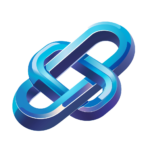
Google Cloud Recommendations AI - Detailed Review
E-Commerce Tools
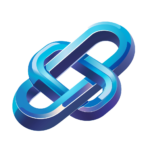
Google Cloud Recommendations AI - Product Overview
Google Cloud Recommendations AI
Google Cloud Recommendations AI is a sophisticated AI-driven tool within the e-commerce sector, aimed at providing personalized product recommendations to customers. Here’s a brief overview of its primary function, target audience, and key features:
Primary Function
Google Cloud Recommendations AI is designed to help businesses deliver personalized product recommendations to their customers. It leverages advanced machine learning algorithms to analyze historical data, including user interactions, product catalogs, and sales history, to predict future user behavior and suggest relevant items.
Target Audience
The primary target audience for Google Cloud Recommendations AI includes e-commerce businesses, retailers, and any organization looking to enhance user engagement and customer satisfaction through personalized recommendations. This tool is particularly beneficial for companies seeking to improve customer retention, increase sales, and optimize their recommendation strategies without requiring extensive machine learning expertise.
Key Features
Personalization
Recommendations AI uses various personalization techniques such as Federated Learning, Active Learning, and Local Memorization to create highly accurate and relevant recommendations based on individual user preferences and behaviors.
Scalability
Built on Google Cloud’s infrastructure, this service can handle large volumes of data and traffic, making it suitable for businesses of all sizes. It automatically manages infrastructure needs, including data preprocessing, model training, and load balancing, eliminating the need for manual intervention.
Integration
The service integrates seamlessly with existing systems and workflows, allowing for easy implementation. It supports data import from various sources such as Google Analytics, Google Tag Manager, BigQuery, Google Cloud Storage, and more.
Real-time Insights
Recommendations AI provides real-time analytics and insights that help businesses understand user behavior and optimize their recommendation strategies. It allows for customization of recommendation types and objectives, such as optimizing for click-through rate, click and play rate, or watch time.
Advanced Machine Learning
The service utilizes advanced machine learning models, including techniques like Content-Based Filtering, Collaborative Filtering, and Contextual Filtering. These models can correct for seasonality and bias, and they excel in situations with long-tail items and cold-start users.
By leveraging these features, Google Cloud Recommendations AI helps businesses deliver more accurate and timely recommendations, enhancing user satisfaction and driving revenue growth.
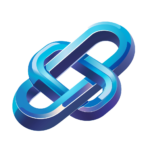
Google Cloud Recommendations AI - User Interface and Experience
User Interface of Google Cloud Recommendations AI
The user interface of Google Cloud Recommendations AI, particularly in the context of e-commerce tools, is designed to be user-friendly and intuitive, even for those without extensive machine learning expertise.
Ease of Use
Google Cloud Recommendations AI is integrated into the Google Cloud Platform (GCP) Retail console, which simplifies the setup and management process. Here are some key aspects that contribute to its ease of use:
- Straightforward Implementation: Users can create a project in GCP, import their product catalog, and determine the types of recommendations and their placements without needing deep ML knowledge.
- Integration with Existing Tools: The system seamlessly integrates with other Google tools such as Google Analytics and Google Tag Manager, allowing users to import existing user events easily.
- Step-by-Step Process: The process involves clear steps like creating a model, setting up placements, and deploying the model, all of which are guided through the Recommendations AI console.
User Experience
The overall user experience is enhanced by several features:
- Personalized Recommendations: The system provides highly personalized recommendations based on user interactions, such as purchases, adds to cart, and views. This personalization is achieved through advanced machine learning techniques like Federated Learning and Active Learning, which ensure the recommendations are relevant and timely.
- Real-Time Insights: Users can access real-time analytics and insights to understand user behavior and optimize their recommendation strategies. This helps in continuously improving the accuracy and effectiveness of the recommendations.
- Multiple Recommendation Types: The system offers different recommendation types, such as “Recommended for You,” “More Like This,” and others, which can be customized to fit specific needs like category pages or item detail pages.
- Customization and Filtering: Users can customize recommendations by setting business objectives (e.g., optimizing for click-through rate or watch time) and applying various filtering rules (e.g., item availability, price).
Deployment and Monitoring
The interface allows users to deploy the trained model into a production environment and monitor its performance. This includes setting up A/B tests to evaluate the model’s effectiveness and making necessary adjustments to improve accuracy over time.
Conclusion
In summary, Google Cloud Recommendations AI offers a user-friendly interface that simplifies the process of setting up and managing personalized recommendation systems, making it accessible to a wide range of users, even those without extensive technical expertise. The system’s focus on real-time insights, customization, and ease of integration with existing tools enhances the overall user experience.
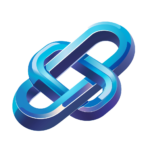
Google Cloud Recommendations AI - Key Features and Functionality
Google Cloud Recommendations AI
Google Cloud Recommendations AI is a sophisticated tool in the e-commerce sector, leveraging advanced machine learning techniques to provide personalized product recommendations. Here are the main features and how they work:
Personalization
Google Cloud Recommendations AI uses personalization techniques to deliver recommendations that align with individual user preferences. This is achieved through methods like:
- Federated Learning: Allows for collaborative model training while maintaining user privacy by training models on local data.
- Active Learning: Enables models to query users for feedback on uncertain predictions, refining the model’s accuracy over time.
- Local Memorization: Retains specific user interactions to enhance future recommendations, creating a more seamless user experience.
Scalability
The service is built on Google Cloud’s infrastructure, which means it can handle large volumes of data and traffic. This scalability makes it suitable for businesses of all sizes, ensuring that the system can grow with the business needs.
Integration
Google Cloud Recommendations AI integrates easily with existing systems and workflows. For instance, if you already use Google Shopping or Google Tag Manager, you can leverage these integrations to ingest data seamlessly into the Recommendations AI system.
Real-time Insights
The service provides real-time analytics and insights that help businesses understand user behavior and optimize their recommendation strategies. This includes monitoring the performance of recommendations and making necessary adjustments to improve accuracy.
Data Collection and Model Training
The process involves:
- Data Collection: Gathering data from various sources such as user interactions, product catalogs, and sales history.
- Model Training: Using AutoML to train custom models that can learn from the data and improve over time.
- Deployment: Implementing the trained model into the production environment to start generating recommendations.
- Monitoring and Optimization: Continuously monitoring the performance and making adjustments as necessary.
Recommendation Types
Google Cloud Recommendations AI offers several types of recommendations:
- Recommended for You: Based on the user’s shopping history, this type is often used on the home page.
- Other Items You May Like: Recommends other relevant items on the product detail page.
- Frequently Bought Together: Suggests complementary items to the one the user is considering buying.
- Shopping Cart and Purchase History: Uses the sequence of user events, including cart clicks and purchases, to inform the recommendations.
Business Rule Application
Businesses can apply various rules to fine-tune what customers see, such as diversifying product displays, filtering by product availability, and using custom tags. This ensures that the recommendations align with the business’s desired outcomes, whether it be engagement, revenue, or conversions.
Automatic Infrastructure Management
Google Cloud Recommendations AI automates several tasks, including data preprocessing, model training, hyper-tuning, load balancing, and infrastructure provisioning. This eliminates the need for manual intervention, allowing businesses to focus on other critical tasks.
Data Privacy and Ownership
The data and models used in Google Cloud Recommendations AI belong solely to the business. They are not used for any other Google product nor shared with other customers, and businesses have the freedom to delete their data and models at any time.
By integrating these features, Google Cloud Recommendations AI enables businesses to deliver highly personalized and effective recommendations, enhancing user satisfaction and driving revenue growth.
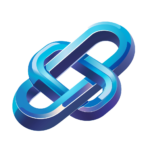
Google Cloud Recommendations AI - Performance and Accuracy
Evaluating the Performance and Accuracy of Google Cloud Recommendations AI
Evaluating the performance and accuracy of Google Cloud Recommendations AI in the e-commerce sector involves several key aspects:
Personalization and Accuracy
Google Cloud Recommendations AI excels in providing personalized recommendations by leveraging advanced machine learning techniques such as Federated Learning, Active Learning, and Knowledge Distillation. These methods enable the system to create models that are highly accurate and responsive to user behavior and preferences. For instance, Federated Learning allows for collaborative model training while maintaining user privacy, which is crucial for building trust and ensuring accurate predictions.
User Engagement
The personalization capabilities of Google Cloud Recommendations AI significantly enhance user engagement. By offering content and products that align with users’ interests, the system fosters a sense of control and satisfaction, leading to improved customer retention and loyalty. This is achieved through techniques like Local Memorization, which retains specific user interactions to enhance future recommendations.
Scalability and Integration
Google Cloud Recommendations AI is built on Google Cloud’s infrastructure, making it highly scalable and capable of handling large volumes of data and traffic. This scalability, combined with easy integration with existing systems and workflows, ensures that businesses of all sizes can implement and benefit from the service without significant disruptions.
Real-time Insights and Optimization
The service provides real-time insights and analytics that help businesses understand user behavior and optimize their recommendation strategies. This continuous monitoring and optimization process ensures that the recommendations remain accurate and relevant over time.
Limitations
Despite its strengths, there are some limitations to consider:
Data Privacy and Compliance
While Google Cloud Recommendations AI offers strong personalization, it is crucial to ensure compliance with data protection regulations. Businesses must carefully manage user data to avoid any privacy violations.
Model Evaluation
Regular evaluation of the model’s performance is necessary to identify areas for improvement. This involves actively seeking user feedback and continuously assessing the model’s accuracy to ensure it remains effective.
Contextual Limitations
The system may not always capture long-term trends or seasonal fluctuations in user behavior, as it primarily relies on recent data. This could lead to less accurate recommendations during periods of unusual activity, such as holidays or special events.
Areas for Improvement
To further enhance the performance and accuracy of Google Cloud Recommendations AI, several areas can be focused on:
User Feedback Mechanisms
Implementing more robust user feedback mechanisms, such as Active Learning, can help refine the model’s accuracy over time. This iterative process ensures the model adapts to changing user preferences.
Data Management
Effective data management strategies are essential. Using scalable data storage solutions and ensuring that data is properly managed can optimize the performance of the AI system.
Model Customization
Continuously evaluating and fine-tuning the models using techniques like Knowledge Distillation can help maintain high performance even in environments with limited computational resources.
In summary, Google Cloud Recommendations AI offers significant benefits in terms of personalization, user engagement, and scalability. However, it is important to address the limitations related to data privacy, model evaluation, and contextual understanding to ensure the system remains accurate and effective. By focusing on these areas, businesses can maximize the potential of this AI-driven tool in the e-commerce sector.
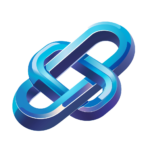
Google Cloud Recommendations AI - Pricing and Plans
General Availability and Pricing
Google Cloud’s Recommendations AI is available with a volume-based pricing model. Here are the key points:Volume-Based Pricing Tiers
Recommendations AI is offered with three volume-based pricing tiers for predictions. Additionally, there are separate charges for model training and tuning.Features and Tiers
- Predictions: The service is priced based on the volume of predictions made. There are three tiers, each with increasing volumes, but specific pricing details per tier are not provided in the available sources.
- Model Training and Tuning: There are additional costs associated with training and tuning the recommendation models. These models can be trained in as little as two to five days, depending on their complexity.
Free Options
While there isn’t a completely free tier for Recommendations AI, new Google Cloud customers can benefit from general free credits and free usage limits on various Google Cloud services. For example:- New customers receive $300 free credits to use towards any Google Cloud services, including Recommendations AI.
- However, Recommendations AI itself does not have a free tier; it requires a paid subscription based on the volume of predictions and model training needs.
API and UI Access
For the broader Recommender service (which includes Recommendations AI), all customers can access recommendations via the UI and API. The API quotas vary depending on the customer’s support package:- Basic or no support: Limited API quotas (e.g., 100 reads per minute, 100 reads per day).
- Standard, Enhanced, or Premium support: Higher API quotas (e.g., 6,000 reads per minute, 1,000,000 reads per day).
Additional Features
Customers with higher support packages (Standard, Enhanced, or Premium) can also export recommendations to BigQuery, which is not available for basic or no-support customers. In summary, while Recommendations AI does not offer a free tier, new customers can use their free credits to try out the service. The pricing is based on prediction volumes and model training costs, with varying API quotas and features depending on the customer’s support package.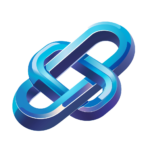
Google Cloud Recommendations AI - Integration and Compatibility
Integration with Google Cloud Platform (GCP)
Recommendations AI is part of the Google Cloud Platform and integrates closely with other GCP services. For instance, you can import your product catalog data from Google Merchant Center, Google BigQuery, or Google Cloud Storage, which simplifies the data ingestion process.Data Sources and Storage
The system allows you to import data from multiple sources, including JSON files or through POST requests. This flexibility ensures that you can use your existing data infrastructure without significant modifications. Additionally, integrating with Google BigQuery enables advanced analytics and machine learning capabilities.User Event Data
Recommendations AI records key user events such as purchases, adds to cart, and views of the cart page. These events are crucial for training the model and can be integrated into your existing website or app through API calls. This integration helps in creating personalized recommendations based on user behavior.API Integration
The Recommendations AI API can be integrated into your application to retrieve recommendations based on user queries. This involves making API calls to the RecommendationServiceClient, which allows you to fetch recommendations and display them to users in real-time.Compatibility with E-commerce Platforms
Recommendations AI can be integrated with various e-commerce platforms, including those using commercetools, a cloud-native, headless commerce platform. The commercetools connector in Google Cloud’s Application Integration facilitates this integration, enabling streamlined data connectivity and management across different applications and services.Cross-Device Compatibility
Since Recommendations AI is cloud-based, it can be accessed and integrated across various devices and platforms. This ensures that recommendations are consistent and personalized regardless of whether the user is interacting through a website, mobile app, or other digital touchpoints.Customization and Controls
The system offers flexibility in configuring recommendations based on user events and attributes. You can set up rules and controls that influence recommendations when certain events occur, such as a purchase or adding an item to the cart. This customization ensures that the recommendations are highly relevant and engaging for the users.Deployment and Evaluation
Recommendations AI models can be deployed as part of an A/B test to evaluate their performance. This allows you to compare the model’s recommendations against existing recommendation systems and fine-tune the model based on performance metrics such as precision and recall.Summary
In summary, Google Cloud Recommendations AI integrates seamlessly with various tools and platforms within the Google Cloud ecosystem and beyond, ensuring a comprehensive and personalized recommendation system that can be deployed across multiple devices and platforms.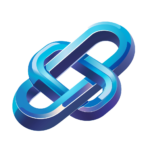
Google Cloud Recommendations AI - Customer Support and Resources
Customer Support and Resources for Recommendations AI
Personalized Recommendations
Google Cloud’s Recommendations AI is primarily focused on providing personalized product recommendations to customers. This is achieved through various machine learning models that analyze user behavior, preferences, and historical data. For instance, the Recommendations AI solution uses models like page-level optimization, revenue optimization, and a “buy-it-again” model to suggest products that are likely to interest the customer, thereby enhancing their shopping experience and driving sales.Real-Time Assistance for Customer Service Agents
While the Recommendations AI itself is more about product suggestions, Google Cloud’s broader Customer Engagement Suite, which includes tools like Agent Assist, provides significant support for customer service operations. Agent Assist offers real-time assistance to customer care representatives, including generated responses, real-time coaching, and conversation summarization. This helps agents resolve customer issues more efficiently and accurately.Analytics and Insights
To ensure the effectiveness of AI-driven recommendations, Google Cloud provides analytics tools that help retailers monitor key metrics such as conversion rates, clickthrough rates, and customer sentiment. These insights are crucial for optimizing the recommendation systems and improving overall customer engagement. Tools like Conversational Insights analyze real-time data from customer operations to provide KPIs, identify call drivers, and highlight areas for improvement.Integration with Existing Systems
Google Cloud’s Recommendations AI and Customer Engagement Suite are designed to integrate seamlessly with existing technologies, including telephony, CRM systems, and workforce management tools. This integration enables a cohesive and efficient customer service operation across all channels.Development and Implementation Resources
For developers and businesses looking to implement these solutions, Google Cloud provides comprehensive resources. This includes documentation, tutorials, and how-to guides for setting up and using the Recommendations AI API, as well as other tools within the Customer Engagement Suite. Developers can also find quickstart guides and code examples to help them get started with integrating these AI tools into their applications.Conclusion
In summary, Google Cloud’s Recommendations AI and associated customer engagement tools offer a suite of features that enhance customer support through personalized recommendations, real-time agent assistance, and detailed analytics, all while ensuring smooth integration with existing systems and providing extensive resources for implementation.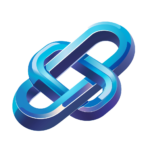
Google Cloud Recommendations AI - Pros and Cons
Advantages of Google Cloud Recommendations AI in E-Commerce
Google Cloud Recommendations AI offers several significant advantages for retailers in the e-commerce sector:Personalization
The AI-driven recommendation system allows for highly personalized interactions with customers. It uses a product-pattern recognizer to analyze customer behavior, such as clicks, cart activities, and purchases, to determine their preferences and adjust search and browse results accordingly. This personalization can lead to a more engaging and relevant shopping experience, which is crucial since 75% of shoppers prefer brands that personalize interactions.Revenue Optimization
Recommendations AI includes a revenue optimization feature that uses machine learning to balance long-term customer satisfaction with short-term revenue goals. This feature combines product categories, item prices, and customer interactions to suggest products that can increase revenue per user session. Retailers have seen double-digit uplift in conversion and clickthrough rates using this technology.Efficiency and Automation
The system automates the process of curating e-commerce pages, saving retailers time and resources that would be spent on manual curation. This automation helps in maintaining well-stocked and right-sized in-store shelves and e-commerce pages, ensuring product availability and reducing the effort required to manage inventory.Global Availability and Multi-Language Support
The new capabilities, including the Recommendations AI, are generally available to retailers worldwide and support 72 languages. This makes it accessible and useful for a broad range of international retailers.Data Control
Retailers maintain full control over their customer data, which is not linked to Google account activity. This ensures that sensitive customer information remains secure and within the retailer’s control.Disadvantages of Google Cloud Recommendations AI in E-Commerce
While Google Cloud Recommendations AI offers several benefits, there are also some limitations to consider:Limited Context
The recommendations are based on recent customer behavior and do not take into account long-term trends or seasonal fluctuations. This might lead to recommendations that are not optimal for all periods, such as holiday seasons or special events.Lack of Holistic Optimization
The Recommendations AI focuses on optimizing specific aspects of the e-commerce experience but does not provide a holistic view of the entire e-commerce strategy. It does not suggest changes that might involve moving to different cloud services or adopting a multi-cloud approach.Need for Human Oversight
While the AI generates valuable insights, it is important to have human reviewers assess the recommendations to ensure they align with the retailer’s unique business needs and do not disrupt system performance or security.Dependency on Data Quality
The effectiveness of the recommendations depends on the quality and accuracy of the data collected from customer interactions. Poor data quality can lead to less accurate and less relevant recommendations. By considering these advantages and disadvantages, retailers can make informed decisions about how to integrate Google Cloud Recommendations AI into their e-commerce strategies.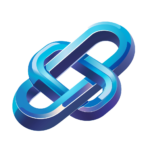
Google Cloud Recommendations AI - Comparison with Competitors
When comparing Google Cloud Recommendations AI with other AI-driven product recommendation tools in the e-commerce sector, several key features and differences stand out.
Unique Features of Google Cloud Recommendations AI
- Advanced Machine Learning: Google Cloud Recommendations AI leverages state-of-the-art machine learning models, including techniques like Federated Learning, Active Learning, and Knowledge Distillation, to provide highly accurate and personalized recommendations.
- Scalability and Automation: The service is built on Google Cloud’s infrastructure, which automatically handles data preprocessing, model training, and infrastructure provisioning, making it scalable and efficient for businesses of all sizes.
- Real-time Insights and Optimization: It provides real-time analytics and insights, allowing businesses to monitor and optimize their recommendation strategies continuously. This includes the ability to apply business rules to fine-tune recommendations based on various criteria such as product availability and custom tags.
- Data Privacy and Security: Google Cloud ensures that retailer data is isolated with strong access controls and is not used for any other Google product or shared with other customers. This provides a high level of data security and privacy.
Alternatives and Comparisons
Amazon Personalize
- Amazon Personalize is another prominent AI-driven recommendation service. It allows users to build, deploy, and manage custom recommendation models using machine learning algorithms. While it offers similar scalability and real-time capabilities, it may require more manual configuration and tuning compared to Google Cloud Recommendations AI.
- Amazon Personalize integrates well with other AWS services, which can be an advantage for businesses already invested in the AWS ecosystem.
Salesforce Einstein
- Salesforce Einstein provides AI-powered recommendations as part of its broader CRM and marketing suite. It focuses on personalizing customer experiences across various touchpoints, including email, mobile, and web.
- Unlike Google Cloud Recommendations AI, Salesforce Einstein is more tightly integrated with Salesforce’s CRM and marketing tools, making it a better fit for businesses already using Salesforce products.
BigCommerce with Google Cloud AI
- BigCommerce has integrated Google Cloud AI tools, including Recommendations AI, to enhance its e-commerce platform. This integration allows merchants to create personalized storefronts and product recommendations, similar to what Google Cloud Recommendations AI offers. However, this is more of an integrated solution within the BigCommerce platform rather than a standalone recommendation service.
Key Differences
- Ease of Use: Google Cloud Recommendations AI stands out for its automated processes, requiring minimal manual intervention in data preprocessing, model training, and infrastructure management. This makes it more user-friendly for businesses that may not have extensive AI expertise.
- Integration: While Amazon Personalize and Salesforce Einstein offer strong integration with their respective ecosystems, Google Cloud Recommendations AI is more versatile and can integrate with a wide range of e-commerce platforms, including Shopify and BigCommerce.
- Customization: Google Cloud Recommendations AI allows for extensive customization through business rules and various machine learning techniques, making it highly adaptable to different business needs and goals.
Conclusion
In summary, Google Cloud Recommendations AI is distinguished by its advanced machine learning capabilities, scalability, and automated processes, making it a strong choice for businesses seeking to enhance their e-commerce operations with AI-driven product recommendations. However, the best choice ultimately depends on the specific needs and existing technology stack of the business.
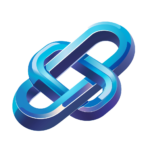
Google Cloud Recommendations AI - Frequently Asked Questions
Frequently Asked Questions about Google Cloud Recommendations AI
Q: What is Google Cloud Recommendations AI and how does it work?
Google Cloud Recommendations AI is a machine learning-based solution that helps retailers deliver personalized product recommendations to their customers. It uses historical data, such as customer clicks, purchases, and other interactions, to optimize product ordering and recommendations on ecommerce sites. The system learns from user behavior to provide relevant and personalized suggestions, enhancing the shopping experience and increasing the likelihood of sales.
Q: How does Recommendations AI handle product recommendations after a customer makes a purchase?
When a customer buys a product, the Recommendations AI system adjusts its recommendations based on the user’s behavior. While it does not immediately stop recommending the purchased product, it typically lowers the priority of that item in subsequent recommendations. This behavior can be customized and fine-tuned based on the retailer’s configuration.
Q: Can I configure recommendations based on user events such as purchases or adding items to the cart?
Yes, Recommendations AI allows you to set up rules and controls that influence recommendations when certain events occur, such as a purchase or adding an item to the cart. These event-based rules can adjust the user’s preferences or alter the recommendation logic for subsequent interactions.
Q: Does Recommendations AI use cookies in the process of importing data, training models, and deploying recommendations?
Recommendations AI may use cookies as part of the end-to-end process, but it is possible to perform these actions without using cookies. However, the exact details on cookie usage can depend on the specific implementation and configuration.
Q: How does Recommendations AI optimize product ordering on ecommerce pages?
Recommendations AI uses machine learning to dynamically optimize the ordering of products on ecommerce pages. It selects the optimal ordering based on historical data, ensuring that the most relevant and likely-to-be-purchased products are displayed first. This optimization helps in improving accuracy, relevance, and the likelihood of making a sale.
Q: What are the benefits of using Recommendations AI for retailers?
Using Recommendations AI can significantly benefit retailers by increasing conversion and clickthrough rates, enhancing customer satisfaction, and lifting revenue per user session. The system has shown double-digit uplift in these metrics in experiments conducted by retailers using the technology.
Q: How does Recommendations AI integrate with other Google Cloud services and ecommerce platforms?
Recommendations AI can be integrated with various ecommerce platforms, such as Shopify and BigCommerce, to leverage Google Cloud’s advanced search and AI capabilities. This integration allows retailers to access Google-quality search and AI innovations directly through their ecommerce solutions.
Q: Are there any new features or upgrades in Recommendations AI that retailers should be aware of?
Recent upgrades include new machine learning capabilities that enable retailers to dynamically optimize product ordering and recommendations panels. There is also a revenue optimization feature that uses machine learning to offer better product recommendations, and a buy-it-again model that provides personalized recommendations for repeat purchases.
Q: How does the pricing model work for Recommendations AI?
Google Cloud generates most recommendations and insights for free to all customers. However, premium recommendations require the purchase of a specific SKU. The availability and API quotas vary depending on the customer’s support package, with higher quotas available for Standard, Enhanced, and Premium support packages.
Q: Can I export recommendations data to other tools like BigQuery?
Yes, customers with a Standard, Enhanced, or Premium support package can export recommendations via BigQuery. This allows for further analysis and integration with other data analytics tools.
By addressing these questions, retailers can better understand how Google Cloud Recommendations AI can enhance their ecommerce operations and improve customer experiences.
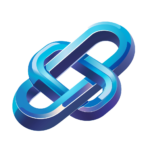
Google Cloud Recommendations AI - Conclusion and Recommendation
Final Assessment of Google Cloud Recommendations AI
Google Cloud Recommendations AI is a powerful tool in the e-commerce sector, particularly for businesses aiming to enhance user engagement and drive revenue through personalized product recommendations.Key Benefits
- Personalization: This service excels in providing highly personalized recommendations based on user interactions, purchase history, and demographic information. This personalization leads to more accurate predictions and a better user experience.
- Scalability: Built on Google Cloud’s infrastructure, Recommendations AI can handle large volumes of data and traffic, making it suitable for businesses of all sizes.
- Real-time Insights: The platform offers real-time analytics and insights, enabling businesses to monitor and optimize their recommendation strategies continuously.
- Ease of Use: The service automates many processes, including data collection, model training, and deployment, eliminating the need for manual preprocessing, training, or hypertuning of machine learning models.
Who Would Benefit Most
- Retailers: E-commerce sites can significantly benefit from Google Cloud Recommendations AI by delivering dynamic and personalized product recommendations, which can increase sales, average order value, and customer lifetime value. Companies like IKEA Retail and Hanes Australasia have already seen positive results, such as increased average order value and revenue per session.
- Media Companies: Media organizations can use this AI to recommend personalized content and advertisements, leading to increased engagement, viewership, and ad revenue.
Overall Recommendation
Google Cloud Recommendations AI is highly recommended for any business in the e-commerce or media sector looking to enhance user engagement and drive revenue through personalized recommendations. Here are a few key reasons why:- Improved User Experience: By providing recommendations that align with user preferences, businesses can foster a sense of control and satisfaction among their customers, leading to higher engagement and retention.
- Business Revenue Growth: Personalized recommendations can lead to increased sales, higher average order values, and improved customer lifetime value, ultimately driving revenue growth.
- Efficient Data Management: The service offers scalable data storage solutions and automated data management, which are crucial for handling large datasets efficiently.