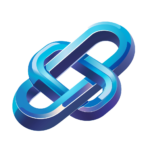
AnimeGAN2 - Detailed Review
Image Tools
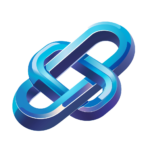
AnimeGAN2 - Product Overview
Introduction to AnimeGANv2
AnimeGANv2 is an advanced AI-driven tool in the image tools category, specifically designed to transform real-world photos and videos into high-quality anime-style images.Primary Function
The primary function of AnimeGANv2 is to convert photographs or videos of real-world scenes into anime-style images. This is achieved through a combination of neural style transfer and generative adversarial networks (GANs), making it a powerful tool for artistic style transfer.Target Audience
AnimeGANv2 is targeted at various groups, including:- Artists and animators looking to automate the process of creating anime-style images.
- Fans of anime who want to transform their photos or videos into anime-style art.
- Researchers and students in the field of computer vision and AI.
- Content creators who need to generate anime-style content efficiently.
Key Features
Improved Image Quality
AnimeGANv2 addresses a significant issue present in its predecessor, AnimeGAN, by preventing the generation of high-frequency artifacts in the images. This is achieved by using layer normalization instead of instance normalization, which helps maintain consistent feature properties across different channels in the feature map.Lightweight Model
The generator network in AnimeGANv2 is more efficient, with a parameter size of 8.6MB, compared to the 15.8MB of AnimeGAN. This reduction makes the model lighter and more manageable without compromising on the quality of the generated images.High-Quality Style Data
AnimeGANv2 uses high-quality style datasets derived from high-definition movie frames. These datasets include styles from renowned anime directors such as Hayao Miyazaki, Makoto Shinkai, and Kon Satoshi, ensuring that the generated images are of high visual quality.Versatile Usage
The tool allows for the conversion of both photos and videos into anime-style content. Users can run inference on photos, convert videos to anime style, and even train the model using specific datasets.Accessibility
AnimeGANv2 is open-source and available for non-commercial use, making it accessible for academic research, teaching, and personal projects. The repository includes detailed instructions for usage, training, and converting media files. In summary, AnimeGANv2 is a sophisticated AI tool that efficiently transforms real-world images and videos into high-quality anime-style content, catering to a wide range of users with its improved features and accessibility.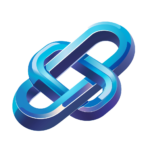
AnimeGAN2 - User Interface and Experience
User Interface
Intuitive Layout
- The interface typically includes a simple and intuitive layout where users can upload their photographs or videos. For example, in a web application setup, there is usually a clear section for image or video upload, along with options to select different anime styles (such as Hayao, Shinkai, or Paprika styles).
Style Selection
- Users can choose from multiple predefined anime styles, each emulating iconic aesthetics from renowned anime directors or films. This selection process is often straightforward, with buttons or dropdown menus to pick the desired style.
Output Display
- There are also buttons for submitting the image or video for conversion and a clear button to reset the input. The output section displays the converted anime-style image or video, allowing users to see the result immediately.
Ease of Use
User-Friendly Process
- AnimeGANv2 is relatively easy to use, even for those without extensive technical knowledge. The process involves uploading an image or video, selecting the desired anime style, and waiting for the conversion to complete. This simplicity makes it accessible to a wide range of users.
Guided Examples
- The application often includes example images or videos to demonstrate how the different styles work, which can help users make informed choices about the style they prefer.
Overall User Experience
Real-Time Feedback
- The overall user experience is enhanced by the real-time conversion capability, allowing users to see the results quickly. This immediate feedback is particularly beneficial for creative exploration and experimentation.
Secure Integration
- The integration of AnimeGANv2 into web applications ensures secure image uploading and efficient style conversion, which contributes to a positive user experience. Features like user authentication and instant style conversion make the process smooth and reliable.
User Satisfaction
- The feedback from user testing indicates high user satisfaction with the system’s usability and performance, suggesting that AnimeGANv2 effectively meets the needs of users looking to transform real-world photographs into anime-style artwork.
Conclusion
In summary, AnimeGANv2 offers a straightforward and efficient user interface that is easy to use, making it an excellent tool for anyone interested in converting images or videos into anime-style content.
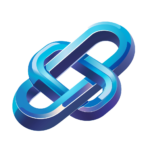
AnimeGAN2 - Key Features and Functionality
AnimeGANv2 Overview
AnimeGANv2 is an advanced AI-driven tool designed to transform real-world photos into high-quality anime images. Here are the key features and how they work:Lightweight Architecture
AnimeGANv2 is built as a lightweight generative adversarial model, which means it has fewer network parameters compared to its predecessor. The generator parameter size of AnimeGANv2 is 8.6MB, significantly less than the 15.8MB of the original AnimeGAN. This reduction in parameters makes the model more efficient and easier to deploy.Layer Normalization
One of the critical improvements in AnimeGANv2 is the use of layer normalization instead of instance normalization. Layer normalization helps prevent the generation of high-frequency artifacts in the images, which were a problem in the original AnimeGAN. This change ensures that the generated images have a more consistent and smooth visual quality.Network Structure
The generator network in AnimeGANv2 uses inverted residual blocks (IRBs) and depthwise separable convolutions, similar to its predecessor. However, the structure is optimized for better performance and efficiency. The network includes convolution blocks, up-sampling operations, and element-wise additions, which are crucial for maintaining the semantic content of the input images while transforming them into anime style.Loss Functions
AnimeGANv2 employs multiple loss functions to guide the generator to produce better animation visual effects. These include:Grayscale Style Loss
Ensures the grayscale version of the generated image matches the style of the target anime.Grayscale Adversarial Loss
Helps the generator produce images that are indistinguishable from real anime images in grayscale.Color Reconstruction Loss
Ensures the color of the generated image aligns well with the expected anime style.Content Loss
Uses high-level feature maps from a VGG network to preserve the semantic content of the input images.Training and Datasets
AnimeGANv2 is trained on newly established high-quality datasets derived from video frames of high-definition anime movies. The images used in training are 256×256 pixels, ensuring high-resolution output. The model can be trained with unpaired data, which simplifies the data collection process.Discriminator
The discriminator in AnimeGANv2 uses layer normalization instead of instance normalization, similar to the generator. This change helps in reducing high-frequency artifacts and improving the overall quality of the generated images.Efficiency and Performance
AnimeGANv2 is optimized for efficiency, allowing it to generate high-quality anime images quickly. The use of layer normalization and the reduced network parameters make it more efficient than the original AnimeGAN, while maintaining or even improving the visual quality of the generated images.Practical Application
AnimeGANv2 can be used to transform photos of real-world scenes into anime style images, which is valuable in various fields such as advertising, film, and children’s education. It automates the process of creating anime images, reducing the need for manual artistic skills and time-consuming manual creation.Conclusion
In summary, AnimeGANv2 integrates AI through advanced generative adversarial networks, optimized architecture, and carefully chosen loss functions to efficiently and effectively transform real-world photos into high-quality anime images.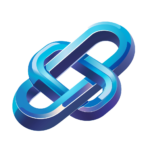
AnimeGAN2 - Performance and Accuracy
Performance of AnimeGANv2
AnimeGANv2, an advanced version of the AnimeGAN model, has shown significant improvements in transforming real-world photos into high-quality anime-style images.Image Quality
AnimeGANv2 generates images with better visual quality compared to its predecessor, AnimeGAN. This is achieved by changing the normalization method from instance normalization to layer normalization, which effectively prevents the generation of high-frequency artifacts.Evaluation Metrics
The model’s performance is evaluated through various metrics. For instance, AnimeGANv2 trained on a new high-quality dataset outperforms other models in terms of visual quality. However, specific quantitative metrics like Mean Opinion Score (MOS) or Frechet Inception Distance (FID) scores for AnimeGANv2 are not provided in the available sources. Instead, it is noted that AnimeGANv2 improves upon AnimeGAN, which itself has been compared to other models like NijiGAN and Scenimefy in different contexts.Style Transfer
AnimeGANv2 is capable of emulating distinct anime styles such as Hayao Style, Shinkai Style, and Paprika Style. The model uses powerful convolutional neural networks (CNNs) to extract high-level features and representations from input images, which are then manipulated to match the aesthetic attributes of each anime style.Architecture and Training
The architecture of AnimeGANv2 includes a generator network and a discriminator network, both composed of multiple convolutional layers. The discriminator effectively discerns real anime images from synthetic ones, ensuring high-quality anime-style artwork generation. The model undergoes iterative refinements during training, incorporating techniques such as edge-promoting adversarial loss and semantic content loss functions.Limitations and Areas for Improvement
High-Frequency Artifacts
Although AnimeGANv2 addresses the issue of high-frequency artifacts by using layer normalization instead of instance normalization, this change might slightly reduce the diversity of styles in the generated images. Instance normalization, while prone to artifacts, promotes different feature properties across channels, which can enhance style diversity.Dataset Dependence
The quality of the generated images is heavily dependent on the quality and diversity of the training dataset. Ensuring that the dataset is comprehensive and free of outliers is crucial for optimal performance. AnimeGANv2’s training dataset includes high-definition style images from video frames, which helps in achieving better results.Comparative Performance
While AnimeGANv2 improves over AnimeGAN, its performance relative to other state-of-the-art models like NijiGAN or StyleGAN2 is not directly compared in the available sources. For example, StyleGAN2 has been shown to achieve a significantly lower FID score (31) in generating anime faces, indicating superior performance in certain aspects.In summary, AnimeGANv2 demonstrates improved performance in generating high-quality anime-style images by addressing issues like high-frequency artifacts and using a more efficient network architecture. However, there is room for further improvement, particularly in comparing its performance against other advanced models and ensuring the diversity of generated styles.
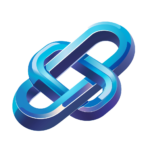
AnimeGAN2 - Pricing and Plans
The Pricing Structure for AnimeGANv2
The pricing structure for AnimeGANv2, an open-source project, is quite straightforward and differs significantly from commercial AI tools.
Free Usage
AnimeGANv2 is completely free to use. It is an open-source project, which means you can download and use it without any cost. Here are the key points:
- No Subscription Fees: There are no monthly or annual fees associated with using AnimeGANv2.
- Open-Source: The project is freely available for academic and non-academic entities for non-commercial purposes such as academic research, teaching, and scientific publications.
- Commercial Use: While it is free, commercial use requires obtaining authorization. You need to contact the developers for an authorization letter if you plan to use it for commercial purposes.
Features
Since AnimeGANv2 is free and open-source, the features are not tiered into different plans. Here are some of the key features you can expect:
- Image to Anime Conversion: Convert real-world photos into anime-style images.
- Video Conversion: Convert videos into anime-style videos.
- High-Resolution Images: Generate high-resolution anime images, though the quality may depend on the input image and training data.
- Custom Model Training: You can train custom models using your own dataset.
- Web Demo: There is also a web demo available for quick and easy use without the need for local installation.
Conclusion
AnimeGANv2 does not have a tiered pricing structure because it is free and open-source. It offers a range of features for converting images and videos into anime styles without any cost, making it accessible to anyone interested in using it.
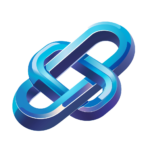
AnimeGAN2 - Integration and Compatibility
AnimeGANv2 Overview
AnimeGANv2, an advanced version of the AnimeGAN model, is designed to integrate seamlessly with various tools and platforms, ensuring broad compatibility and ease of use.
Platform Compatibility
- Google Colab: AnimeGANv2 can be easily run on Google Colab, a cloud-based platform, using a provided notebook. This allows users to transform photos into anime-style images without the need for local installations.
- PyTorch and TensorFlow: The model is available in both PyTorch and TensorFlow versions, making it versatile for different deep learning frameworks. The PyTorch version was released in February 2021, thanks to contributions from @bryandlee.
Software Requirements
- To run AnimeGANv2 locally, users need Python 3.6, TensorFlow-GPU 1.15.0, CUDA 10.0.130, and cuDNN 7.6.0. Additional dependencies include OpenCV, tqdm, numpy, glob, and argparse. For ONNX file execution, onnxruntime is required.
File Format Compatibility
- AnimeGANv2 supports various image formats such as JPG, PNG, and WebP. However, using JPG is recommended for optimal results.
Integration with Web Applications
- The model can be integrated into web applications, as demonstrated by research that incorporates user authentication, secure image uploading, and instant style conversion features. This integration allows for real-time image-to-anime conversion, enhancing user experience and usability.
Training and Deployment
- AnimeGANv2 can be trained using high-quality datasets derived from BD movies, which helps in generating better visual quality. The model’s generator network is optimized to be smaller (approximately 8.17 Mb), making it more efficient for deployment.
Cross-Device Compatibility
While the documentation does not explicitly mention cross-device compatibility, the use of cloud platforms like Google Colab and the support for various deep learning frameworks suggest that AnimeGANv2 can be adapted to run on different devices, including those with GPU capabilities.
Conclusion
In summary, AnimeGANv2 is highly compatible with various platforms, frameworks, and tools, making it a versatile and accessible solution for transforming photos into anime-style images.
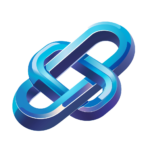
AnimeGAN2 - Customer Support and Resources
Support Options
- GitHub Issues and Pull Requests: The AnimeGANv2 repository on GitHub allows users to report issues, request features, and contribute to the project through the issues and pull requests sections. This is a direct way to engage with the developer community and get help with specific problems.
Additional Resources
Documentation and Tutorials
- The GitHub repository provides detailed documentation on how to set up, train, and use AnimeGANv2. This includes step-by-step instructions for inference, converting videos to anime, and training the model.
- There are also external tutorials, such as the step-by-step guide on installing and running AnimeGANv2 on Windows, which covers setting up the environment, installing dependencies, and converting images and videos.
Pretrained Models and Datasets
- The project offers pretrained models for different anime styles, such as Hayao, Shinkai, and Paprika, which can be downloaded and used directly. Additionally, there are high-quality datasets available for training, which include various anime styles and real-world images.
Community and Contributions
- Users can contribute to the development of AnimeGANv2 by checking out the project’s repository on GitHub, where they can find guidelines and issue tracking information. This community involvement helps in improving the model and addressing user needs.
License and Usage Terms
- The repository clearly outlines the license terms, which allow for non-commercial use such as academic research, teaching, and scientific publications. For commercial use, users need to contact the authors for authorization.
Web Demo
- For those who prefer a quick and easy way to use AnimeGANv2 without setting up a local environment, there is a web demo provided by Hugging Face. This allows users to generate anime images without the need for installations or setups.
These resources ensure that users have comprehensive support and the necessary tools to effectively use and contribute to AnimeGANv2.
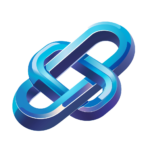
AnimeGAN2 - Pros and Cons
Advantages of AnimeGANv2
Improved Image Quality
AnimeGANv2 addresses the issue of high-frequency artifacts that were present in the original AnimeGAN model. By using layer normalization instead of instance normalization, it prevents the generation of localized noise and high-frequency artifacts, resulting in higher visual quality of the generated anime-style images.Efficient Network Architecture
The model features a more efficient generator network with reduced parameters. The generator parameter size of AnimeGANv2 is 8.6MB, which is significantly lower than the 15.8MB of the original AnimeGAN. This reduction makes the model more lightweight and easier to train.High-Quality Dataset
AnimeGANv2 is trained on a newly established high-quality dataset derived from high-definition video frames of iconic anime films. This dataset includes styles from renowned directors such as Miyazaki Hayao, Makoto Shinkai, and Kon Satoshi, ensuring that the generated images capture the distinctive characteristics of these styles.Effective Style Transfer
The model employs powerful convolutional neural network (CNN) architectures to extract high-level features from input images, which are then stylized to match the aesthetic attributes of different anime styles. This results in faithful and expressive transformations of real-world photographs into anime-style images.User-Friendly Implementation
AnimeGANv2 provides a straightforward implementation process, with tools and scripts available for inference, video conversion, and training. This makes it accessible for users to transform photos and videos into anime-style content without extensive technical expertise.Disadvantages of AnimeGANv2
Training Requirements
The model requires specific hardware and software configurations for optimal performance, such as a GPU (e.g., 2080Ti) and specific versions of TensorFlow and CUDA. This can be a barrier for users without access to such resources.Dataset Dependence
The quality and diversity of the generated images are heavily dependent on the quality and diversity of the training dataset. If the dataset lacks variety or has inconsistent brightness and tone, it can affect the overall quality of the generated images.Potential for Inconsistent Results
While the model is designed to handle various anime styles, the results can vary depending on the input images. For example, stylizing photos with people as the main subject may require additional training data to achieve optimal results.Limited Customization
While AnimeGANv2 supports multiple anime styles, the customization options for these styles are limited to the predefined datasets and styles included in the model. Users may not be able to easily create or customize new styles without retraining the model. In summary, AnimeGANv2 offers significant improvements in image quality, efficiency, and style transfer capabilities, but it also has specific requirements and limitations that users should be aware of.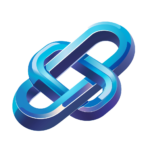
AnimeGAN2 - Comparison with Competitors
Unique Features of AnimeGANv2
- AnimeGANv2 is a lightweight generative adversarial model with fewer network parameters, making it easier to train and more efficient in terms of computational resources.
- It incorporates a total variation loss in the generator loss to solve the problem of high-frequency artifacts in the generated images, resulting in smoother and more natural-looking anime-style outputs.
- The model uses new high-quality style data, often sourced from BD movies, to improve the visual quality of the generated anime images.
Alternatives and Comparisons
DeOldify
- DeOldify is a deep learning project focused on colorizing and restoring old images and videos. While it doesn’t specifically target anime-style transformations, it can enhance and restore images, which might be a complementary tool in the workflow of creating anime-style images.
Animegan2-pytorch
- This is a PyTorch implementation of AnimeGANv2. It offers the same functionality as the original AnimeGANv2 but with the flexibility of using PyTorch, which can be beneficial for those already working within the PyTorch ecosystem.
Bringing-Old-Photos-Back-to-Life
- This project, presented at CVPR 2020, focuses on restoring old photos. While not specifically aimed at anime-style transformations, it can be useful for enhancing and restoring images before applying anime-style effects.
UGATIT (Selfie2Anime)
- UGATIT, also known as Selfie2Anime, is another tool for transforming selfies into anime-style images. However, AnimeGANv2 is noted for its superior quality and more natural anime-style outputs compared to UGATIT.
VansPortrait
- VansPortrait uses AnimeGANv2 among other algorithms to transform selfies into anime portraits. It offers a user-friendly interface and the ability to produce sketch or cartoon pictures in addition to anime-style images.
Other Notable Alternatives
Selfie2Waifu, TwinFace, Anime Faces AI
- These tools are specifically designed for transforming selfies into anime or manga-style images. They offer various features such as user-friendly interfaces and online accessibility, but may not match the quality and customization options provided by AnimeGANv2.
PaddleGAN
- PaddleGAN is a comprehensive GAN library that includes various applications like image style transfer, photo repair, and cartoon generation. While it is not specifically focused on anime-style transformations, it offers a broad range of GAN-based tools that could be adapted for such purposes.
Conclusion
In summary, AnimeGANv2 stands out due to its lightweight architecture, efficient training process, and high-quality anime-style outputs. However, depending on specific needs such as restoring old photos or using different frameworks (like PyTorch), the mentioned alternatives can be valuable tools in the broader context of image transformation and enhancement.
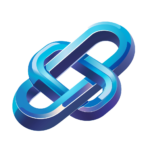
AnimeGAN2 - Frequently Asked Questions
Frequently Asked Questions about AnimeGANv2
Q: What is AnimeGANv2 and what does it do?
AnimeGANv2 is an improved version of the AnimeGAN model, which uses generative adversarial networks (GANs) to transform real-world photos into anime-style images. It enhances the visual quality of the generated images compared to its predecessor and reduces high-frequency artifacts.
Q: How does AnimeGANv2 improve over AnimeGAN?
AnimeGANv2 addresses several issues present in AnimeGAN, such as the generation of high-frequency artifacts. It achieves this by changing the normalization of features in the network and reducing the scale of the generator network. Additionally, it uses a new high-quality dataset derived from high-definition anime movies.
Q: What types of anime styles can AnimeGANv2 generate?
AnimeGANv2 can generate images in various iconic anime styles, including Miyazaki Hayao (e.g., from “The Wind Rises”), Makoto Shinkai (e.g., from “Your Name” and “Weathering with You”), and Kon Satoshi (e.g., from “Paprika”).
Q: What is the architecture of AnimeGANv2?
AnimeGANv2 employs a GAN framework consisting of a generator network and a discriminator network. The generator uses convolutional layers and activation functions to capture intricate details and characteristics of anime artwork. The discriminator evaluates the authenticity of generated images using multiple convolutional layers to analyze low-level and high-level features.
Q: What are the system requirements to run AnimeGANv2?
To run AnimeGANv2, you need Python 3.6, TensorFlow-GPU 1.15.0, a GPU (such as an NVIDIA 2080Ti), CUDA 10.0.130, cuDNN 7.6.0, OpenCV, and other libraries like NumPy and argparse.
Q: How do I use AnimeGANv2 to convert photos to anime-style images?
You can use AnimeGANv2 by running the test.py
script, specifying the checkpoint directory, test directory, and save directory. For example: python test.py --checkpoint_dir checkpoint/generator_Hayao_weight --test_dir dataset/test/HR_photo --save_dir Hayao/HR_photo
.
Q: Can AnimeGANv2 convert videos to anime-style videos?
Yes, AnimeGANv2 can convert videos to anime-style videos. You can use the video2anime.py
script, specifying the input video, checkpoint directory, and output directory. For example: python video2anime.py --video video/input/お花見.mp4 --checkpoint_dir checkpoint/generator_Hayao_weight --output video/output
.
Q: How do I train AnimeGANv2?
To train AnimeGANv2, you need to download the necessary datasets, perform edge smoothing, and then run the train.py
script. Detailed steps include downloading VGG19, the train/val photo dataset, and running the training script with specified parameters.
Q: Is AnimeGANv2 available for commercial use?
AnimeGANv2 is available for non-commercial purposes such as academic research and teaching. For commercial use, you need to contact the authors via email to obtain the necessary authorization.
Q: What is the license for using AnimeGANv2?
AnimeGANv2 is made freely available for non-commercial purposes under specific license terms. You must agree to these terms to use the model.
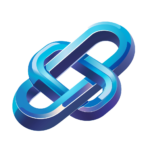
AnimeGAN2 - Conclusion and Recommendation
Final Assessment of AnimeGANv2
AnimeGANv2 is a significant advancement in the field of AI-driven image tools, particularly for transforming real-world photos into anime-style images. Here’s a comprehensive assessment of its benefits, target users, and overall recommendation.Key Improvements and Features
AnimeGANv2 addresses several critical issues present in its predecessor, AnimeGAN. The most notable improvements include:- Reduction of High-Frequency Artifacts: By using layer normalization instead of instance normalization, AnimeGANv2 effectively prevents the generation of high-frequency artifacts, resulting in smoother and more visually appealing anime-style images.
- Efficient Network Architecture: The generator network has been optimized to reduce the number of parameters, making the model more efficient and easier to train.
- High-Quality Dataset: The model is trained on a newly established high-quality dataset derived from high-definition anime movies, which enhances the visual quality of the generated images.
Target Users
AnimeGANv2 would be highly beneficial for several groups:- Graphic Designers and Artists: Those looking to transform real-world photos into anime-style images can leverage AnimeGANv2 for creative projects, saving time and effort.
- Marketing and Advertising Professionals: Given the broad appeal and cultural resonance of anime in Japan and globally, marketers can use AnimeGANv2 to create engaging anime-style content for their campaigns.
- Researchers and Students: The model’s improvements and the availability of its code on GitHub make it a valuable tool for academic research and educational purposes in the fields of computer vision and AI.
Usage and Practicality
- Ease of Use: The model comes with a TensorFlow implementation and even an online access project, allowing users to perform photo animation through a browser without extensive technical setup.
- Customization: Users can train the model with their own datasets, such as adding photos of people to improve the stylization of human subjects.