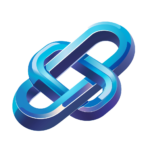
MiniGPT-4 - Detailed Review
Image Tools
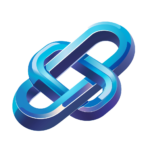
MiniGPT-4 - Product Overview
Introduction to MiniGPT-4
MiniGPT-4 is an open-source AI model that combines a visual encoder with a large language model (LLM) to enhance vision-language capabilities. This model is not officially connected to OpenAI or GPT-4 but is built on the Vicuna LLM, which is itself based on the open-source Large Language Model Meta AI (LLaMA).
Primary Function
The primary function of MiniGPT-4 is to process and generate outputs based on both images and text. It can describe images, answer questions about image content, generate stories and poems inspired by images, and even create websites from hand-written drafts.
Target Audience
MiniGPT-4 is versatile and can benefit various audiences, including:
- Educators: It can help in teaching and learning by generating detailed explanations and stories based on images.
- Healthcare Professionals: It can aid in diagnostics, treatment planning, and patient education by analyzing medical images.
- Marketing and Advertising Professionals: It can create engaging content such as stories and poems based on images.
- General Users: It can assist in tasks like generating recipes from food photos and providing cooking instructions.
Key Features
- Detailed Image Description Generation: MiniGPT-4 can provide comprehensive descriptions of images, helping users understand the content and context of the visuals.
- Website Creation from Hand-Written Drafts: It can generate an entire website by analyzing an image of a hand-written draft.
- Creative Content Generation: It can write stories, poems, and other creative content inspired by given images.
- Problem-Solving: It can analyze images containing problems or challenges and generate solutions.
- Culinary Assistance: It can provide recipes and cooking instructions based on images of food.
Technical Details
MiniGPT-4 aligns a frozen visual encoder from BLIP-2 with a frozen LLM, Vicuna, using a single projection layer. This alignment allows the model to exhibit many capabilities similar to those of GPT-4. The model is fine-tuned using a conversational template, which improves its language output and overall usability.
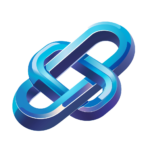
MiniGPT-4 - User Interface and Experience
Interface Overview
The MiniGPT-4 demo, accessible through the official website, provides a straightforward and easy-to-use interface. Here, users can interact with the model by uploading images and entering prompts to generate various types of responses.
Key Features
- Image Upload: Users can upload images directly to the platform. This could be anything from a scenic beach to a handwritten draft or a complex diagram.
- Prompt Input: After uploading an image, users can enter a prompt to guide the model’s response. For example, “Describe the beach scene in the image” or “Create a website layout based on the handwritten draft”.
- Response Generation: Once the image and prompt are submitted, the model generates a response. This could be a detailed description of the image, answers to questions about the image, or even the generation of HTML and CSS code for a website based on a handwritten draft.
Ease of Use
The interface is relatively simple to use, even for those without extensive technical background. Here are some key points:
- User-Friendly Interface: The demo page is laid out in a clear and accessible manner, allowing users to easily upload images and enter prompts.
- Step-by-Step Process: The process involves selecting a task, uploading an image, entering a prompt, and then generating the output. This step-by-step approach makes it easy for first-time users to get started.
Overall User Experience
The overall user experience is positive due to several factors:
- Fast Response Times: MiniGPT-4 is known for its efficiency, with average response times under 8 seconds, which enhances the user experience by providing quick and relevant responses.
- Coherent and Detailed Responses: The model generates coherent and detailed responses, whether it’s describing an image, answering questions, or generating text based on the image. This makes the interaction feel natural and helpful.
- Versatility: The model can handle a variety of tasks, from describing historical monuments to generating website layouts from handwritten drafts, which keeps the user engaged and interested in exploring its capabilities.
In summary, the user interface of MiniGPT-4 is designed to be easy to use, with a clear and intuitive layout that allows users to quickly and effectively interact with the model to achieve their desired outcomes.
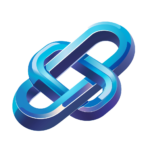
MiniGPT-4 - Key Features and Functionality
MiniGPT-4 Overview
MiniGPT-4 is a sophisticated AI model that integrates vision and language capabilities, making it a versatile tool for various applications. Here are the main features and how they work:Multimodal Capabilities
MiniGPT-4 combines a frozen visual encoder, specifically BLIP-2, with a large language model (LLM) called Vicuna. This integration allows the model to process and generate text based on images. For instance, it can describe images, answer questions about them, and even generate text that continues a conversation about the image.Image Description and Question Answering
The model can look at an image and generate a detailed text description of what is in the image. It can also answer questions about the image, providing coherent and relevant responses. This is achieved through the alignment of the visual encoder and the LLM, enabling the model to comprehend both visual and textual data.Text Generation
MiniGPT-4 can generate text based on an image, including creating stories, poems, and even providing instructions. For example, it can write stories or poems inspired by given images or teach users how to cook based on food photos.Efficient Training
The model uses a two-stage training approach. The first stage aligns the visual and language models using a large dataset, while the second stage fine-tunes the model using a smaller, high-quality dataset. This approach allows for quick training, with the second stage taking only about 7 minutes on a single A100 GPU.Alignment Technique
MiniGPT-4 employs a novel alignment technique using a single projection layer to connect the visual encoder and the LLM. This method ensures high efficiency and low computational cost, making the model highly practical for various applications.Quality-Focused Dataset Fine-tuning
The model is fine-tuned using a high-quality, well-aligned dataset to ensure coherent and natural language generation. This step is crucial for enhancing the model’s reliability and usability, as it helps avoid issues like repetition and fragmented sentences in the output.Use Cases
Creative Writing Assistance
MiniGPT-4 can inspire and aid in the creation of stories and poetry based on images.Problem-Solving
The model can offer solutions to problems presented in images, which is useful for educational and professional purposes.Culinary Guidance
It can teach users how to cook based on food photography.Visual Search and Image Retrieval
MiniGPT-4 is also useful for tasks like image captioning, visual question answering, and image retrieval.Input Requirements
To use MiniGPT-4, you need to prepare your input data in a specific format, which includes image-text pairs. The images are processed by the visual encoder, and the text is processed by the LLM. Here is an example of the input format:{ "image": "image.jpg", "text": }This structured input allows the model to effectively process and generate relevant outputs.
Conclusion
Overall, MiniGPT-4’s integration of vision and language capabilities, combined with its efficient training and high-quality dataset fine-tuning, makes it a valuable tool for a wide range of applications.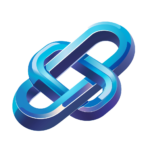
MiniGPT-4 - Performance and Accuracy
When Evaluating MiniGPT-4
When evaluating the performance and accuracy of MiniGPT-4 in the image tools AI-driven product category, several key points stand out:
Training and Fine-Tuning
MiniGPT-4 undergoes a two-stage training process. The first stage involves pretraining with roughly 5 million aligned image-text pairs, which allows the model to develop basic vision-language capabilities. However, this initial stage can impact the model’s ability to generate fluent and natural language outputs.
The second stage is a fine-tuning process using a smaller but high-quality dataset of image-text pairs. This dataset, often around 3,500 pairs, is curated to enhance the model’s generation reliability and usability. This fine-tuning stage is computationally efficient, taking only about 7-10 minutes with a single A100 GPU.
Performance Metrics
MiniGPT-4 demonstrates significant improvements in various vision-language tasks after the fine-tuning stage. Here are some key performance metrics:
- Image Description and Captioning: MiniGPT-4 can generate detailed and coherent descriptions of images, outperforming some existing models in this task.
- Multimodal Tasks: In evaluations such as MME, MMBench, and VQA datasets, fine-tuned versions of MiniGPT-4, like InstructionGPT-4, show better performance. For instance, InstructionGPT-4 outperforms the original MiniGPT-4 with a 23 score enhancement on MME, a 1.55 score improvement on MMBench, and a 1.76% increase in performance on VQA datasets.
Limitations and Areas for Improvement
Despite its advancements, MiniGPT-4 has some limitations:
- Hallucinations: The model can sometimes generate descriptions that include non-existent elements in the image, such as hallucinating unexisting tablecloths or incorrectly locating windows.
- Data Quality: The quality of the fine-tuning dataset is crucial. Using high-quality but fewer instruction data points can significantly improve performance, but low-quality data can hinder it.
- Specific Tasks: While MiniGPT-4 performs well in many vision-language tasks, it may still struggle with certain specific tasks or require further fine-tuning for optimal performance in those areas.
Engagement and Factual Accuracy
MiniGPT-4 is capable of engaging users by generating detailed and natural language outputs about images. However, ensuring factual accuracy is essential, especially since the model can sometimes generate incorrect details. The fine-tuning process helps in improving this aspect, but continuous monitoring and refinement of the model are necessary to maintain high accuracy.
In summary, MiniGPT-4 shows promising performance in the image tools AI-driven product category, particularly after the fine-tuning stage. However, it is important to address its limitations, such as hallucinations and the need for high-quality training data, to further enhance its accuracy and reliability.
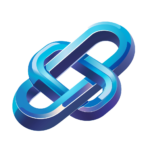
MiniGPT-4 - Pricing and Plans
Pricing
- Input Tokens: $0.15 per million tokens.
- Output Tokens: $0.60 per million tokens.
Plans and Features
While the specific website for MiniGPT-4 does not provide detailed pricing plans, here is how GPT-4o mini fits into OpenAI’s overall pricing structure:Free Access
- You can access GPT-4o mini for free through certain platforms like Merlin AI, which offers a limited number of queries for free. For example, Merlin AI provides 102 free queries for GPT-4o mini.
Paid Plans
- OpenAI Plans:
- Free Plan: Limited access to GPT-4o mini, with restrictions on usage.
- Plus Plan: $20 per month, includes extended limits on messaging, file uploads, and other features, but still limited access to GPT-4o mini.
- Pro Plan: $200 per month, offers unlimited access to GPT-4o mini along with other advanced features.
- Team Plan: $25-30 per user per month (billed annually or monthly), provides higher message limits and expanded access to GPT-4o mini.
- Enterprise Plan: Custom pricing, includes high-speed access to GPT-4o mini, expanded context window, and enterprise-grade features.
Key Features
- GPT-4o mini:
- Supports text and vision inputs.
- Excels in textual and multimodal reasoning.
- Has a 128K context length.
- Significantly cheaper than previous models like GPT-3.5 Turbo.
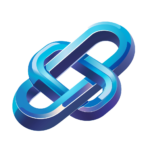
MiniGPT-4 - Integration and Compatibility
Integration with Other Tools
MiniGPT-4, as a vision-language model, is designed to be versatile and integrable with various tools and systems. Here are some key points on its integration:
Dataset Creation and Alignment
MiniGPT-4 uses a two-stage training approach, where the first stage aligns a frozen visual encoder from BLIP-2 with a frozen Large Language Model (LLM), Vicuna, using just one projection layer. This alignment is done using large datasets like Laion and CC datasets, and later fine-tuned with a smaller, high-quality dataset created by the model itself and ChatGPT.
Compatibility with AI Frameworks
MiniGPT-4 can be integrated into AI frameworks that support PyTorch, as it is trained and deployed using PyTorch commands. For example, the training scripts provided use `torchrun` commands to launch the training processes.
Compatibility Across Different Platforms and Devices
MiniGPT-4 shows promising compatibility across various platforms and devices:
NVIDIA GPUs
The model is optimized for training on NVIDIA GPUs, specifically A100s. The first pretraining stage uses 4 A100s and takes about 10 hours, while the second finetuning stage uses just 1 A100 and takes only 7 minutes.
NVIDIA Jetson AGX Orin
MiniGPT-4 can be deployed on the NVIDIA Jetson AGX Orin edge device, allowing for local and secure inferencing independent of network limitations. This makes it suitable for edge computing applications.
General Hardware
While the model is optimized for high-performance GPUs, it can theoretically be run on other hardware configurations, though performance may vary. However, specific instructions are provided for deployment on Jetson devices, indicating a focus on edge computing capabilities.
Deployment and Usage
For practical use, MiniGPT-4 can be accessed and used in several ways:
Web Interface
Users can interact with MiniGPT-4 through a web interface where they can upload images and receive text descriptions or answers to questions about the images.
Local Deployment
Developers can deploy MiniGPT-4 locally on their own servers or edge devices, following the provided setup instructions. This allows for customized integration into various applications.
Overall, MiniGPT-4 is engineered to be adaptable and efficient, making it a valuable tool for integrating vision-language capabilities into a wide range of applications and platforms.
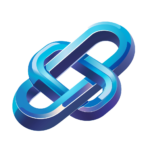
MiniGPT-4 - Customer Support and Resources
Customer Support Options for MiniGPT-4
When considering the customer support options and additional resources for MiniGPT-4, it’s important to note that MiniGPT-4 is primarily a technical tool focused on vision-language understanding, rather than a consumer product with traditional customer support.Documentation and Guides
The primary resources for MiniGPT-4 are found in its GitHub repository and associated documentation. Here, users can access comprehensive guides on how to install, set up, and use the model. This includes step-by-step instructions on cloning the repository, installing dependencies, downloading pretrained weights, and running sample code.Community Support
Since MiniGPT-4 is an open-source project, much of the support comes from the community. Users can engage with other developers and users through issues and discussions on the GitHub repository. This community-driven approach can be helpful for troubleshooting and sharing knowledge.Online Demo
For those who want to explore the capabilities of MiniGPT-4 without coding, there is an online demo available. This demo allows users to upload images, enter prompts, and see the model’s responses in action. This can be a useful resource for understanding what the model can do before deciding to use it.Fine-Tuning and Customization
The model provides resources for fine-tuning, which can help users make the model more reliable and user-friendly for their specific needs. This includes using conversational templates to improve language generation and coherence.Educational Resources
MiniGPT-4 can be particularly useful for educational purposes, such as explaining complex concepts based on diagrams or images. The model can generate detailed and coherent explanations, making it a valuable tool for both students and teachers.Conclusion
In summary, while MiniGPT-4 does not offer traditional customer support like many commercial products, it provides extensive documentation, community support, and resources for fine-tuning and customization, making it a powerful tool for those willing to engage with its technical aspects.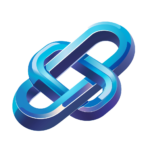
MiniGPT-4 - Pros and Cons
Advantages of MiniGPT-4
Efficiency and Computational Resources
MiniGPT-4 is highly computationally efficient, which is a significant advantage. It achieves this through limited training requirements, where only the linear projection layer needs to be trained, reducing the need for extensive computational resources. This model can be trained in less than 24 hours on a standard GPU.Optimized Data Use
The model is trained on approximately 5 million aligned image-text pairs, which is a large but optimized dataset. This ensures effective learning without excessive computational power.Streamlined Architecture
MiniGPT-4 uses a single linear projection layer to connect the visual encoder and the language model, simplifying data flow and reducing processing time. This architecture aligns a frozen visual encoder with a frozen large language model (LLM) called Vicuna, making it lightweight and efficient.Multimodal Capabilities
MiniGPT-4 can handle both visual and textual inputs, enabling it to generate detailed image descriptions, write stories based on images, create websites from hand-drawn UIs, and even generate recipes from food images. It can also provide advice and insights based on visual information.Improved Language Generation
Initially, the model faced issues with generating unnatural language outputs. However, through the use of high-quality datasets and conversational templates during fine-tuning, the language outputs have become more natural and coherent.Disadvantages of MiniGPT-4
Speed and Responsiveness
One of the main limitations is the speed of the model. Even with high-end GPUs, the model can be slow, particularly for users without powerful discrete GPUs. This can make the experience feel unresponsive compared to cloud-based AI tools.Hallucinations and Misinterpretations
Like other AI chatbots, MiniGPT-4 can “hallucinate” or make up information, which can lead to inaccurate outputs. It may also misinterpret certain elements in complex images.Limited Visual Perception
The model has limited visual perception and may struggle to recognize detailed textual information in images. It can encounter challenges in understanding complex images and may misinterpret certain elements.Inference Time
Despite its efficiency in training, the model’s inference can still be slow, even on high-end GPUs, which can result in slow response times. In summary, MiniGPT-4 offers significant advantages in terms of efficiency, multimodal capabilities, and improved language generation, but it also faces challenges related to speed, accuracy, and visual perception limitations.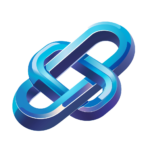
MiniGPT-4 - Comparison with Competitors
When comparing MiniGPT-4 to other AI-driven image tools, several unique features and potential alternatives stand out.
Unique Features of MiniGPT-4
MiniGPT-4 distinguishes itself through its advanced multimodal capabilities, combining both vision and language understanding. Here are some of its key features:Multimodal Processing
MiniGPT-4 can describe images, answer questions about them, and generate text based on the visual content. It uses a combination of Vicuna, a large language model, and BLIP-2, a visual encoder, connected by a single linear projection layer.Efficient Training
The model requires minimal training time, with the ability to fine-tune in just 7 minutes on a single A100 GPU. This efficiency is due to its streamlined architecture and optimized data use.Detailed Image Descriptions
MiniGPT-4 can generate vivid and detailed descriptions of images, capturing visual elements and even the mood of the scene.Potential Alternatives
While MiniGPT-4 is highly capable, there are other AI image tools with their own strengths:DALL-E 3
This model excels in generating images from text prompts but can struggle with changing perspectives. It is accessible via Bing Chat and Microsoft Image Creator. Unlike MiniGPT-4, DALL-E 3 focuses more on generating images rather than describing them.Midjourney
Known for producing high-resolution images, Midjourney has long loading times and is no longer offering free images. It is more geared towards generating images rather than describing them.Adobe Firefly
This tool offers unique features like adjusting camera angles and has 100 monthly generative credits. However, it does not have the same level of multimodal capabilities as MiniGPT-4.Stable Diffusion
This model allows for generating images based on text prompts and has a prompt database. However, it suffers from long loading times and is not optimized for image description tasks.Key Differences
Multimodal Capabilities
MiniGPT-4 stands out for its ability to interpret and generate text based on images, a feature that is not as prominent in other models like DALL-E 3 or Midjourney.Efficiency and Speed
MiniGPT-4’s quick training and response times make it more efficient compared to models like Stable Diffusion or Midjourney, which have longer loading times.Application Focus
While other models are primarily focused on generating images from text, MiniGPT-4 is unique in its ability to describe images and engage in conversations about them. In summary, MiniGPT-4 offers a unique blend of vision and language processing that sets it apart from other AI image tools. However, depending on your specific needs, such as generating high-resolution images or adjusting camera angles, other models like Midjourney or Adobe Firefly might be more suitable alternatives.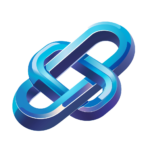
MiniGPT-4 - Frequently Asked Questions
What is MiniGPT-4?
MiniGPT-4 is an AI model that combines vision and language understanding. It uses a combination of a visual encoder and a large language model (LLM) called Vicuna to process and generate human-like text based on images.
How does MiniGPT-4 work?
MiniGPT-4 works by aligning a frozen visual encoder with a frozen LLM, Vicuna, using a single linear projection layer. The visual encoder converts images into a format the language model can understand, and the projection layer aligns the visual features with the language model.
What are the core components of MiniGPT-4?
The core components of MiniGPT-4 include a frozen visual encoder (responsible for understanding visual data), a Vicuna large language model (for natural language processing), and a single linear projection layer that connects these two components.
What capabilities does MiniGPT-4 have?
MiniGPT-4 can describe images, answer questions about images, generate text based on images, and even continue conversations about the images. It can also identify objects, describe actions, provide contextual information, write stories and poems inspired by images, and more.
How was MiniGPT-4 trained?
MiniGPT-4 was trained in two stages: pretraining on a large dataset of image-text pairs to align the visual and language models, and fine-tuning on a smaller, high-quality dataset using a conversational template to improve its performance and coherence.
How efficient is MiniGPT-4 in terms of training and response time?
MiniGPT-4 is highly efficient. It only requires training the linear projection layer, which can be done in less than 24 hours on a standard GPU. The fine-tuning stage takes around 7 minutes on a single A100 GPU. The average response time is under 8 seconds.
Can MiniGPT-4 be used for educational purposes?
Yes, MiniGPT-4 can be very useful for educational purposes. It can provide detailed and coherent explanations based on diagrams or images, making it a valuable tool for students and teachers alike.
How do I get started with MiniGPT-4?
You can start by exploring the online demo, which provides a user-friendly interface to upload images and enter prompts. For more advanced use, you can refer to the GitHub repository for comprehensive documentation and code examples.
What kind of data does MiniGPT-4 use for training?
MiniGPT-4 is trained on approximately 5 million aligned image-text pairs. This large but optimized dataset ensures the model learns effectively without requiring excessive computational power.
What are the potential applications of MiniGPT-4?
MiniGPT-4 has a wide range of potential applications, including web development, content creation, education, and more. Its unique blend of visual and language processing capabilities makes it a valuable asset for various industries.
Is MiniGPT-4 open source?
Yes, MiniGPT-4 is presented as an open-source alternative to other vision-language models like GPT-4, making it accessible for developers and researchers to use and build upon.
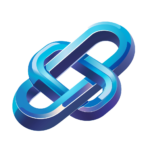