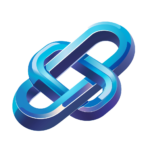
Moodle Analytics - Detailed Review
Education Tools
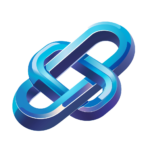
Moodle Analytics - Product Overview
Introduction to Moodle Analytics
Moodle Analytics is a powerful tool within the Moodle Learning Management System (LMS) that helps educators and administrators make data-driven decisions to improve student outcomes and educational processes.
Primary Function
The primary function of Moodle Analytics is to analyze the vast amount of data generated by the Moodle LMS to provide valuable insights into student behavior, performance, and engagement. This data-driven approach enables educational institutions to identify trends and patterns, predict student success, and make informed decisions about instructional strategies, resource allocation, and curriculum design.
Target Audience
The target audience for Moodle Analytics includes educators, teachers, and administrators within educational institutions. This tool is particularly useful for those who need to monitor student progress, identify areas where students may struggle, and optimize the learning environment to meet student needs.
Key Features
Data Analysis and Insights
Moodle Analytics uses sophisticated data analytics methodologies to analyze student interactions with the Moodle platform. This helps in identifying student behavior patterns, learning preferences, and performance indicators.
Predictive Models
The tool includes predictive models that can forecast student success or identify students at risk of dropping out. For example, the “Students at risk of dropping out” model can send automated notifications to students who are likely to fail, allowing for early intervention.
Learning Analytics API
Moodle’s Learning Analytics API is an open system that supports various models for learning analytics. It includes indicators, targets, insights, notifications, and actions, which can be customized and extended through plugins.
Compliance and Integration
Moodle Analytics ensures compliance with data protection regulations like GDPR, as all data processing occurs within the Moodle ecosystem, eliminating the need to extract data to external platforms.
Institutional and Classroom Level Decision-Making
The tool supports decision-making at both the classroom and institutional levels. Educators can use it to adjust instructional tactics, while administrators can assess program efficacy, distribute resources more effectively, and make informed policy decisions.
By leveraging these features, Moodle Analytics helps educational institutions continuously improve their practices, enhance student outcomes, and foster a culture of continuous development.
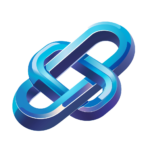
Moodle Analytics - User Interface and Experience
User Interface
Moodle provides a personalized dashboard for users, which can be customized to display relevant information such as course details, upcoming deadlines, and important dates. This dashboard extends to the analytics section, where users can access various reports and analytics tools. The interface is clean and organized, allowing users to quickly locate and analyze the data they need.
Ease of Use
The analytics tools in Moodle are generally easy to use, even for those who are not highly tech-savvy. The system offers built-in reports based on log data, which provide a holistic picture of participant engagement over a specified period. These reports are descriptive in nature, making it clear what actions have been taken, by whom, and when.
For more advanced analytics, Moodle integrates with third-party plugins such as IntelliBoard and LearnerScript, which are Moodle Certified Integrations. These integrations offer highly visual graphs, ready-to-use dashboard blocks, and the ability to schedule automated reports. This makes it simpler for users to analyze and interpret the data without needing extensive technical knowledge.
User Experience
The overall user experience is enhanced by the customizable nature of the dashboard and the clear presentation of data. Users have reported that Moodle is generally easy to use, with a usability rating of 3.96 out of 5 in some studies. This ease of use is crucial for busy educators who need to quickly access and analyze learning data to improve course content and student engagement.
However, there are some areas where users might encounter minor issues, such as finding specific information like feedback and grades on the dashboard. User feedback has highlighted the need to surface such critical information more prominently to improve the user experience further.
Conclusion
In summary, Moodle Analytics offers a user-friendly interface that is easy to navigate, with clear and descriptive reports that help educators make informed decisions about their courses and student engagement. While there may be some minor usability issues, the overall experience is positive and supportive of educational goals.
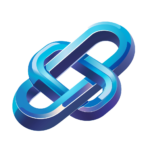
Moodle Analytics - Key Features and Functionality
Moodle Analytics and AI Integration
Moodle Analytics, particularly when integrated with AI, offers a range of powerful features that significantly enhance the learning experience and educational outcomes. Here are the main features and how they work:Learning Analytics Models
Moodle Analytics uses models to predict various outcomes, such as student performance and engagement. The core Moodle LMS includes three built-in models:Students at Risk of Dropping Out
This model predicts which students are likely to drop out based on their engagement and performance indicators. It helps educators identify at-risk students early and implement interventions to improve their learning outcomes.Upcoming Activities Due
This model notifies educators and students about upcoming activities and deadlines, ensuring everyone stays on track.No Teaching
This model identifies courses or sections where no teaching activity is occurring, helping administrators ensure that all courses are actively managed.Customizable Models and Plugins
In addition to the core models, Moodle allows for the creation and installation of custom models through plugins. This flexibility enables institutions to adapt the analytics to their specific needs.Indicators, Targets, Insights, Notifications, and Actions
Each model can include indicators (predictors), targets (the outcome being predicted), insights (the predictions), notifications (messages sent based on insights), and actions (suggested steps for recipients of notifications).AI-Driven Personalized Learning
AI integration in Moodle Analytics enables personalized learning experiences. AI algorithms analyze student data and behaviors to recommend customized learning paths, content, and assignments that cater to individual learning styles and speeds.Customized Learning Paths
AI suggests learning paths that are tailored to each student’s needs, ensuring that the learning material is neither too easy nor too challenging.Adaptive Learning Modules
These modules adjust the content based on individual progress and performance, providing a personalized and engaging experience.Automated Assessment and Feedback
AI-powered assessment tools within Moodle can automatically grade assignments, quizzes, and even essays. This reduces the administrative burden on educators and provides students with instant feedback, facilitating a faster learning feedback loop.Intelligent Assessments
AI adapts assessments to each learner’s skill level, ensuring that questions are relevant and challenging enough to accurately assess their understanding.Predictive Analytics
Moodle Analytics, enriched with AI, can predict learner behavior and identify potential dropouts or areas where students might struggle. This allows educators to intervene proactively and offer additional support or resources.Early Intervention
Predictive analytics help educators identify at-risk students early, enabling timely interventions to improve learning outcomes.Insights and Notifications
Educators can view insights and predictions, such as selecting insights per course with the “Students at risk of dropping out” model. The system also provides notifications based on these insights, ensuring that educators and students are informed about critical issues.Evaluation Logs
Educators can view previous evaluation logs, including learning curve graphs and TensorBoard logs, to track student progress over time.AI-Powered Support
AI-powered chatbots integrated into Moodle provide 24/7 support to students, answering questions, providing guidance, and assisting with course navigation. This enhances the overall user experience and frees up educators to focus on more complex tasks.Immediate Feedback and Support
Chatbots equipped with Natural Language Processing (NLP) capabilities offer instant support and guidance, enhancing the learning experience.Data-Driven Insights
AI analyzes learner data to provide educators with insights into course effectiveness. This data-driven approach helps educators optimize their courses for better results, fostering continuous improvement.Course Optimization
By analyzing learner data, educators can fine-tune course content and strategies to achieve better learning outcomes.Automation of Routine Tasks
AI can automate routine administrative tasks such as grading assignments or tracking attendance, freeing up educators to focus on more valuable aspects of teaching.Efficient Administration
Automation of routine tasks reduces the administrative burden, allowing educators to concentrate on teaching and mentoring. By integrating AI into Moodle Analytics, educators can create a more engaging, effective, and personalized learning environment, ultimately leading to better educational outcomes.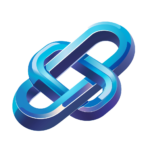
Moodle Analytics - Performance and Accuracy
Evaluating the Performance and Accuracy of Moodle Analytics
Evaluating the performance and accuracy of Moodle Analytics, particularly in the context of education tools using AI, involves several key aspects.
Performance
Moodle Analytics leverages machine learning models to predict learning outcomes, such as identifying students at risk of dropping out or detecting no teaching activity. These models are trained on historical data from the site, which enables them to make predictions based on patterns and trends identified in the data.
Model Training and Data
For these models to perform accurately, they must be trained on a site with previous completed courses. This training is crucial because the models cannot make predictions until they have sufficient historical data to analyze.
Multiple Models
Moodle supports running multiple prediction models simultaneously, even within the same course. This capability allows for A/B testing to compare the performance and accuracy of different models, helping educators determine which models are most effective.
Accuracy
The accuracy of Moodle Analytics is heavily dependent on the quality and quantity of the data available.
Data Requirements
Models require fixed start and end dates for courses to make accurate predictions. This limitation means that models may not be suitable for courses with rolling enrollments until future updates address this issue.
Model Evaluation
Predictions are evaluated against actual outcomes, typically after specific intervals of the course (e.g., quarters or tenths). The “quarters accumulative” and “tenths accumulative” options tend to generate more accurate predictions by including data from all previous intervals, although they may require more time and memory to execute.
Limitations
While Moodle Analytics offers significant insights, there are several limitations to consider:
Initial Configuration
The system requires initial configuration and the enabling of models, which must be aligned with the educational priorities of the institution. Models are not enabled by default and need careful consideration before use.
Data Dependency
Models must be trained on local site data, which can be a limitation if the site lacks sufficient historical data. There is an ongoing need for diverse data sets from various Moodle-using institutions to improve the models’ general applicability.
Course Type Restrictions
Current models are best suited for courses with fixed start and end dates, making them less effective for courses with rolling enrollments.
Areas for Improvement
Expansion of Model Types
Future versions of Moodle are expected to include models that support a wider range of course types, such as those with rolling enrollments.
Broader Data Integration
There is a continuous need for more diverse data sets to enhance the models’ accuracy and applicability across different educational settings.
User Accessibility
Currently, models and predictions are only visible to teachers and administrators. Expanding accessibility to other stakeholders could enhance the utility of the analytics.
In summary, Moodle Analytics performs well in predicting learning outcomes and identifying engagement patterns, but its accuracy is highly dependent on the availability and quality of historical data. Addressing the limitations, particularly around data requirements and course types, will be crucial for improving its performance and accuracy.
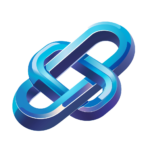
Moodle Analytics - Pricing and Plans
Pricing Structure of Moodle
When considering the pricing structure of Moodle, it’s important to distinguish between the core Moodle platform and the various analytics tools and plugins that can be integrated into it.
Core Moodle Platform
- Free Plan: Moodle itself is free to download and use, making it an attractive option for individual educators or small organizations. However, this plan requires self-hosting, which demands technical knowledge for installation and maintenance.
MoodleCloud Plans
- For those who prefer a hosted solution with minimal technical requirements, MoodleCloud offers several plans. The pricing starts at $120 per year for 50 users, making it suitable for small to medium-sized organizations. These plans are scalable and provide enhanced security and data protection.
Moodle Certified Partners
- Larger organizations or those requiring extensive customization and dedicated support can opt for Moodle Certified Partners. These partners provide a range of services including hosting, customization, support, and training. The pricing varies based on the services and scale of the deployment.
Analytics and Reporting Plugins
- While Moodle itself does not have a specific pricing tier for analytics, various plugins and tools can be integrated to enhance analytics capabilities.
- Free Plugins: Plugins like My Feedback are free and provide basic feedback reporting features.
- Paid Plugins: Tools like LearnerScript offer advanced learning analytics and reporting features, with a one-time purchase fee of $2450. This plugin is particularly useful for small to medium-scale Moodle LMSs.
- Zoola and Other Tools: Other reporting platforms like Zoola offer customizable dashboards and detailed reports, but their pricing is not specified in the context of Moodle plans. These tools are often integrated via API solutions.
Key Features by Plan
Free Plan:
- Self-hosting required
- Access to core Moodle features
- Limited support
MoodleCloud Plans:
- Hosted solution
- Scalable pricing starting at $120/year for 50 users
- Enhanced security and data protection
- Access to key Moodle features
Moodle Certified Partners:
- Customized hosting and support
- Extensive customization and training
- Pricing varies based on services and scale
In summary, while Moodle itself is free, the costs associated with hosting, implementation, and support can vary widely depending on the chosen plan and any additional analytics or reporting tools integrated into the platform.
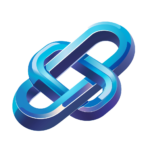
Moodle Analytics - Integration and Compatibility
Moodle’s Analytics Capabilities
Moodle’s analytics capabilities are highly integrative and compatible with various tools and platforms, making it a versatile option for educational institutions and organizations.Built-in Analytics and API
Moodle has an inherent analytics system that provides a range of reports based on log data, giving educators a comprehensive view of learner engagement over time. The Moodle Learning Analytics API allows for the setup of analytics to track specific metrics and make predictions based on user data. This API is open and can be extended with new custom models using reusable targets, indicators, and other components.Third-Party Integrations
For more comprehensive insights, Moodle offers certified integrations with third-party analytics tools. Two notable integrations are IntelliBoard and LearnerScript. These tools seamlessly integrate with Moodle to provide enhanced reporting and learning analytics. IntelliBoard collects and analyzes LMS data to help evaluate and improve eLearning initiatives, including identifying at-risk learners through predictive analytics. LearnerScript helps measure learning outcomes, understand unique learner needs, and provides actionable insights to improve courses and programs.Device and Browser Analytics
The Device Analytics plugin is another useful integration that allows administrators to capture statistics on the devices and browsers used by learners. This plugin provides a quick overview of user devices, including operating systems, display sizes, and input types, all presented in graphical and tabular dashboards. This is particularly useful for understanding the technical environment of your learners.Integration with External Tools
Moodle supports the integration of external tools through Learning Tools Interoperability (LTI) protocols. This allows tools like Zoom Meetings and Poodll to be connected to Moodle. Similarly, AI systems that follow LTI and x-API protocols can potentially be integrated to diagnose learners’ progress, prescribe learning paths, and recommend content, such as h4P content. However, the specific requirements and feasibility of integrating AI engines with Moodle and h4P would need to be discussed in detail with Moodle partners or the developers of the AI systems.Cross-Platform Compatibility
Moodle’s analytics tools and integrations are generally compatible across various devices and platforms. The Device Analytics plugin, for example, helps administrators understand the diverse range of devices learners use, ensuring that the learning environment is optimized for different browsers, operating systems, and devices.Customization and Extensibility
The Moodle Learning Analytics system is highly customizable and extensible. Models can be designed and selected to match the educational priorities of the institution, and the system can be extended with new custom models based on PHP and Python machine learning backends.Conclusion
In summary, Moodle’s analytics capabilities are highly integrative, compatible with various tools and platforms, and can be customized to meet the specific needs of educational institutions. This ensures that educators can make data-driven decisions to enhance learner engagement, persistence, and retention.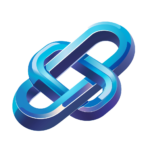
Moodle Analytics - Customer Support and Resources
Support Channels
Moodle, being an open-source project, does not offer traditional customer support channels like live chat, phone support, or email support. However, there are several alternative ways to get help:
- Forums and Community Support: Moodle has an active community forum where you can post questions and get answers from other users and experts. This is a valuable resource for troubleshooting and getting insights from experienced users.
- Social Media: You can reach out to Moodle through their social media platforms to ask questions or seek assistance.
- Knowledge Base and Self-Service Support: Moodle provides a comprehensive knowledge base that includes guides, tutorials, and troubleshooting tips. This self-service support can help you resolve many common issues on your own.
Additional Resources
For specific needs related to Moodle Analytics, here are some additional resources:
- Learning Analytics API and Models: Moodle offers the Learning Analytics API, which allows for the creation and use of various analytics models. These models can predict outcomes such as students at risk of dropping out, upcoming activities due, and more. You can also install additional plugins from the Moodle plugins directory to extend these capabilities.
- Documentation and Guides: The Moodle documentation provides detailed specifications and guides on how to use and configure the Learning Analytics system. This includes information on indicators, targets, insights, notifications, and actions within the analytics models.
- Moodle Partners: If you need paid support, you can contact Moodle Partner companies. These partners offer professional support services that can help with more complex issues or customized solutions.
Faculty and User Guides
For faculty and users, there are specific guides available that can help with setting up and using Moodle effectively. These guides cover topics such as course setup, using specific features, and troubleshooting common issues. For example, the Faculty Quick Start Guide provides detailed information on using Moodle 4.0 and its updated features.
By leveraging these resources, you can effectively use Moodle and its analytics features to enhance your educational experience.
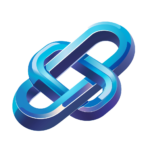
Moodle Analytics - Pros and Cons
Advantages of Moodle Analytics
Moodle Analytics offers several significant advantages that can enhance educational outcomes and decision-making processes.Insightful Data Analysis
Moodle Analytics provides educators and administrators with valuable insights into student behavior, performance, and engagement. It uses sophisticated data analytics methods to analyze logins, participation rates, forum activity, and time spent on learning activities, helping to identify patterns and trends in student engagement and motivation.Predictive and Proactive Capabilities
The system includes machine-learning based models and static models that can predict outcomes such as students at risk of dropping out. These models enable early intervention, allowing educators to send targeted messages or review detailed activity reports to support at-risk students.Personalized Learning
By analyzing engagement metrics and performance data, educators can develop a thorough understanding of student behavior and preferences. This data-driven approach helps in personalizing learning for each student, promoting academic success and creating a more engaging and inclusive learning environment.Institutional Decision-Making
Moodle Analytics supports data-informed decision-making at both the classroom and institutional levels. Administrators can assess the efficacy of programs, initiatives, and policies, identify problem areas, and make informed decisions to improve the educational process.Customizable and Extensible
The Moodle Learning Analytics API allows for the creation of custom models based on reusable targets, indicators, and other components. This flexibility enables institutions to design models that align with their specific educational priorities.Disadvantages of Moodle Analytics
While Moodle Analytics offers numerous benefits, there are also some challenges and limitations to consider.Initial Configuration and Training
The system requires initial configuration and the training of machine-learning models on site data before they can make predictions. This can be time-consuming and may delay the implementation of analytics.Resource Requirements
Maintaining and updating Moodle Analytics can be resource-intensive. Institutions need to manage their own hosting, security, and updates, which can require substantial IT resources.Limited Descriptive Analytics
Built-in reports in Moodle are primarily descriptive, telling participants what happened but not why. Additional context and human judgment are often needed to interpret these reports and generate meaningful predictions or prescriptions.Security Considerations
As with any open-source system, Moodle Analytics can be more prone to security vulnerabilities if not properly managed. Ensuring the security of the platform is crucial to protect sensitive student data.User Interface and Learning Curve
While not specific to analytics, the overall Moodle platform can have a steep learning curve and an outdated user interface, which may affect the ease of use and engagement with the analytics tools. By understanding these advantages and disadvantages, educational institutions can make informed decisions about how to effectively implement and utilize Moodle Analytics to improve learning outcomes.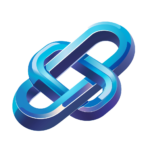
Moodle Analytics - Comparison with Competitors
When Comparing Moodle’s Analytics Capabilities
When comparing Moodle’s analytics capabilities with other education tools in the AI-driven category, several key features and alternatives stand out.
Moodle Analytics
Moodle offers a comprehensive analytics system, particularly enhanced by its use of xAPI and Caliper for experience tracking.
- AI Models for At-Risk Students: Moodle uses AI tools to identify students at risk, analyzing 42 parameters grouped into cognitive presence, social presence, and teacher presence. These models can be customized and visualized using various plugins.
- Customizable Reports and Plugins: Moodle allows for the creation of custom reports and graphs, and its open-source nature provides access to a wide range of free community-made analytics plugins. Additionally, Moodle’s Learning Analytics API enables predictive analytics.
- Built-in and Third-Party Integrations: Moodle’s core offers descriptive analytics, such as course completion and activity reports. Users can also install third-party plugins and Moodle Certified Integrations to enhance these capabilities.
Blackboard Analytics
Blackboard, another prominent Learning Management System (LMS), offers strong analytic tools, especially with the Blackboard Ultra plan.
- Straightforward Experience: Blackboard provides a more direct solution with strong analytic tools out of the box, including AI models to identify at-risk students based on missed deadlines, grades, course activity, and course access.
- Standalone Add-ons: Blackboard’s analytics are available as standalone add-ons, including modules for analyzing return on investment and tracking student performance.
Canvas LMS Analytics
Canvas LMS is another competitor with notable analytics features.
- Attendance and Performance Tracking: Canvas offers robust attendance tracking using features like “Roll Call” and seating charts. It also tracks performance and course completion, providing data on activities, submissions, and grades.
- Analytics Hub: Canvas has an integrated Analytics Hub that provides advanced settings for data visualization and AI-assisted insights into course effectiveness.
Other Alternatives
For those looking for alternative solutions beyond traditional LMS analytics, several AI-driven education tools are worth considering:
- Gradescope: An AI-assisted grading and assessment tool that offers analytics and rubric development. It is particularly useful for digital grading and assessment.
- Smart Sparrow: An adaptive e-learning platform that uses AI to personalize learning and provide real-time feedback. It is not an LMS but can be integrated to enhance learning analytics.
- Edmentum: An AI-powered learning platform that provides personalized learning experiences, assessing students’ strengths and weaknesses and offering targeted instruction and real-time feedback.
Key Differences
- Customization and Integration: Moodle stands out for its highly customizable reports and extensive plugin ecosystem, which can be both a strength and a complexity. Blackboard and Canvas offer more streamlined, out-of-the-box solutions.
- AI Capabilities: All three LMS platforms use AI to identify at-risk students, but Moodle’s flexibility in creating custom AI models is unique. Other tools like Gradescope and Edmentum focus more on specific aspects of learning analytics and personalization.
When choosing an LMS or analytics tool, it’s crucial to consider the specific needs of your organization, such as the level of customization required, the ease of use, and the particular analytics features that are most valuable.
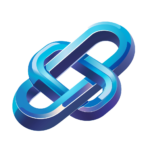
Moodle Analytics - Frequently Asked Questions
Frequently Asked Questions about Moodle Learning Analytics
Will I start getting predictions as soon as I enable the model?
When you enable a learning analytics model in Moodle, it does not immediately start generating predictions. The model must first be trained on your site’s historical data, which includes previous courses. This training process occurs in the background after the model is enabled. Once the model is trained, you will begin to receive predictions about current courses.Is there a way to export and import model data between sites?
Yes, you can export model data from one site and import it into another. This involves placing the exported model data into the model data directory of the new site. However, there is an ongoing effort to collect model data from multiple sites to create a shared training set for future Moodle installations.Is there a way to export and import the settings of a model between sites?
Currently, the functionality to export and import model settings between sites is in development. You can follow the progress on this feature through the Moodle issue tracker, specifically MDL-60944.How can I disable Moodle Learning Analytics notifications or restrict them to certain users?
To disable or restrict notifications, you need to manage the “analytics:listinsights” capability. By default, this capability is assigned to teachers and managers. You can modify the Teacher role to remove this capability or create a new role with this capability and assign it to specific individuals. This will control who receives the notifications.How accurate are the model predictions?
The accuracy of the model predictions depends on the quality and quantity of your site’s data. Factors such as the number of activities in each course and the percentage of the course conducted online in Moodle influence the accuracy. You can evaluate the model’s accuracy through the Analytics: Evaluate feature.How can I create my own Learning Analytics models?
To create your own learning analytics models, you currently need to implement them as Moodle plugins in PHP. You can refer to the Moodle Learning Analytics API for detailed instructions. There are ongoing efforts to develop tools that will allow non-programmers to create models as well.What types of analytics models are available in Moodle?
Moodle LMS comes with several core analytics models, including “Students at risk of dropping out,” “Upcoming activities due,” and “No teaching.” Additionally, you can install various plugins from the Moodle plugins directory to add more models. Certified integrations like IntelliBoard and LearnerScript also provide comprehensive learning analytics capabilities.How do analytics models work in Moodle?
Analytics models in Moodle combine indicators (predictors) and a target (the outcome being predicted) to generate insights (predictions). These models use machine learning algorithms to analyze site data and predict events such as students at risk of dropping out. The system can send notifications and suggest actions based on these predictions. The Analytics API allows for the definition of these models and the reuse of core indicators.Can I view and analyze previous predictions and evaluation logs?
Yes, you can view insights and predictions, including previous evaluation logs such as learning curve graphs and TensorBoard logs. This allows you to review the accuracy and effectiveness of the models over time.How often are predictions calculated, and what data is considered?
Predictions are calculated based on analysis intervals, which define when the system will calculate predictions and the portion of activity logs to consider. These intervals can be coded as PHP classes and are crucial for ensuring that predictions are timely and relevant, especially for models like “Students at risk of dropping out.” By addressing these questions, you can better understand how to implement, manage, and benefit from Moodle’s learning analytics capabilities.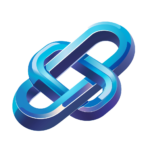
Moodle Analytics - Conclusion and Recommendation
Final Assessment of Moodle Analytics
Moodle Analytics is a powerful tool within the Moodle Learning Management System (LMS) that leverages data analytics to provide valuable insights into student behavior, performance, and engagement. Here’s a comprehensive overview of its benefits and who would most benefit from using it.
Key Features and Benefits
Predictive Models
Predictive Models: Moodle Analytics uses predictive models to detect trends and predict student behavior. These models combine indicators (predictors) and targets (the outcome being predicted) to generate insights and notifications. For example, the core analytics models include “Students at risk of dropping out,” “Upcoming activities due,” and “No teaching,” with the option to add more models via plugins.
Data-Driven Decision Making
Data-Driven Decision Making: Educators and administrators can make informed decisions by analyzing engagement metrics and performance data. This data-driven approach helps in identifying areas where students may struggle or disengage, allowing for adjustments in curriculum design, instructional materials, and resource allocation.
Personalized Learning
Personalized Learning: Moodle Analytics enables teachers to create individualized learning environments by analyzing student behavior patterns, learning preferences, and performance indicators. This helps in providing additional support to underperforming students and challenging high-achieving students with enrichment programs and advanced coursework.
Compliance and Integration
Compliance and Integration: The analytics system is fully integrated within Moodle, ensuring compliance with data protection regulations like GDPR. This integration eliminates the need to extract data and load it into other platforms, making it easier to manage and train machine learning algorithms.
Actionable Insights
Actionable Insights: The system generates notifications and suggested actions based on predictions. For instance, teachers can send messages to students at risk, view student activity reports, or implement intervention programs. This ensures that insights lead to tangible actions that improve learning outcomes.
Who Would Benefit Most
Educators
Educators: Teachers can benefit significantly from Moodle Analytics by gaining insights into student behavior and performance. This helps them in personalizing learning, identifying at-risk students, and implementing targeted interventions.
Administrators
Administrators: School and institutional administrators can use Moodle Analytics to assess the effectiveness of programs, initiatives, and policies. It helps in resource allocation, identifying problem areas, and making informed decisions to improve the educational process at an institutional level.
Students
Students: Ultimately, students benefit from a more engaging and inclusive learning environment. By identifying their needs and preferences, educators can provide the necessary support and challenges to ensure academic success.
Overall Recommendation
Moodle Analytics is a highly recommended tool for educational institutions looking to leverage data for improving learning outcomes. Its ability to provide predictive insights, support personalized learning, and facilitate data-driven decision making makes it an invaluable asset for both educators and administrators. The integration within the Moodle LMS ensures ease of use and compliance with data protection regulations, making it a practical and effective solution for enhancing the educational experience.