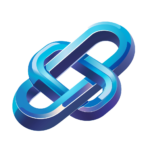
BioGPT - Customer Support and Resources
When looking into the customer support options and additional resources for BioGPT, which is a part of the Hugging Face Transformers library, it’s important to note that BioGPT itself is a model and not a standalone product with dedicated customer support.
However, here are some resources and support avenues that can be helpful:
Hugging Face Community and Documentation
Documentation and Code Examples
The Hugging Face Transformers library, where BioGPT is hosted, provides extensive documentation and code examples. You can find detailed information on how to use BioGPT models, including configuration and implementation details, in the GitHub repository and the Hugging Face documentation.
GitHub Issues and Discussions
Technical Support
For technical issues or questions, you can open an issue or participate in discussions on the Hugging Face Transformers GitHub page. This is a community-driven platform where developers and users can share knowledge and resolve issues.
Hugging Face Forums
Community Support
Hugging Face also has a forum where users can ask questions, share knowledge, and get help from the community.
Model Configuration and Usage
Using BioGPT
The `BioGptConfig` and `BioGptModel` classes are well-documented, allowing you to initialize and use the model with specific configurations. You can find examples of how to do this in the code snippets provided in the GitHub repository.
Since BioGPT is an open-source model and part of a larger library, it does not have a dedicated customer support team like commercial products might. However, the community resources and documentation provided by Hugging Face are comprehensive and can help you get started and resolve most issues.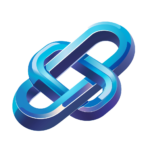
BioGPT - Pros and Cons
Advantages of BioGPT
BioGPT, a biomedical-specific generative AI tool, offers several significant advantages in the healthcare and medical research sectors:Efficiency and Speed
BioGPT can process and analyze vast amounts of medical data much faster than a human, saving healthcare professionals valuable time and improving patient outcomes.Accuracy and Insight
BioGPT is highly accurate in its analysis and recommendations, often identifying patterns and correlations that may not be immediately apparent to humans. It has demonstrated human parity in answering biomedical research questions, particularly in tasks like PubMedQA.Personalized Medicine
BioGPT can help develop personalized treatment plans by analyzing a patient’s medical history and genetic data, identifying potential risk factors, and recommending appropriate interventions.Medical Research
It assists researchers in identifying new drug targets, predicting drug efficacy, and identifying potential side effects of medications. BioGPT also aids in clinical trial patient selection and the development of digital biomarkers.Medical Education
BioGPT can help medical students and professionals by providing real-time recommendations and insights during clinical practice and assisting in understanding complex medical concepts and terminology.Cost-Effectiveness
By reducing the time and resources required for medical analysis, BioGPT can help reduce healthcare costs and increase efficiency.Disadvantages of BioGPT
Despite its numerous benefits, BioGPT also has several limitations and challenges:Data Quality
The accuracy of BioGPT depends on the quality of the data it analyzes. If the data is incomplete or inaccurate, BioGPT may not generate accurate insights.Privacy Concerns
There are concerns about data privacy and security, as with any AI technology. BioGPT must be designed with appropriate safeguards to protect patient data.Inaccurate or Misleading Text
BioGPT, like other generative AI models, can produce inaccurate or misleading text without references, potentially disseminating misinformation. It may also perpetuate biases present in the medical research it was trained on.Regulatory and Guidance Issues
The medical publishing community is calling for more regulation and guidance on the use of BioGPT to ensure its appropriate and ethical use. There is a need for clear guidelines on disclosing AI use within manuscripts and other medical publications.Overreliance
There is a concern about potential user overreliance on AI tools like BioGPT, which could lead to a decrease in critical thinking and human oversight in medical decision-making. By acknowledging both the advantages and disadvantages, healthcare professionals and researchers can better integrate BioGPT into their workflows while addressing its limitations.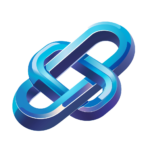
BioGPT - Comparison with Competitors
When comparing BioGPT with other AI-driven content tools in the biomedical domain, several key aspects stand out:
Unique Features of BioGPT
- Generative Capabilities: BioGPT is distinct for its ability to generate text, a feature that sets it apart from other models like BioBERT and PubMedBERT, which are primarily discriminative. This makes BioGPT ideal for tasks such as generating fluent descriptions for biomedical terms, biomedical text generation, and answering medical questions.
- Pre-training on Biomedical Literature: BioGPT is pre-trained on a large corpus of biomedical literature, including PubMed abstracts, which enhances its performance on biomedical natural language processing tasks. It achieves high accuracy on tasks like PubMedQA (78.2% accuracy) and end-to-end relation extraction tasks (e.g., 44.98% F1 score on BC5CDR).
- Efficiency and Speed: BioGPT can generate high-quality text quickly, using advanced decoding methods like beam-search decoding, which allows for more control over the output.
Potential Alternatives
While there are no direct alternatives that match BioGPT’s specific focus on biomedical text generation, here are some AI tools that offer related functionalities, although they may not be as specialized:General Content Generation Tools
- Copy.ai: This tool is designed for generating marketing copy, blog intros, and product descriptions but is not specialized in the biomedical domain. It uses advanced AI language models to generate content quickly but lacks the domain-specific training of BioGPT.
- BlogNLP: This AI writing tool helps with automated content creation, overcoming writer’s block, and improving blogging skills. However, it is not focused on biomedical literature and does not offer the same level of domain-specific performance as BioGPT.
Social Media and Content Management Tools
- PostPaddy: This AI-driven social media and content management software helps generate content ideas, schedule posts, and engage with audiences. While useful for general content creation, it does not have the specialized biomedical capabilities of BioGPT.
- ReContent.AI: This tool repurposes content across different platforms but is not tailored for biomedical text generation or analysis.
Key Differences
- Domain Specificity: BioGPT is uniquely trained on biomedical literature, making it highly effective for biomedical tasks. Other tools, while versatile, lack this domain-specific training.
- Generative vs. Discriminative: BioGPT’s ability to generate text is a significant advantage over models that are primarily discriminative, such as BioBERT and PubMedBERT.
- Performance Metrics: BioGPT outperforms previous models on several biomedical NLP tasks, which is a critical factor for researchers and professionals in the biomedical field.
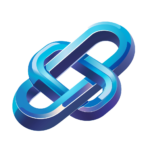
BioGPT - Frequently Asked Questions
Here are some frequently asked questions about BioGPT, along with detailed responses to each:
Q: What is BioGPT?
BioGPT, or Biomedical Generative Pretrained Transformers, is a specialized generative language model developed by Microsoft. It is pre-trained on large-scale biomedical literature, including 15 million PubMed abstracts, to perform various biomedical natural language processing (NLP) tasks.Q: What makes BioGPT unique?
BioGPT stands out for its generative capabilities in the biomedical domain, which is a significant advancement over previous BERT-based models that were primarily discriminative. It can generate coherent and contextually relevant medical text, in addition to performing tasks like relation extraction and question answering.Q: What are the primary tasks that BioGPT can perform?
BioGPT is capable of several key tasks, including:- Text Generation: Generating fluent descriptions for biomedical terms.
- Biomedical Natural Language Processing: Performing tasks such as relation extraction, question answering, and text classification.
- Biomedical Question Answering: Achieving high accuracy on benchmarks like PubMedQA.
- Relation Extraction: Performing end-to-end relation extraction tasks with high F1 scores on datasets like BC5CDR, KD-DTI, and DDI.
Q: How accurate is BioGPT on various biomedical tasks?
BioGPT has demonstrated impressive accuracy on several biomedical NLP tasks:- BC5CDR: 44.98% F1 score.
- KD-DTI: 38.42% F1 score.
- DDI: 40.76% F1 score.
- PubMedQA: 78.2% accuracy.
Q: What is the architecture of BioGPT?
BioGPT uses a transformer architecture, similar to other popular models like BERT and GPT, but with a focus on generation rather than classification or regression. It is implemented using PyTorch and the Transformers library.Q: How fast can BioGPT generate text?
BioGPT can generate high-quality text at an incredible speed. For example, generating 5 different text sequences of up to 20 words each takes only a fraction of a second.Q: What are the recommended use cases for BioGPT?
BioGPT is ideal for:- Biomedical Text Generation: Generating descriptions for biomedical terms.
- Relation Extraction: Extracting relations from medical documents.
- Medical Question Answering: Answering questions based on biomedical literature.
- Knowledge Extraction: Extracting knowledge from medical literature.
Q: How does BioGPT handle factual accuracy and hallucinations?
While BioGPT generates fluent and syntactically correct text, it can sometimes produce hallucinations, especially when generating definitions or relations. These hallucinations can be difficult to spot and may require expert verification to ensure factual accuracy.Q: Can BioGPT be integrated into existing pipelines?
Yes, BioGPT can be easily integrated into existing pipelines. It supports various decoding strategies, including beam search, and is implemented using PyTorch and the Transformers library.Q: What data formats does BioGPT support?
BioGPT accepts input in the form of tokenized text sequences. This means you need to pre-process your text data by breaking it down into individual tokens or words before feeding it into the model.Q: Is BioGPT available for public use?
Yes, BioGPT is available for public use. It is developed by Microsoft and distributed under the MIT license, with over 56,509 downloads recorded.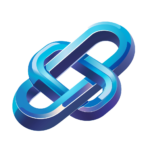
BioGPT - Conclusion and Recommendation
Final Assessment of BioGPT
BioGPT, developed by Microsoft, is a generative Transformer language model specifically pre-trained on large-scale biomedical literature. Here’s a comprehensive assessment of its capabilities and who would benefit most from using it.Capabilities and Performance
BioGPT stands out for its ability to generate text, a feature that sets it apart from other models like BERT, which are primarily discriminative. It achieves state-of-the-art performance in various biomedical natural language processing (NLP) tasks, including relation extraction, question answering, and text classification. For instance, it achieves a 44.98% F1 score on BC5CDR and 78.2% accuracy on PubMedQA.Primary Use Cases
BioGPT is ideal for several key applications:- Biomedical Text Generation: It can generate fluent descriptions for biomedical terms, which is beneficial for researchers and scientists needing to create content quickly.
- Biomedical Research: It assists in identifying potential new drug targets, predicting drug efficacy, and identifying potential side effects of medications.
- Medical Literature Analysis: It helps in analyzing and summarizing large amounts of medical literature, including electronic health records (EHRs), clinical trial reports, and other medical texts.
- Medical Education: It can aid medical students and professionals in understanding complex medical concepts and terminology.
Benefits
- Time-Saving: BioGPT can process and analyze vast amounts of medical data much faster than humans, saving valuable time for healthcare professionals.
- Increased Accuracy: It is highly accurate in its analysis and recommendations, identifying patterns and correlations that may not be immediately apparent to humans.
- Efficiency: The model supports various decoding strategies, including beam search, making it efficient for generating high-quality text quickly.
Who Would Benefit Most
BioGPT would be highly beneficial for:- Researchers and Scientists: Those working in the biomedical field can leverage BioGPT for generating descriptions, analyzing literature, and identifying new drug targets.
- Healthcare Professionals: Doctors, nurses, and other healthcare workers can use BioGPT to develop personalized treatment plans, predict disease outcomes, and recommend appropriate interventions.
- Medical Writers: BioGPT can assist in generating coherent and contextually relevant medical text, summarizing clinical information, and evaluating medical literature.