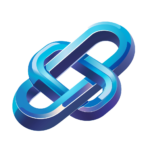
DQLabs - Detailed Review
Data Tools
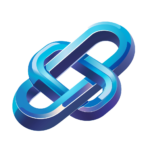
DQLabs - Product Overview
DQLabs Overview
DQLabs is an AI-driven data quality and observability platform that plays a crucial role in ensuring the reliability, accuracy, and consistency of data for various organizational needs.
Primary Function
The primary function of DQLabs is to manage the entire data quality lifecycle. It automates data quality checks, provides deep insights into data health and performance, and facilitates efficient issue resolution and remediation workflows. This helps organizations deliver reliable and accurate data products, leading to better business outcomes.
Target Audience
DQLabs is designed for a diverse audience, including:
- Data Leaders (CDO/CDAOs)
- Business Stakeholders (Data Governance Leaders)
- Data Stewards
- Data Scientists
- Data Analysts
- Citizen Users
- Data Engineers
- DataOps professionals
This broad applicability makes it a versatile tool for various roles within an organization.
Key Features
- Automated Data Quality Checks: DQLabs offers over 250 out-of-the-box automated rules for data quality, covering aspects such as volume, schema, freshness, data reliability, distribution, frequency, statistics, and pattern matching. Users can also define custom rules to suit specific needs.
- AI/ML Driven Anomaly Detection: The platform uses AI-augmented models for deep profiling, anomaly detection, and real-time data analysis. This includes displaying distribution across value frequency or range frequency and performing pattern analysis.
- Data Observability and Discovery: DQLabs integrates data observability, measurement, discovery, and remediation into a cohesive platform. It provides comprehensive insights through statistical summaries, pattern analysis, and predictive measures.
- Metadata Management and Data Catalog: The platform features an AI-driven DataSense™ module for modernizing metadata, catalog, and taxonomy management. It includes search and query capabilities for metadata to facilitate data asset discovery.
- Data Curation and Master Data Management: DQLabs includes an ML-based smart curation module for automatic data curation and master data management. This allows for the creation and management of master records for various business entities.
- User-Friendly Interface and Customizable Workflows: The platform offers a user-friendly interface with role-based functionalities and the ability to define and execute custom workflows, combining out-of-the-box functionalities with customizable features.
Overall, DQLabs is a comprehensive solution that leverages AI, ML, and semantics to ensure high-quality data, making it an essential tool for organizations seeking to maintain data integrity and reliability.
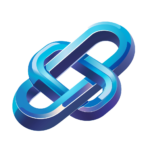
DQLabs - User Interface and Experience
User Interface Overview
The user interface of DQLabs is crafted with a strong focus on ease of use and user satisfaction, making it an intuitive and accessible tool for a wide range of users within an organization.
User-Friendly Interface
DQLabs boasts a no-code interface that simplifies the management of data quality, eliminating the need for specialized technical expertise. This interface reduces the learning curve for new users, enabling quicker adoption and shorter training periods. As a result, users can implement and utilize the software more rapidly, which enhances operational efficiency and productivity.
Streamlined Workflows
The platform features streamlined workflows and simplified navigation, allowing users to perform tasks more efficiently. This streamlined approach saves time and effort, leading to higher productivity and minimizing errors. The intuitive design ensures that users can focus on their tasks without getting bogged down in manual processes.
Persona-Based Dashboards
DQLabs offers persona-based dashboards that cater to the needs of different stakeholders within the organization. For example, data engineers and architects can monitor the overall health and reliability of the data, while data analysts and scientists can ensure the data is fit for purpose using custom tags and rules. Data leaders and Chief Data Officers can create customized dashboards and reports to track key data quality metrics and KPIs. This personalized approach ensures that each user can view data quality in a way that is relevant to their role.
Automated Monitoring and Alerts
The platform includes advanced anomaly detection capabilities powered by machine learning and AI. These ML models automatically detect trends and anomalies, providing real-time alerts categorized by priority. This automated monitoring ensures that data quality issues are promptly identified and addressed, minimizing human intervention.
Collaboration and Communication
DQLabs facilitates seamless communication and collaboration among users through conversational analytics. Users can comment on alerts, tables, or issues, enabling effective feedback loops between data producers and consumers. The platform also integrates with enterprise ticketing systems like Jira and ServiceNow, allowing users to create work tickets directly from the platform for issue remediation.
Accessibility for Non-Technical Users
The user-friendly interface makes complex data quality management tasks more accessible to non-technical users. By simplifying tasks and presenting information clearly, DQLabs promotes collaboration and data-driven decision-making across different teams and departments within the organization.
Integration and Connectivity
Connecting to various data sources is straightforward with DQLabs. The platform offers out-of-the-box smart connectors that allow users to manage data sources in any form, shape, or location without complex installations or agents. This ease of connectivity enhances the overall user experience by making data integration seamless.
Conclusion
In summary, DQLabs’ user interface is designed to be intuitive, efficient, and accessible. It simplifies data quality management, enhances user satisfaction, and promotes collaboration across different roles within an organization. The platform’s focus on ease of use and automation ensures that users can manage data quality effectively without requiring extensive technical expertise.
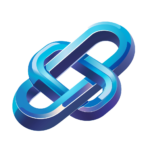
DQLabs - Key Features and Functionality
DQLabs Overview
DQLabs is a comprehensive Data Quality and Observability platform that integrates several key features to ensure reliable, accurate, and trustworthy data. Here are the main features and how they work:Smart Native Connectors
DQLabs offers out-of-the-box smart connectors that allow users to manage data sources from various locations and formats without complex installations or agents. This feature enables easy connection to data sources such as Snowflake, data warehouses, data lakes, and business intelligence tools, ensuring seamless integration and secure metadata retrieval.Business Dashboards and Insights
The platform provides readily available insights on alerts and data issues through business dashboards and configurable widgets. These dashboards can be customized to track key data quality metrics and KPIs, allowing different personas within the organization to view data quality issues relevant to their roles. This feature facilitates personalized and comprehensive views of the organization’s data quality landscape.ML-based Anomaly Detection
DQLabs uses advanced anomaly detection algorithms powered by machine learning (ML) and artificial intelligence (AI). These algorithms automatically detect anomalies across all metadata and custom measures, eliminating the need for manual threshold setting. The system identifies trends in the data and flags deviations, providing real-time alerts categorized by priority (low, medium, high) based on the severity of the anomaly.Drift and Behavior Analysis
The platform includes smart data quality and observability monitoring capabilities that adjust and adapt automatically for actionable alerts. This feature ensures that data quality issues are proactively identified and addressed, helping in maintaining consistent data quality and governance.Measure Data Quality
DQLabs scans various types of data assets in real-time and generates a trustworthy Data Quality score. This score can be tracked over time for continuous improvement, providing a clear metric to assess and enhance data quality.Semantic Discovery
The platform offers semantic-driven data discovery, allowing users to discover and extract semantics across their data store with just a few clicks. This feature auto-discovers rules and actively manages metadata, ensuring that data columns are automatically classified and understood within their business context.Usage Analytics
DQLabs monitors table and column usage across the data ecosystem by role or user over time. This helps in understanding which data assets are critical or unused, enabling better data management and optimization.Issue Management
The platform integrates seamlessly with third-party tools such as Slack, Teams, Jira, and ServiceNow to improve user collaboration. Users can create work tickets directly from the platform, assigning them to the appropriate teams for remediation. This feature facilitates effective collaboration and tracking of issue resolution.Improved Governance
DQLabs enhances governance and catalog management by integrating with major platforms. It powers active metadata strategies and ensures that data quality metrics are synchronized across different systems, such as the Atlan data catalog. This integration ensures real-time visibility and management of data quality at the asset level.Agentless Installation
The onboarding process with DQLabs is straightforward and agentless. Users can connect to data sources without complex installations, using only a service account with the necessary permissions. This simplifies the process of integrating new data sources into the platform.Persona-Based Dashboards and Customization
DQLabs allows users to create customized dashboards and reports tailored to their specific roles. For example, data leaders and Chief Data Officers can track key data quality metrics and KPIs through scheduled and delivered reports. This democratizes data quality management, aligning it with specific business requirements.No-Code Interface
The platform features an intuitive, no-code interface that empowers business users to customize rules, dashboards, and data contracts without specialized technical expertise. This allows business users to actively participate in data quality management, aligning processes with their specific business needs.AI and ML Integration
DQLabs leverages AI and ML extensively. It uses AI-augmented models for deep profiling, displaying distribution across value frequency or range frequency, and pattern analysis. The platform performs various anomaly detections in real-time, ensuring proactive identification and resolution of data issues. Additionally, it employs large language models (LLMs) and Generative AI (GenAI) for advanced data quality checks and enhanced metadata enrichment.Customizable Solution Capabilities
DQLabs offers more than 250 out-of-the-box data quality automatic rules across various checks such as Volume, Schema, Freshness, Data Reliability, Distribution, Frequency, Statistics, and Pattern matching. Users can also define custom rules and apply common monitoring rules for data quality, including rules related to unexpected values, nulls, schema changes, and more.Conclusion
Overall, DQLabs provides a cohesive platform that combines data observability, measurement, discovery, and remediation to streamline data quality processes and deliver reliable and accurate data. Its integration of AI and ML enhances the platform’s capabilities in anomaly detection, semantic discovery, and issue resolution, making it a valuable tool for organizations seeking to improve their data quality and governance.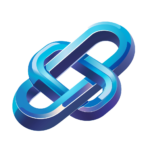
DQLabs - Performance and Accuracy
Evaluating DQLabs in the Data Tools AI-Driven Product Category
Evaluating the performance and accuracy of DQLabs in the Data Tools AI-driven product category involves examining several key aspects of its features and functionalities.
Performance
DQLabs demonstrates strong performance in several areas:
Automated Data Quality Checks
The platform offers over 50 out-of-the-box data quality checks across various categories such as health, frequency, distribution, and statistical measures. This automation helps in quickly identifying and addressing data quality issues.
Real-Time Monitoring
DQLabs provides continuous data monitoring with automated dashboards and alerts for data quality issues. This real-time monitoring ensures that any deviations from standard data are promptly identified and addressed.
Machine Learning and AI
The platform leverages machine learning (ML) and artificial intelligence (AI) to enhance data quality. ML algorithms automatically detect anomalies, identify errors, and suggest relevant data quality rules, which significantly improves the efficiency and accuracy of data management.
Accuracy
The accuracy of DQLabs is supported by several features:
Deep Profiling
DQLabs profiles data comprehensively, capturing structure, metadata, relationships, dependencies, and lineage. This detailed profiling is crucial for creating effective data quality rules and ensuring data accuracy.
Data Cleansing and Standardization
The platform identifies errors such as typos, invalid formats, and duplicate entries, and ensures consistent data formats throughout the environment. This helps in maintaining accurate and reliable data.
Semantic Discovery
DQLabs analyzes data attributes to identify or link semantic types, providing valuable context for rule creation. This semantic understanding enhances the accuracy of the data quality rules suggested by the platform.
Limitations and Areas for Improvement
While DQLabs offers a comprehensive suite of features, there are some challenges and areas that require attention:
Resource Requirements
Implementing a robust data quality program like DQLabs may require a skilled team with expertise in data governance, data cleansing, and data analysis. This can be a challenge due to the high demand and cost of hiring such professionals.
Data Consistency
Ensuring data consistency across different departments can be a hurdle. DQLabs helps in standardizing data formats, but the initial setup and integration may require significant effort to align with existing data practices.
Change Resistance
Shifting established data practices to adopt new tools like DQLabs can face internal resistance. Overcoming this resistance and demonstrating the immediate ROI of data quality initiatives is crucial for successful implementation.
Ownership and Governance
Clear ownership and a well-defined data governance framework are essential for the success of any data quality program. DQLabs can integrate with existing governance frameworks, but the organization must establish clear responsibilities and processes.
Engagement and User Experience
DQLabs fosters collaboration and knowledge sharing across teams through its intuitive user interface, interactive dashboards, and customizable workflows. This makes it easier for both business and technical stakeholders to engage with the platform and improve data quality.
In summary, DQLabs performs well in automating data quality checks, real-time monitoring, and leveraging AI and ML for enhanced accuracy. However, it is important for organizations to address potential limitations such as resource requirements, data consistency, change resistance, and the need for clear ownership and governance.
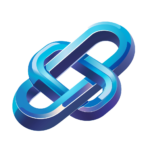
DQLabs - Pricing and Plans
Pricing Structure of DQLabs
The pricing structure of DQLabs, an AI-driven data quality platform, is not explicitly outlined in terms of specific tiers or prices in the available sources. Here are the key points regarding their pricing and plans:Custom Pricing
DQLabs provides custom pricing for their software, which means that the costs are quotation-based and vary depending on the specific needs and requirements of the organization.No Free Plan
DQLabs does not offer a free plan or a freemium model. Users must contact the vendor to get a quote for their business needs.Free Trial
DQLabs does offer a free trial, allowing potential users to test the software’s features and functionality before committing to a purchase.Plan Categories
While the exact pricing is not provided, DQLabs is categorized into different plans such as:- Essential Custom
- Professional Custom
- Enterprise Custom
Features
DQLabs includes a wide range of features such as:- Data Profiling
- Data Cleansing
- Data Matching
- Data Enrichment
- Data Monitoring
- Data Lineage
- Data Governance
- Data Integration
- Data Standardization
- Data Validation
- Data Masking
- Data Cataloging
- Data Stewardship
- Data Quality Rules, Dashboards, Metrics, Alerts, and Reports
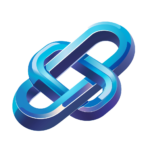
DQLabs - Integration and Compatibility
DQLabs Overview
DQLabs, an AI-driven data quality platform, is designed to integrate seamlessly with a wide range of tools and platforms, ensuring compatibility and efficiency across various data environments.
Integration with Data Warehouses and Tools
DQLabs connects effortlessly with key components of the modern data stack, including data warehouses, transformation tools, and BI platforms. This integration allows organizations to ensure data quality and observability without disrupting their existing workflows.
Data Catalog Integration
DQLabs supports bi-directional integration with leading data catalog tools, enabling centralized access to accurate and reliable data. Users can push and pull relevant data elements, such as tables, columns, and domains, between DQLabs and data catalogs. This integration enhances data trust and usability by providing clear visibility into data quality metrics and dimensions.
Third-Party Tools and Services
DQLabs integrates seamlessly with popular third-party tools and services, including issue management systems like Jira, Slack, Teams, and ServiceNow. This integration streamlines data quality workflows and reporting processes, allowing organizations to manage data quality issues within their current operational frameworks.
API Integration
The platform offers flexible API integration options, enabling organizations to incorporate DQLabs’ data quality features directly into their data pipelines and automated workflows. This allows developers to embed data quality checks, anomaly detection, and other functionalities into custom applications or processes, ensuring consistent data quality across different platforms.
Databricks Integration
Specifically, DQLabs integrates well with Databricks, allowing users to discover and profile data assets within their Databricks environment. It leverages Databricks’ compute power by running Spark SQL queries or using the existing Spark instance, all without moving data out of Databricks. This integration automates data profiling and cataloging, reducing manual effort and enhancing data discovery.
Cross-Platform Compatibility
DQLabs supports connections to offline, online, and real-time data sources using out-of-the-box connectors. The AI-driven DataSense™ module modernizes metadata, catalog, and taxonomy management, facilitating the discovery of data assets across various sources.
Conclusion
In summary, DQLabs’ integration capabilities are extensive and flexible, allowing it to work seamlessly with a variety of tools, platforms, and services. This ensures that data quality management is streamlined and efficient, making it easier for organizations to maintain high-quality data across their entire data ecosystem.
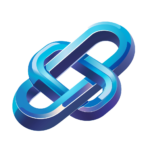
DQLabs - Customer Support and Resources
DQLabs offers a comprehensive suite of customer support options and additional resources to ensure users get the most out of their AI-driven data quality platform.
Customer Support Services
- DQLabs provides support services through designated Technical Support Contacts, who are knowledgeable in the use of the software and the customer’s operating environment. Customers can designate up to three Technical Support Contacts at any time.
- Support is available Monday to Friday from 8:00 AM to 8:00 PM EST, excluding federal holidays. The response times vary based on the severity of the issue: Urgent issues are addressed within 4 service hours, High severity issues within 6 service hours, and Normal/Low severity issues within 16 service hours.
Customer Success Manager
- Each customer is assigned a DQLabs Customer Success Manager at no additional cost. This manager serves as the primary point of contact and escalation, providing assistance with deployment strategies, feature usage, product updates, and managing issues reported by Technical Support Contacts.
Support Request Process
- Support requests are made through Trellance’s product support system and are managed via an assigned support queue. DQLabs uses commercially reasonable efforts to resolve each significant failure by providing a reasonable workaround, a solution update, or a specific action plan.
Additional Resources
- Documentation and Updates: DQLabs provides updates and revisions to the software and documentation, which are made available to customers. These updates include corrections and new releases of the software.
- User Communities and Forums: While the specific resources from DQLabs’ website are not detailed, similar platforms often offer access to user communities, forums, and online resources for technical assistance and customer services.
- Remote Support Analysis Module: This module includes features to trace activity to specific user logins, which can be disabled by the customer if needed. However, disabling this module may impair DQLabs’ ability to provide support services.
Product and Technical Assistance
- DQLabs offers comprehensive support through online and telephone contact information for technical assistance. This includes access to product downloads, support policies, and other helpful resources.
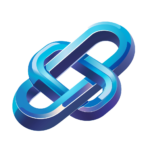
DQLabs - Pros and Cons
Advantages of DQLabs
Automation and Efficiency
DQLabs stands out for its ability to automate every aspect of data management, including scaling, governance, and automation. This AI-powered tool eliminates the need for manual intervention, such as ETL processes, workflows, and rules, allowing for autonomous execution without configuration.
Data Quality and Observability
The platform provides instant data quality alerts, measurements, and metrics, enabling all personas within an organization to collaborate and address data quality issues effectively. It promotes a decentralized data ownership culture, which improves data relevance and collaboration across different roles.
Integration and Compatibility
DQLabs is highly integrable with various tools within the data ecosystem, including data lakes, warehouses, transformation tools, and BI platforms. This versatility makes it a valuable addition to any modern data stack.
Decentralized Collaboration
The platform supports both “Data Minds” (such as Data Engineers, Data Scientists, and Data Analysts) and “Business Minds” (including Data Stewards, SMEs, and Data Leaders), allowing them to view and measure data quality in ways relevant to their roles. This collaborative approach ensures direct business outcomes regardless of the organization’s size or data maturity.
Scalability and Cost-Effectiveness
DQLabs can scale according to the organization’s data maturity cycle, making it suitable for both new and established data ecosystems. It offers cost-effective solutions, particularly in managing large datasets and reducing the need for costly data transformation processes upfront.
Automated Analysis and Resolution
The platform automates business quality checks and resolution using a semantic layer, delivering “fit-for-purpose” data for consumption across reporting and analytics. It also provides over 50 data quality checks, full-stack observability, and lineage tracking to proactively identify and address data issues.
Disadvantages of DQLabs
Learning Curve
While DQLabs automates many processes, its advanced features and AI-driven capabilities might require some time for users to fully grasp and utilize effectively. This could be a challenge for teams without extensive experience in AI and data management.
Resource Intensity
Although DQLabs is cost-effective in many aspects, it may require significant processing power, especially when dealing with raw data in data lakes. This could lead to higher operating expenses if extensive processing or quality management is necessary.
Schema Changes and Data Reconciliation
The platform, while managing schema changes efficiently, highlights that such changes can still cause serious production issues if not handled properly. This means that users need to be cautious and proactive in managing schema standardization to avoid data reconciliation nightmares.
Dependency on AI and Machine Learning
DQLabs relies heavily on AI and machine learning to make decisions and automate data management. This could be a drawback if the AI models are not accurately trained or if there are limitations in the data used to train these models.
In summary, DQLabs offers significant advantages in automation, data quality, and collaboration, but it also requires careful management of resources, schema changes, and a good understanding of its AI-driven features.
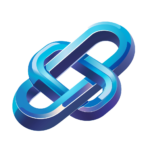
DQLabs - Comparison with Competitors
When Comparing DQLabs to Other AI-Driven Data Quality and Analytics Tools
Several key features and differences stand out.
Unique Features of DQLabs
- Semantic-Driven Data Discovery and Remediation: DQLabs uses a semantic-driven approach to automatically classify and understand the business context of data columns, leveraging a robust semantic layer and machine learning algorithms. This allows for auto-generation of data contracts and standardized rules for high-priority attributes.
- No-Code Interface: DQLabs empowers business users with an intuitive, no-code interface that enables them to customize rules, dashboards, and data contracts without needing specialized technical expertise.
- Advanced Anomaly Detection: The platform features machine learning-powered anomaly detection that automatically identifies trends and anomalies, providing real-time alerts categorized by priority. This ensures proactive identification and addressing of data quality issues with minimal human intervention.
- Integration with Enterprise Systems: DQLabs seamlessly integrates with enterprise ticketing systems like Jira and ServiceNow, allowing users to create work tickets directly from the platform for issue remediation.
Competitors and Alternatives
Plaid
- Market Share: Plaid is a significant competitor with a market share of around 73.66% in the data quality category. It is known for its extensive integration capabilities, particularly in the fintech sector, but may not offer the same level of semantic-driven data discovery as DQLabs.
- Features: While Plaid excels in data connectivity and aggregation, it may lack the advanced AI-driven data quality management features that DQLabs provides.
Segment
- Market Share: Segment holds around 24.26% of the market share in the data quality category. It is strong in customer data management but might not have the same focus on AI-driven data quality and governance as DQLabs.
- Features: Segment is more oriented towards customer data integration and analytics, which is different from DQLabs’ focus on comprehensive data quality management.
Collibra Data Quality
- Market Share: Collibra Data Quality has a smaller market share of about 0.67%. It is known for its data governance and cataloging capabilities, which align somewhat with DQLabs’ features.
- Features: Collibra focuses more on data governance and cataloging, whereas DQLabs combines these with advanced AI-driven data quality and anomaly detection.
IBM InfoSphere QualityStage
- Features: IBM InfoSphere QualityStage offers automated pattern detection and natural language query support through IBM Watson Analytics. However, it can be complex with a steep learning curve, which contrasts with DQLabs’ user-friendly, no-code interface.
- Integration: IBM InfoSphere QualityStage integrates well with other IBM tools but may not offer the same ease of connection to diverse data sources as DQLabs.
Tableau
- While Tableau is primarily a business intelligence platform, it does offer AI capabilities such as Tableau GPT and Tableau Pulse for enhanced data analysis and governance. However, it is not specifically focused on data quality management like DQLabs.
- Features: Tableau excels in data visualization and reporting but lacks the specialized AI-driven data quality features of DQLabs.
AnswerRocket
- AnswerRocket is a search-powered AI data analytics platform that allows users to ask questions in natural language to get insights. It is more focused on ad hoc analysis and reporting rather than comprehensive data quality management.
- Features: AnswerRocket is easy to use for non-technical users but lacks the advanced data quality and governance features that DQLabs offers.
Conclusion
In summary, DQLabs stands out with its semantic-driven approach, no-code interface, and advanced anomaly detection capabilities, making it a strong choice for organizations seeking a unified platform for data quality management. However, depending on specific needs, other tools like Plaid, Segment, or Collibra Data Quality might be more suitable for different aspects of data management and analytics.
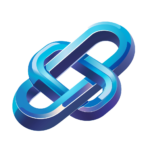
DQLabs - Frequently Asked Questions
Frequently Asked Questions about DQLabs
What is DQLabs and what does it do?
DQLabs is an AI-augmented data quality platform that helps organizations manage their entire data quality life cycle. It provides automated data quality checks, data observability, and metadata management to ensure data reliability and performance. The platform uses machine learning (ML) and large language models (LLMs) to offer advanced data quality checks and metadata enrichment.What are the key features of the DQLabs platform?
DQLabs offers several key features, including automated anomaly detection with over 250 out-of-the-box data quality rules, real-time data monitoring, and issue resolution at the attribute, table, and pipeline levels. It also includes adaptive auto thresholds using ML-based supervised learning, deep profiling, and pattern analysis. Additionally, the platform supports custom behavioral analysis, remediation workflows, and business dashboards for insights.How does DQLabs handle data quality monitoring?
DQLabs provides continuous data quality monitoring with auto thresholds and benchmarking. It uses ML-based supervised learning to set adaptive thresholds, allowing for the monitoring of any attributes across the organization. The platform also tracks row count, freshness, schema, and duplicates at the metadata level and performs deep column-level profiling.Can users create custom rules and workflows in DQLabs?
Yes, users can define and execute custom workflows in DQLabs. The platform allows users to create custom data quality rules and apply common monitoring rules related to unexpected values, nulls, schema changes, data volumes, data types, value frequencies, and formats. It also supports ML-based time-series anomaly detection for custom behavioral analysis.What types of data sources can DQLabs connect to?
DQLabs offers smart connectors that allow connections to an unlimited amount of data sources in any form, shape, and location. This includes offline, online, and real-time sources, facilitating comprehensive data profiling and curation.How does DQLabs support data curation and master data management?
DQLabs includes an ML-based smart curation module that automatically curates data based on metadata definitions. Users can schedule and automate data curation with controls on data quality thresholds. For master data management, the platform allows easy entity definition to create master records for customers, products, and other business entities, and supports automated processing and updating of these records.What kind of insights and dashboards does DQLabs provide?
DQLabs offers readily available insights on alerts and data issues across the data stack through business dashboards and configurable widgets. The platform provides comprehensive insights into data health through statistical summaries, pattern analysis, and predictive measures, along with quality metrics for each attribute.Is DQLabs user-friendly and accessible to different types of users?
Yes, DQLabs is designed to be user-friendly and accessible to a diverse audience, including data leaders, business stakeholders, data stewards, data scientists, data analysts, citizen users, data engineers, and DataOps professionals. The platform offers role-based functionalities to cater to different user needs effectively.What are the pricing plans for DQLabs?
DQLabs offers a Basic pricing plan that costs $2500 per month. There may be other plans available, but the details of those are not specified in the available resources.Does DQLabs support multiple languages?
Yes, DQLabs supports multiple languages, including English, French, German, Spanish, and Chinese (Simplified).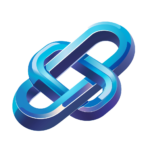
DQLabs - Conclusion and Recommendation
Final Assessment of DQLabs
DQLabs is a comprehensive and innovative platform in the Data Tools AI-driven product category, specializing in automated Data Quality and Data Observability. Here’s a detailed assessment of its benefits, target users, and overall recommendation.Key Benefits
- Automated Data Quality and Observability: DQLabs offers over 250 out-of-the-box automated data quality rules, covering aspects such as volume, schema, freshness, and more. It also employs AI and ML models for deep profiling, anomaly detection, and predictive measures, ensuring high data quality standards.
- Real-Time Anomaly Detection: The platform detects anomalies based on historical trends and alerts users with prioritized notifications, helping them focus on critical issues first. Users can also customize these alerts according to their specific dataset requirements.
- Comprehensive Insights and Dashboards: DQLabs provides rich dashboards, root-cause analysis, and impact analysis using lineage and semantics. This helps in gaining a clear picture of the data system’s health and making informed decisions.
- Integration and Collaboration: The platform integrates seamlessly with various third-party tools like Slack, Teams, Jira, and ServiceNow, enhancing user collaboration across the organization. It also supports multiple data sources through smart native connectors.
- Customizable Workflows: DQLabs allows users to define and execute custom workflows, combining out-of-the-box functionalities with customizable features. This flexibility is particularly beneficial for diverse user roles within an organization.
Target Users
DQLabs is tailored for a wide range of users, including:- Data Leaders (CDO/CDAOs): Who need comprehensive insights into data health and performance.
- Business Stakeholders (Data Governance Leaders): Who require accurate data to make informed business decisions.
- Data Stewards, Data Scientists, Data Analysts: Who benefit from automated anomaly detection and data quality checks.
- Citizen Users, Data Engineers, and DataOps professionals: Who can leverage the platform’s user-friendly interface and role-based functionalities.
Overall Recommendation
DQLabs is highly recommended for organizations seeking to improve their data quality, governance, and observability. Here are some key reasons:- Cost Savings: By ensuring high data quality, organizations can reduce the significant costs associated with poor data quality, estimated to be around $12.9 million annually according to Gartner.
- Competitive Advantage: Good quality data provides a clearer picture of industry dynamics, enabling more accurate marketing messages and better anticipation of customer needs. This can give organizations a competitive edge in their respective markets.
- User-Friendly and Customizable: The platform’s ability to cater to diverse user needs through its user-friendly interface and customizable workflows makes it a versatile tool for various roles within an organization.