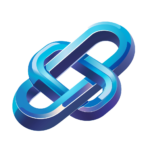
DataRobot Paxata - Detailed Review
Data Tools
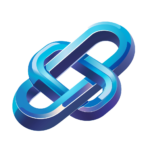
DataRobot Paxata - Product Overview
Introduction to DataRobot Paxata
DataRobot Paxata is an integral component of the DataRobot AI platform, focusing on self-service data preparation and enterprise data fabric solutions. Here’s a breakdown of its primary function, target audience, and key features:Primary Function
DataRobot Paxata is designed to address the critical need for efficient data preparation in AI projects. It automates the data prep process, transforming raw data into ready-to-use datasets for machine learning models. This tool helps bridge the gap between data collection and AI model deployment, making the entire AI lifecycle more streamlined and efficient.Target Audience
The primary users of DataRobot Paxata include business analysts, citizen data scientists, and other stakeholders within organizations who need to prepare data for AI applications. This tool is particularly useful for those without extensive technical backgrounds, as it offers a user-friendly, visual interface for data preparation.Key Features
Visual ETL Interface
Paxata provides a visual Extract, Transform, Load (ETL) interface that allows users to prepare data with little to no code. This interface enables users to select steps for data preparation, including joins, transformations, and formatting changes, all with a few clicks.Data Fabric
Paxata acts as a data fabric, connecting to various data sources and transforming data into a usable format. It leverages algorithmic intelligence and distributed computing, optimized for hybrid and multi-cloud environments.Integration with DataRobot AI Catalog
The data prep tools from Paxata are integrated into DataRobot’s AI Catalog, making it easier for users to prepare data for machine learning. This integration ensures that finalized datasets are ready for model building within the DataRobot platform.Continuous Data Management
Paxata supports the continuous process of updating and retraining AI models as new data sources become available. This is crucial for maintaining the accuracy and relevance of AI models over time.Enterprise Scale and Governance
The platform is built to handle enterprise-scale data integration workloads, ensuring security, governance, and compliance. It stores test results and metadata alongside each AI asset, facilitating confident deployment of models to production. By combining these features, DataRobot Paxata provides a comprehensive solution for data preparation, making it easier for organizations to build, deploy, and maintain AI applications efficiently.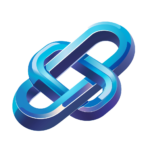
DataRobot Paxata - User Interface and Experience
User Interface of DataRobot Paxata
The user interface of DataRobot Paxata, integrated into the DataRobot AI platform, is characterized by its user-friendly and intuitive design, making it accessible to both technical and non-technical users.Visual ETL Interface
DataRobot Paxata features a visual Extract, Transform, Load (ETL) interface that allows users to prepare data with little to no code. This interface enables users to connect to various data sources, select steps for data preparation, and perform complex joins, transformations, and formatting changes with just a few clicks.Automation and Simplification
The platform automates many data integration processes, simplifying the task of cleaning, transforming, and enriching raw data for analysis. This automation capability reduces the time and effort required for data preparation, making it easier for users to create high-quality datasets ready for machine learning and analytics.Clear Workflow
The visual ETL tool clearly outlines all the steps within the data preparation process. Users can easily track and modify previous steps if needed, ensuring transparency and flexibility in the data preparation workflow.Integration with DataRobot AI Catalog
DataRobot Paxata is seamlessly integrated with the DataRobot AI catalog, allowing users to send finalized datasets directly to the platform for model building. This integration streamlines the process of moving from data preparation to model deployment.Ease of Use
The interface is highly user-friendly, with features that make it easy for users to engage with the platform. The self-service data preparation capabilities empower business users and analysts to prepare data without extensive technical expertise.Additional Features
DataRobot Paxata also supports advanced features such as image recognition and classification through its visual AI capabilities. Users can drag and drop images into a project and build custom deep learning models, combining image data with other feature types.Conclusion
Overall, the user experience of DataRobot Paxata is marked by its simplicity, automation, and clear workflow, making it an effective tool for users to prepare and analyze data efficiently.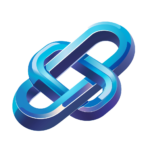
DataRobot Paxata - Key Features and Functionality
The Integration of DataRobot and Paxata
The integration of DataRobot and Paxata brings a comprehensive set of features and functionalities to the DataRobot AI platform, particularly in the area of data preparation and machine learning. Here are the key features and how they work:
Data Preparation
DataRobot Paxata integrates advanced data preparation tools into the DataRobot platform. Here’s how it works:
- Data Ingestion: Paxata can ingest data from a wide variety of sources, including complex semi-structured files like XML or JSON, NoSQL and relational databases, and cloud applications.
- Data Profiling: The platform profiles the data, generating a scorecard that shows data type distribution, field completeness, field length analysis, and other metrics. This helps in identifying trends, outliers, and patterns across the entire dataset.
- Data Cleaning and Transformation: Paxata automatically cleans and transforms raw data, handling tasks such as missing value imputation, standardization, and normalization. It also standardizes similar values and misspellings, and performs joins, appends, and overlaps across different data sources using smart machine learning recommendations.
Integration with AI Catalog
The Paxata platform is integrated with DataRobot’s AI Catalog, which serves as a central hub for all data across the DataRobot platform.
- Data Sharing and Collaboration: Users can share, search, and tag data within the AI Catalog, making it easier for teams to collaborate on machine learning projects.
Automated Feature Engineering
DataRobot, enhanced by Paxata, automates feature engineering to improve the predictive power of models.
- Feature Creation: The platform automatically creates new features from the dataset, including interaction terms, aggregations, and variable transformations. This process helps in identifying the most relevant and powerful predictors for the models.
Model Selection and Training
DataRobot automates the entire machine learning workflow, including model selection and training.
- Model Evaluation: The platform evaluates hundreds of machine learning models in parallel, using techniques like cross-validation to assess their performance. It ranks the models based on metrics such as accuracy, precision, recall, and F1 score, ensuring the best-performing model is selected.
Deep Learning and Visual AI
The integration also includes enhancements in deep learning and visual AI capabilities.
- Deep Learning: DataRobot has introduced a new Keras-based model framework that allows users to build and deploy deep learning models. This includes automated time series forecasting with deep learning techniques to handle large-scale multi-series forecasting applications.
- Visual AI: Users can address computer vision use cases by combining images with other data types. The platform allows for drag-and-drop image uploads and the creation of custom deep learning models for image recognition and classification.
MLOps Enhancements
DataRobot’s MLOps (Machine Learning Operations) capabilities have been enhanced with the integration.
- Model Deployment: Users can deploy model files developed in languages like Python and R via Kubernetes. The platform also offers Monitoring Agents to capture metrics from models deployed in various environments.
- Batch Scoring: DataRobot supports unlimited batch scoring with integrations to leading cloud storage options, making it easier to manage large-scale model deployments.
User-Friendly Interface
The platform is designed to be user-friendly, making advanced analytics accessible to a wide range of users.
- Interactive Interface: Paxata’s interactive, Excel-like interface allows users to search, investigate, and discover trends and patterns in the data. This interface also provides immediate visual feedback, enabling users to validate their data visually.
These features collectively enable DataRobot users to automate many steps in the machine learning lifecycle, from data preparation to model deployment, significantly reducing the time and effort required to build and deploy predictive models.
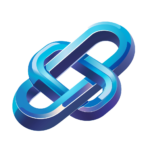
DataRobot Paxata - Performance and Accuracy
Evaluating the Performance and Accuracy of DataRobot with Paxata Integration
Data Preparation and Integration
DataRobot’s acquisition of Paxata has significantly enhanced its data preparation capabilities. Paxata’s self-service data preparation tools help automate the data prep process, which is a critical step in building accurate predictive models. This integration allows users to prepare data for machine learning more efficiently, combining enterprise-scale data integration with AI-enabled data prep. This streamlines the process of getting data ready for modeling, reducing the time spent on data preparation and deployment.Model Accuracy and Performance
DataRobot’s platform is equipped with tools to monitor and improve model accuracy. The Accuracy tab in the DataRobot console allows users to analyze the performance of model deployments over time using standard statistical measures and exportable visualizations. This helps in identifying whether a model’s quality is decaying and if it needs to be replaced. The platform also provides insights based on the problem type and its associated optimization metrics.Feature Engineering and Discovery
DataRobot excels in feature engineering and discovery, which are crucial for model accuracy. The platform can automatically generate new features from the data, and users can build customized recipes for their specific use cases. This feature engineering capability helps in identifying the most relevant features that provide signal to the model, thereby improving its accuracy.External Data Integration
Integrating external data can significantly improve model accuracy. For example, using Ready Signal’s comprehensive Data Catalog with DataRobot’s time series capabilities has shown to improve forecasting accuracy by up to 13% by adding relevant external features such as influenza-like illness rates, rainfall, and foreign exchange rates.Limitations and Areas for Improvement
- Data Processing Limits: The accuracy scores displayed in the Accuracy tab may not reflect every row of larger prediction requests due to data processing limits. These limits can be hourly, daily, or weekly, and there is also a megabyte-per-hour limit. To achieve more precise scores, it is recommended to span requests over multiple hours or days.
- Setup Requirements: The Accuracy tab is not enabled by default and requires specific setup steps, including enabling target monitoring, setting an association ID, and uploading actual values. There must also be a minimum of 100 rows of predictions with corresponding actual values to enable the accuracy analysis.
- Data Quality: While DataRobot can handle “dirty” data and automate much of the data prep, the quality of the input data still plays a critical role in model accuracy. Ensuring high-quality and relevant data is essential for achieving accurate predictive models.
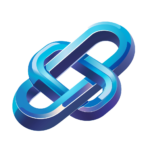
DataRobot Paxata - Pricing and Plans
The Pricing Structure of DataRobot
The pricing structure of DataRobot, which includes integration with Paxata for data preparation, is structured around several key plans and features. Here’s a breakdown of what you can expect:
Pricing Formats
DataRobot offers two main pricing formats:
- Hosted Enterprise Cloud Version: This plan is not based on credits and is billed more directly. It is designed for enterprise users and does not involve the credit system.
- Credit Version: This plan uses a credit system, where users need credits to run various operations within the platform. Credits are allocated based on the project goals specified during the sign-up process.
Free Trial
DataRobot provides a comprehensive free trial that operates on the credit system. Here are some key points about the free trial:
- The free trial requires you to create an account and specify your project goals to receive a certain amount of credits.
- Once the credits are exhausted, the account becomes read-only.
- The free trial has several limitations, including no exports, no support (though community support is available), limited users, and no sharing capabilities.
Enterprise Plan Features
For the Hosted Enterprise Cloud Version, here are some of the features you can expect:
- Automated Machine Learning: DataRobot automates the entire modeling lifecycle, allowing users to build highly accurate predictive models quickly and easily.
- Model Management: Features include model monitoring, deployments, batch prediction jobs, and the ability to host, serve, and monitor custom models.
- Advanced Capabilities: This includes access to the Deployments page, alerts and notifications, service health monitoring, data drift monitoring, and accuracy monitoring. Additional features like Portable Prediction Servers (PPS), governance workflows, automated retraining, and challenger models are also available.
Discounts and Special Plans
DataRobot offers discounts for specific sectors:
- Academic Institutions: Discounts are available for academic institutions to make AI more accessible for educational purposes.
- Government Agencies: Discounts are offered to government agencies to help them make more informed decisions using predictive models and data analytics.
- Nonprofits: Discounts are also available for nonprofit organizations.
Integration with Paxata
While Paxata is not a part of the pricing plan itself, it is often used in conjunction with DataRobot for data preparation. Paxata automates raw data preparation, including finding, ingesting, profiling, cleaning, and transforming raw data. It integrates well with DataRobot’s AI Cloud Manager to provide a seamless data-to-insights workflow.
In summary, DataRobot’s pricing is customized, with a focus on either a credit-based system or a more direct enterprise billing model. The platform offers a free trial with limitations and provides discounts for specific sectors. The features and capabilities are extensive, especially in the enterprise plan, and can be enhanced with integrations like Paxata for data preparation.
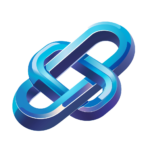
DataRobot Paxata - Integration and Compatibility
The Integration of DataRobot Paxata
The integration of DataRobot Paxata into the DataRobot AI platform is a significant enhancement that streamlines data preparation and machine learning processes. Here’s how it integrates with other tools and its compatibility across different platforms:
Data Preparation and AI Catalog
DataRobot Paxata is integrated with DataRobot’s AI Catalog, allowing users to explore, clean, combine, and shape data for training and deploying machine learning models. This integration enables seamless data preparation directly within the AI platform, reducing the time and effort required to get data machine learning-ready.
Deep Learning and Automated Time Series
The platform includes new deep learning features, such as a Keras-based model framework, which allows users to build and deploy deep learning models efficiently. Automated Time Series forecasting has also been enhanced with deep learning techniques, facilitating large-scale multi-series forecasting applications.
Visual AI and Computer Vision
DataRobot Paxata supports visual AI, enabling users to address computer vision use cases by dragging and dropping images into projects and building custom deep learning models. This feature allows users to combine images with other data types, enhancing image recognition and classification capabilities.
MLOps and Deployment
DataRobot MLOps has been enhanced to include pre-packaged model environments, allowing users to drag-and-drop model files developed in languages like Python and R, and deploy them via Kubernetes. Monitoring Agents can capture metrics from models deployed in almost any environment, and the platform supports unlimited batch scoring with integrations to leading cloud storage options.
Cross-Platform Compatibility
The DataRobot platform, including the Paxata integration, is designed to be compatible with various environments. Users can deploy models via Kubernetes, which is a widely used container orchestration system, ensuring flexibility across different cloud and on-premises infrastructures. The platform also supports model files developed in multiple programming languages, such as Python and R, making it versatile for different user needs.
Integration with Other Tools
DataRobot Paxata integrates well with other tools within the DataRobot ecosystem. For example, the AI Catalog, which was enhanced by the acquisition of Cursor, serves as a central hub for all data across the platform. This integration allows for smooth data flow between data preparation, model building, and deployment stages.
Conclusion
In summary, the DataRobot Paxata integration offers a comprehensive solution for data preparation, machine learning, and model deployment, with strong compatibility across various platforms and tools, making it a cohesive and efficient AI-driven product.
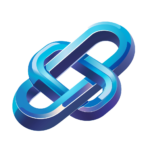
DataRobot Paxata - Customer Support and Resources
Customer Support Options
When using DataRobot’s AI-driven products, including the integrated Paxata data preparation tools, you have several customer support options and additional resources available to ensure you get the help you need.Technical Support
For any technical issues or questions, you can contact DataRobot’s support team via email or visit the dedicated Support site. This resource is handy for resolving problems related to installation, feature usage, or any other technical queries.Documentation and Education
DataRobot provides comprehensive documentation that covers various aspects of their platform. You can find detailed guides on getting started, feature usage, and advanced topics. The documentation includes quickstarts, such as the one for Data Prep, which walks you through importing datasets, working with projects, and performing data operations.Training and Certification
To enhance your skills and knowledge, DataRobot offers training and certification programs through DataRobot University. This resource helps you learn how to effectively use the platform and its tools, including Paxata for data preparation.Community and Additional Resources
DataRobot also provides access to resources like the AI Catalog, which is a collaborative environment where users can share, search, and tag data. This catalog integrates with Paxata, allowing you to pull and push data seamlessly, which is particularly useful for automating data preparation processes.Specific Support Channels
For specific needs, such as support for the Python or R clients, you can visit PyPI or CRAN, respectively, or email the relevant team. This ensures you get targeted help for your particular use case.Conclusion
By leveraging these support options and resources, you can efficiently address any challenges and make the most out of DataRobot’s AI-driven products, including the Paxata data preparation tools.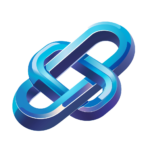
DataRobot Paxata - Pros and Cons
Advantages of DataRobot Paxata
DataRobot Paxata, a data preparation platform integrated into the DataRobot AI suite, offers several significant advantages:
User-Friendly Interface
The platform is known for its intuitive and user-friendly interface, making it accessible for users of various skill levels to clean, transform, and enrich raw data.
Automation Capabilities
It automates data integration and preparation processes, significantly reducing the time and effort required to get data ready for machine learning and analytics.
Data Transformation Features
DataRobot Paxata provides robust data transformation features, enabling users to create high-quality datasets efficiently.
Integration with AI Catalog
The platform is seamlessly integrated with DataRobot’s AI Catalog, allowing users to share, search, and tag data, and to pull and push data from the catalog.
Efficient Data Handling
It effectively handles both structured and unstructured data, providing AI-assisted data examination and accurate reports.
Continuous Platform Enhancement
DataRobot continuously invests in its platform, including the addition of new features like Visual AI and Automated Deep Learning, which enhance the overall user experience and capabilities.
Disadvantages of DataRobot Paxata
Despite its numerous advantages, DataRobot Paxata also has some notable disadvantages:
Limited Advanced Analytics
The platform may have limited advanced analytics functionalities compared to some of its competitors, which can be a drawback for users needing more sophisticated analytical tools.
Integration Issues
There can be difficulties integrating DataRobot Paxata with certain systems or tools, such as Alteryx, which may pose challenges for users with specific integration needs.
Cost
The platform is considered expensive, which can be a significant barrier for companies operating on tight budgets.
Learning Curve
New users may face a steep learning curve, as the software can be challenging to use without prior experience in data science and machine learning.
Limited Customization
Experienced data scientists might find that the platform lacks full flexibility for manual model tweaks, which can limit their ability to customize models according to their specific needs.
Potential for Quantity Over Quality
The automated model creation process might encourage quantity over quality in model creation, which could affect the overall accuracy and reliability of the models generated.
These points highlight the key benefits and drawbacks of using DataRobot Paxata, helping potential users make informed decisions about whether this platform meets their needs.
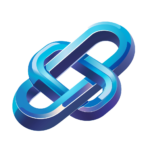
DataRobot Paxata - Comparison with Competitors
When comparing DataRobot with its integrated Paxata capabilities to other AI-driven data tools, several key aspects and alternatives come into focus.
Data Preparation and Automation
DataRobot’s acquisition of Paxata significantly enhances its data preparation capabilities. Paxata offers a self-service data preparation platform that automates tasks such as finding, ingesting, profiling, cleaning, and transforming raw data. This integration allows DataRobot users to automate their data preparation processes, reducing the time and effort required to get data machine learning-ready. In contrast, tools like Alteryx and Trifacta also specialize in data preparation and wrangling, using AI to automate repetitive tasks. Alteryx enables users to perform complex data manipulations without extensive coding knowledge, while Trifacta focuses on cleaning and transforming data efficiently.End-to-End Machine Learning Platform
DataRobot stands out as an end-to-end platform for machine learning, starting with automated data preparation and ending with automated model creation and deployment. This comprehensive approach is unique, as many other platforms may require integration with third-party tools for data preparation. Microsoft Azure Machine Learning, for example, offers a powerful visual drag-and-drop authoring environment but may require separate tools for data preparation. Similarly, Google Cloud Vertex AI provides training and prediction services but might need additional integration for comprehensive data preparation.Integration and Collaboration
The DataRobot AI Catalog, which includes Paxata, serves as a collaborative environment where users can share, search, and tag data. This integration allows for seamless data flow between different stages of the machine learning lifecycle. IBM Cognos Analytics also offers integrated solutions with IBM Watson, supporting natural language queries and automated pattern detection. However, it can be complex and has a steep learning curve, which might not be as user-friendly as DataRobot’s AI Catalog.User Experience and Accessibility
DataRobot’s platform, enhanced by Paxata, is designed to be user-friendly and accessible to a wide range of users. The interactive, Excel-like interface of Paxata makes it easier for users to search, investigate, and discover trends in their data. Tableau, another prominent tool, is known for its advanced visualizations and intuitive drag-and-drop interface. It uses AI to make data analysis more accessible, even for beginners. However, Tableau’s primary focus is on visualization rather than the end-to-end machine learning process.Alternatives and Competitors
For those looking for alternatives, here are some notable competitors:- Microsoft Azure Machine Learning: Offers a visual drag-and-drop environment and strong integration with Microsoft products, but may require additional tools for data preparation.
- Google Cloud Vertex AI: Provides comprehensive machine learning services but can be harder to use and may need separate data preparation tools.
- Databricks Data Intelligence Platform: Built on a lakehouse architecture, it offers an open, unified foundation for data and AI, with strong integration and customization capabilities.
- Alteryx: Specializes in data preparation and blending, making it a good choice for those who need robust data manipulation capabilities without extensive coding.
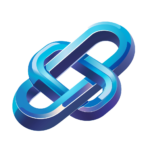
DataRobot Paxata - Frequently Asked Questions
Frequently Asked Questions about DataRobot Paxata
What are the main functions of DataRobot Paxata?
DataRobot Paxata has several key functions. It allows you to import datasets from various sources, explore, clean, combine, and condition the data. Additionally, it enables you to publish prepared data as an AnswerSet, which is a snapshot of your prepped data. Each step you perform in Paxata creates a version, allowing you to continue working on the data without overwriting previous versions.How do I import data into DataRobot Paxata?
To import data into DataRobot Paxata, you can either upload a local dataset or connect to an external data source. You can import data directly into a project or into the Data Prep library. The process involves logging in, selecting the Library or Project option, and then using the ” import” feature to upload your local file or connect to an external data source.What types of data sources can DataRobot Paxata connect to?
DataRobot Paxata can ingest data from a wide variety of sources, including JDBC-enabled data sources, S3, Azure Blob, Google Cloud Storage, URLs, and more. It also supports complex semi-structured files such as XML or JSON, NoSQL and relational databases, and cloud applications.What data cleaning and transformation operations can be performed in DataRobot Paxata?
DataRobot Paxata allows you to perform various data cleaning and transformation operations. These include standardizing values, removing duplicates, finding and fixing errors, pivoting, transposing, grouping by, and more. The software also standardizes similar values and misspellings, and it can join, append, and overlap data across different sources with smart machine learning recommendations.How do I manage and organize my datasets in DataRobot Paxata?
In DataRobot Paxata, you can manage your datasets within the Library and Projects sections. The Library allows you to add new datasets, manage existing ones, and publish AnswerSets. You can also create projects where you can explore and prepare your data. Each dataset in the Library provides options to export, edit details, add new versions, automate, and profile the dataset.What is an AnswerSet in DataRobot Paxata?
An AnswerSet in DataRobot Paxata is a snapshot of your prepped data. When you publish your data as an AnswerSet, it captures the state of your data at that point, allowing you to save and share the prepped data. This feature ensures that you can track and reproduce the steps taken to prepare the data.How does DataRobot Paxata handle data profiling and analysis?
DataRobot Paxata profiles data and generates a scorecard showing data type distribution, field completeness, field length analysis, top/bottom patterns, leading or trailing string patterns, min/max and range, special character analysis, and more. It provides an interactive, Excel-like interface to search, investigate, and discover trends, outliers, and patterns across the entire dataset.Can I automate repetitive tasks in DataRobot Paxata?
Yes, DataRobot Paxata allows you to automate repetitive tasks through workflow automations. You can automate the dataset if the automation feature is enabled, which helps reduce the number of repetitive tasks and ensures consistency in data preparation.How does DataRobot Paxata ensure data governance and auditing?
DataRobot Paxata documents your steps to create repeatability, auditing, and governance. Each step you perform is tracked, and you can always go back to a previous version of the dataset. This ensures transparency and accountability in the data preparation process.What kind of support and resources are available for DataRobot Paxata users?
DataRobot Paxata provides various support resources, including an in-line help menu, a new user guide, and a help shelf with comprehensive documentation. Users can also contact DataRobot’s Customer Success team for feedback and support.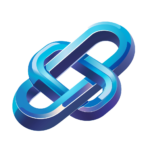
DataRobot Paxata - Conclusion and Recommendation
Final Assessment of DataRobot Paxata
DataRobot Paxata is a powerful tool in the AI-driven data tools category, particularly focused on automated machine learning and comprehensive data preparation. Here’s a detailed look at its benefits and who would most benefit from using it.Key Features
Data Preparation
Paxata, now integrated with DataRobot, offers an enterprise-grade, self-service data preparation application. It can ingest data from various sources, including complex semi-structured files, NoSQL and relational databases, and cloud applications. The software profiles data, generates scorecards, and provides an interactive, Excel-like interface to search, investigate, and discover trends and outliers.
Data Cleaning and Transformation
Paxata includes tools for standardizing values, removing duplicates, finding and fixing errors, and transforming data into a usable format. It also allows users to shape data using pivot, transpose, group by, and other functions.
Automation and Efficiency
DataRobot automates the entire modeling lifecycle, enabling users to quickly build highly accurate predictive models. Paxata’s automated raw data preparation capabilities make it easier for business analysts and citizen data scientists to prep data for machine learning.
Target Users
DataRobot Paxata is most beneficial for several key groups:Large Enterprises
Companies looking to optimize operations and drive innovation through AI and machine learning will find DataRobot Paxata invaluable. It helps streamline processes and enhance decision-making.
Financial Institutions
These organizations can benefit from enhanced risk management and fraud detection through predictive analytics provided by DataRobot Paxata.
Retail Companies
Retailers aiming to personalize customer experiences and improve sales forecasting can leverage the data analytics and machine learning capabilities of DataRobot Paxata.
Healthcare Organizations
Healthcare providers can improve patient outcomes and streamline processes using the data analytics and AI tools offered by DataRobot Paxata.
Data Scientists and Analysts
Data scientists, business analysts, IT professionals, and C-level executives responsible for driving digital transformation will appreciate the ease of data preparation and the automated machine learning features.