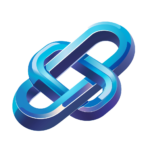
Segment Anything Model (SAM) by Meta AI - Detailed Review
Image Tools
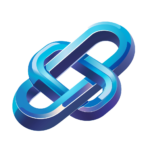
Segment Anything Model (SAM) by Meta AI - Product Overview
Introduction to Segment Anything Model (SAM)
The Segment Anything Model (SAM) developed by Meta AI is a revolutionary tool in the image tools AI-driven product category, specifically focused on image segmentation.Primary Function
SAM’s primary function is to accurately locate and segment objects or regions within images. This is achieved through a promptable segmentation task, where the model can be guided by various inputs such as clicks, boxes, text, or other spatial or textual clues to identify specific objects or areas in an image.Target Audience
SAM is designed to be accessible to a broad range of users, including but not limited to:- Researchers in computer vision and related fields
- Developers working on image segmentation tasks
- Professionals in content creation, such as video editors and graphic designers
- Industries like e-commerce, augmented reality (AR), and virtual reality (VR)
- Scientists conducting image analysis in various fields
Key Features
Architecture
SAM’s architecture consists of three main components: the image encoder, prompt encoder, and mask decoder. The image encoder processes input images into a dense feature matrix using a transformer-based approach, similar to those in advanced NLP models. The prompt encoder handles the input prompts, and the mask decoder generates the segmentation masks based on the combined information.Zero-Shot Transfer
SAM is capable of zero-shot transfer, meaning it can generalize to new tasks and image domains without the need for custom data annotation or extensive retraining. This is made possible by its training on a massive dataset of over 1 billion segmentation masks.Real-Time Interaction
The model is designed for real-time interaction, which is crucial for applications like AR/VR where immediate feedback is necessary. This real-time capability also benefits content creation tasks that require rapid segmentation.Multimodal Understanding
SAM can be integrated into larger AI systems for multimodal understanding, allowing it to interpret both text and visual content. This makes it versatile for various applications, including web page analysis and interactive systems.Efficient Data Annotation
SAM accelerates the creation of large-scale datasets by reducing the time and resources required for manual data annotation. This is achieved through its “data engine” approach, which iteratively uses the model to aid in data collection and enhance its performance.Versatile Use Cases
SAM has a wide range of applications, including:- Content creation: automating object extraction for collages or video editing
- E-commerce: segmenting and presenting new fashion items without specific model training
- Scientific research: locating and tracking objects in videos
- AR/VR: enabling object selection and transformation.
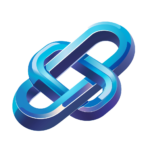
Segment Anything Model (SAM) by Meta AI - User Interface and Experience
The Segment Anything Model (SAM) by Meta AI
The Segment Anything Model (SAM) by Meta AI is characterized by a user-friendly and highly interactive interface, making image segmentation accessible and efficient for a wide range of users.
Promptable Interface
SAM features a “promptable interface” that allows users to provide inputs in various forms, such as clicks, bounding boxes, or freeform text. This versatility enables users to specify the segmentation task according to their needs, making the process highly adaptable and flexible.
Interactive Segmentation
The model supports interactive segmentation, allowing users to provide real-time guidance to refine segmentation masks. Users can interactively click on points to include or exclude from the object, draw bounding boxes, or provide textual descriptions. This interactive capability makes the segmentation process more intuitive and user-friendly.
Real-Time Processing
SAM is designed for real-time processing, generating segmentation masks swiftly, typically within 50 milliseconds after precomputing the image embedding. This real-time capability enables immediate feedback, which is crucial for applications such as augmented reality and content creation tasks that require rapid segmentation.
User-Friendly Interaction
The model’s task design accounts for cases where prompts might be ambiguous, potentially referring to multiple objects. Despite this ambiguity, SAM aims to generate a reasonable mask for one of the possible interpretations, ensuring usability even in challenging scenarios. This adaptability to ambiguity enhances the overall user experience by reducing the need for precise or complex inputs.
Efficiency and Accessibility
SAM’s architecture allows it to operate efficiently on a CPU in a web browser, making it accessible for real-time interaction without the need for specialized hardware. This efficiency is a critical factor in SAM’s usability, as it enables users to interact with the model seamlessly in various environments.
Simplified Data Annotation
SAM significantly simplifies the data annotation process, a task that is often time-consuming and resource-intensive. By automating the segmentation process, SAM allows users to focus on other critical data tasks such as mapping relationships between objects, assigning attributes, and evaluating the training data for balance, diversity, and bias.
Conclusion
Overall, the user interface of SAM is designed to be intuitive, flexible, and highly efficient, making image segmentation a straightforward and accessible task for a broad range of users and applications.
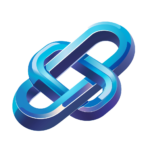
Segment Anything Model (SAM) by Meta AI - Key Features and Functionality
The Segment Anything Model (SAM) by Meta AI
The Segment Anything Model (SAM) by Meta AI is a revolutionary image segmentation model that offers several key features and functionalities, making it a versatile and powerful tool in the field of computer vision.
Promptable Segmentation Task
SAM is built around a promptable segmentation task, allowing users to generate segmentation masks using various prompts such as clicks, boxes, or freeform text. This flexibility enables users to specify the segmentation task according to their needs, making the model highly adaptable to different scenarios.
Advanced Architecture
The model’s architecture consists of three main components:
- Image Encoder: This component processes and transforms input images into a comprehensive set of features using a transformer-based approach, similar to those in advanced NLP models. It compresses images into a dense feature matrix that serves as the foundation for identifying various image elements.
- Prompt Encoder: A lightweight prompt encoder converts user prompts into embedding vectors in real time. This encoder interprets various prompt formats and converts them into a format the model can understand.
- Mask Decoder: The lightweight mask decoder predicts segmentation masks by combining information from both the image embedding and the prompt embedding. This decoder is responsible for generating accurate masks that identify the object or region specified by the user.
SA-1B Dataset
SAM is trained on the expansive SA-1B dataset, which contains over 1 billion segmentation masks spread across 11 million diverse, high-resolution images. This dataset is the largest segmentation dataset to date and provides SAM with a vast and diverse training data source, enabling it to generalize to new tasks and image domains without extensive retraining.
Zero-Shot Performance
One of the standout features of SAM is its zero-shot performance. The model can accurately segment images without prior specific training on those tasks, similar to how foundation models in NLP generalize across various tasks. This capability makes SAM a ready-to-use tool for diverse applications with minimal need for prompt engineering.
Real-Time Interaction
SAM supports real-time interaction, allowing users to provide real-time guidance to refine masks. After precomputing the image embedding, SAM can generate segmentation masks swiftly, typically in just 50 milliseconds. This real-time processing capability is crucial for applications like augmented reality and content creation tasks that require rapid segmentation.
Interactive Segmentation
The model supports interactive segmentation, enabling users to interactively click on points to include or exclude from the object, draw bounding boxes, or provide textual descriptions. This interactive feature makes the segmentation process more intuitive and user-friendly.
Adaptive to Ambiguity
SAM’s task design accounts for cases where prompts might be ambiguous, potentially referring to multiple objects. Despite this ambiguity, SAM aims to generate a reasonable mask for one of the possible interpretations, ensuring usability even in challenging scenarios.
Multimodal Understanding
SAM’s promptable segmentation can be integrated into larger AI systems for more comprehensive multimodal understanding, such as interpreting both text and visual content on webpages. This integration enhances the model’s ability to handle complex tasks that involve multiple forms of data.
Efficient Data Annotation
SAM’s data engine accelerates the creation of large-scale datasets by reducing the time and resources required for manual data annotation. The model assists in generating segmentation masks, which can then be refined or supplemented by human annotators, making the annotation process more efficient.
Applications
SAM’s versatility and real-time capabilities make it valuable for various industries, including:
- Content Creation: Automating object extraction for collages or video editing.
- Augmented Reality (AR) and Virtual Reality (VR): Enabling object selection and transformation.
- Scientific Research: Locating and tracking objects in videos to study phenomena.
- Fashion Retail: Instantly segmenting and presenting new fashion items without specific model training.
Conclusion
In summary, SAM’s unique architecture, zero-shot performance, real-time interaction, and integration with a massive dataset make it a powerful and adaptable tool for a wide range of image segmentation tasks and applications.
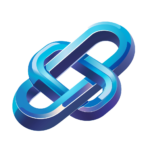
Segment Anything Model (SAM) by Meta AI - Performance and Accuracy
The Segment Anything Model (SAM) by Meta AI
The Segment Anything Model (SAM) by Meta AI is a significant advancement in image segmentation, offering impressive performance and accuracy in various scenarios. Here are some key points regarding its performance, accuracy, and limitations:
Performance and Accuracy
SAM is built on a vast dataset, the SA-1B dataset, which includes over 11 million images and 1 billion masks. This extensive training data enables SAM to achieve state-of-the-art performance in image segmentation tasks. It can accurately locate and segment specific objects or all objects within an image, often surpassing fully supervised models in terms of accuracy.
SAM’s architecture allows for zero-shot transfer, meaning it can adapt to new image distributions and tasks without prior knowledge. This capability makes it highly efficient and adaptable, especially in real-time applications where it can generate segmentation masks in as little as 50 milliseconds.
Precision and Efficiency
SAM stands out for its precision and efficiency. It uses prompt engineering and self-supervised learning to create detailed segmentation masks with minimal human intervention. This approach reduces the need for manual annotation, which is time-consuming and prone to human error. SAM’s accuracy is particularly notable in handling diverse data types and complex images, where traditional segmentation tools often struggle.
Limitations
Despite its impressive capabilities, SAM has several limitations:
- Fine-Grained Details: SAM struggles with very detailed parts and exact object edges. It may not capture small objects or precise boundaries as accurately as needed in certain applications, such as medicine or environmental monitoring.
- Tree-Like Structures and Low Textural Contrast: SAM can misinterpret objects with dense, tree-like structures or those with low textural contrast from their surroundings, leading to over-segmentation or failure to differentiate objects from the background.
- Object Tracking in Videos: SAM 2, the latest version, can lose track of objects across drastic camera viewpoint changes, long occlusions, or in crowded scenes. This issue can be mitigated with manual intervention and correction clicks.
- Multiple Object Segmentation: While SAM 2 can segment multiple objects simultaneously, its efficiency decreases significantly when handling multiple objects. It processes each object separately without inter-object communication, which can impact performance.
- Small Objects: SAM has difficulty detecting very small objects, such as plankton or lipid sacs, which can be a significant issue in fields like healthcare or environmental science.
Areas for Improvement
To further enhance SAM’s performance, several areas need attention:
- Boundary Details: Improving SAM’s ability to capture detailed edges and boundaries is crucial for applications requiring high precision.
- Handling Small Objects: Enhancing SAM’s capability to detect and segment very small objects will expand its utility in various fields.
- Video Segmentation: Improving SAM 2’s ability to track objects across different frames and handle complex video scenarios will make it more reliable for video analysis.
- Inter-Object Communication: Incorporating shared object-level contextual information could improve the efficiency of segmenting multiple objects simultaneously.
In summary, SAM is a powerful tool for image segmentation, offering high accuracy and efficiency. However, it has specific limitations that need to be addressed to make it more versatile and accurate in all scenarios.
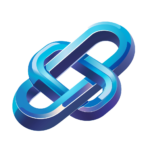
Segment Anything Model (SAM) by Meta AI - Pricing and Plans
The Segment Anything Model (SAM) by Meta AI
The Segment Anything Model (SAM) by Meta AI is an image segmentation tool that does not have a publicly disclosed pricing structure in the same way as software services with tiered plans. Here are the key points regarding its availability and usage:
Open Source Availability
SAM is released as an open-source project, which means the pre-trained models, code, and the SA-1B dataset are freely accessible under the Apache 2.0 license. This allows researchers and developers to use and modify the model without any licensing fees.
Free Access
Since SAM is open-source, there is no cost associated with using the model. You can download the pre-trained models and use them for various image segmentation tasks without any financial obligations.
No Tiered Plans
Unlike many software services, SAM does not offer different pricing tiers or plans. The model is available in its entirety for anyone to use, provided they comply with the terms of the Apache 2.0 license.
Usage and Support
While there are no direct costs, using SAM may require significant computational resources and technical expertise, especially for training and fine-tuning the model. However, there are no subscription fees or licensing costs involved.
Summary
In summary, the Segment Anything Model (SAM) by Meta AI is freely available as an open-source project, and there are no associated pricing tiers or costs for its use.
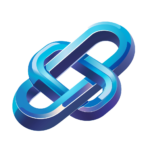
Segment Anything Model (SAM) by Meta AI - Integration and Compatibility
Integration with Other AI Models and Tools
SAM can be integrated with other advanced AI models and tools to enhance its functionality. For instance, it can work in conjunction with multimodal systems like OpenAI’s GPT-4 Vision and Anthropic’s multimodal Claude, allowing for diverse downstream applications such as real-time video segmentation in augmented reality (AR) and video editing.
Additionally, SAM can be combined with other image processing algorithms, such as Stable Diffusion for inpainting tasks. The Ikomia API, for example, streamlines the integration of SAM with other algorithms from platforms like YOLO, Hugging Face, and OpenMMLab, making it easy to manage multiple dependencies and perform various image processing tasks with simple text commands.
Compatibility Across Platforms
SAM is highly versatile and can be used across various platforms:
- Desktop and Cloud Environments: SAM can be integrated into tools like Encord, where it significantly improves AI-assisted labeling by reducing the time and effort required for segmenting images. Encord supports annotating images, videos, and specialized data types like satellite imagery and DICOM files, including medical imaging formats such as X-ray, CT, and MRI.
- Mobile Devices: The MobileSAM variant, optimized for mobile applications, uses a lightweight image encoder to reduce computational demands without compromising performance. This makes advanced AI-powered image segmentation accessible on mobile devices, enabling real-time applications with a processing time of about 12ms on a single GPU.
- Development Environments: SAM can be set up and used in Python environments, and there are comprehensive guides available for loading SAM, generating segmentation masks, and converting object detection datasets into segmentation masks.
Compatibility with Specific Software and Hardware
For technical implementation, SAM requires specific software and hardware:
- GPU Support: Processing image data on a GPU requires a supported GPU device and the Parallel Computing Toolbox™, especially when using tools like MATLAB’s Image Processing Toolbox Model for Segment Anything Model.
- Deep Learning Toolbox: The support package for SAM in MATLAB also requires the Deep Learning Toolbox™, ensuring that the model can be effectively utilized for image segmentation tasks.
In summary, SAM’s flexibility and compatibility make it a valuable tool that can be integrated into various AI-driven workflows, from desktop and cloud environments to mobile devices, and it supports a range of software and hardware configurations.
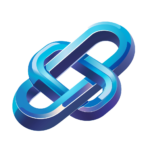
Segment Anything Model (SAM) by Meta AI - Customer Support and Resources
Customer Support
While the provided sources do not specify dedicated customer support channels like phone numbers or live chat services, users can reach out for issues or questions through email. For example, if you have any questions or issues regarding the SA-V dataset for SAM 2, you can email support@segment-anything.com.Additional Resources
Documentation and Guides
Meta AI provides comprehensive documentation and guides on how to use the Segment Anything Model. The official resources include detailed explanations of the model’s architecture, the SA-1B dataset, and how to implement the model for various image segmentation tasks.Open-Source Access
The Segment Anything Model, including the pre-trained models and code, is available open-source under the Apache 2.0 license. This allows researchers and developers to access and use the model freely, which is hosted on GitHub.Datasets
The SA-1B dataset, which contains over 1 billion high-quality segmentation masks derived from 11 million images, is a crucial resource. This dataset is diverse, covering various domains, objects, and scenarios, making it highly valuable for training and fine-tuning segmentation models.Interactive Demo
An interactive demo web UI is available, allowing users to test the Segment Anything Model with different prompts and see the segmentation results in real-time. This demo is part of the resources provided to help users get started with the model.Research Papers and Publications
For those interested in the technical details and research behind SAM, the official resources include links to research papers and publications. These provide in-depth information about the model’s architecture, training process, and performance.Community and Forums
While there is no specific mention of dedicated forums or community support channels, the open-source nature of the project and its presence on platforms like GitHub may facilitate community interaction and support through issues and discussions on these platforms. Overall, the resources provided by Meta AI for the Segment Anything Model are geared towards supporting researchers, developers, and users in effectively utilizing the model for various image segmentation tasks.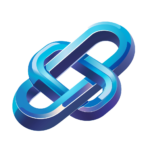
Segment Anything Model (SAM) by Meta AI - Pros and Cons
The Segment Anything Model (SAM) by Meta AI
The Segment Anything Model (SAM) by Meta AI is a significant advancement in image segmentation, offering several key advantages and some notable limitations.
Advantages
Zero-Shot Performance
Zero-Shot Performance: SAM can accurately segment images without the need for specific training on the task at hand, a capability known as zero-shot inference. This makes it highly versatile and efficient.
Real-Time Interaction
Real-Time Interaction: SAM can generate segmentation masks in as little as 50 milliseconds, even on a simple CPU in a web browser, making it suitable for real-time applications.
Minimal Supervision
Minimal Supervision: SAM requires much less human input compared to traditional models. It can work effectively with minimal labeled data, which is a significant advantage in many scenarios.
Diverse Applications
Diverse Applications: SAM can be applied in a wide range of fields, including healthcare, robotics, and environmental monitoring, due to its flexibility and generalization capabilities.
Large Dataset
Large Dataset: SAM is trained on the SA-1B dataset, which includes over 11 million images and 1 billion segmentation masks. This extensive dataset enhances its accuracy and ability to generalize across different tasks and environments.
Disadvantages
Fine-Grained Details
Fine-Grained Details: SAM can struggle with very detailed work, such as finding the exact edges of objects or handling very small objects. It may not perform as well as custom models in tasks requiring high precision.
Boundary Details
Boundary Details: SAM can miss the mark on detailed edges, which is a critical issue in applications like medical imaging or environmental monitoring where precise outlines are necessary.
Comparative Performance
Comparative Performance: In some tests, custom models have outperformed SAM, especially in complex datasets like COCO that require precise annotations. SAM may need further refinement in such scenarios.
High-Detail Tasks
High-Detail Tasks: While SAM is excellent at segmenting larger sections of images, it can be less accurate in tasks that require very detailed and precise segmentation.
In summary, SAM offers significant advantages in terms of its zero-shot performance, real-time capabilities, and minimal need for supervision. However, it faces challenges with fine-grained details and boundary precision, areas where custom models might be more effective.
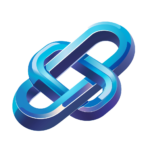
Segment Anything Model (SAM) by Meta AI - Comparison with Competitors
The Segment Anything Model (SAM) by Meta AI
The Segment Anything Model (SAM) by Meta AI stands out in the image tools AI-driven product category due to its innovative architecture and capabilities. Here’s a comparison with some of its competitors and an overview of its unique features:
Unique Features of SAM
- Promptable Segmentation: SAM is designed to generate segmentation masks based on any given prompt, whether it’s spatial or text clues identifying an object. This flexibility is a significant advancement in image segmentation tasks.
- Advanced Architecture: SAM uses a powerful image encoder, a prompt encoder, and a lightweight mask decoder. This architecture enables real-time mask computation and ambiguity awareness, making it highly versatile.
- Zero-Shot Performance: SAM displays outstanding zero-shot performance, meaning it can accurately segment images without prior specific training on those tasks. This is a result of its training on the large SA-1B dataset, which includes over 1 billion masks on 11 million images.
- Large-Scale Training Data: The SA-1B dataset is the largest segmentation dataset to date, providing SAM with a diverse and extensive training source that enhances its generalization across various tasks.
Comparison with Other Image Segmentation Tools
Adobe Firefly
- While Adobe Firefly is a powerful tool for text-to-image generation and has features like generative fill and text effects, it is not specifically optimized for image segmentation tasks. It integrates well with other Adobe products but lacks the specialized segmentation capabilities of SAM.
- Use Case: Firefly is more suited for creative professionals looking for text-to-image generation rather than precise image segmentation.
AI Product Photography Tools
Tools like Pebblely, Photoroom, Flair.ai, and Claid.ai are primarily focused on product photography and do not offer the same level of advanced image segmentation as SAM.
- Pebblely: Known for its user-friendly interface and bulk image processing, Pebblely is great for removing backgrounds and creating custom scenes but does not have the sophisticated segmentation capabilities of SAM.
- Photoroom: This tool excels in product blending and preservation but is more geared towards creating realistic product photos rather than complex image segmentation.
- Flair.ai: Offers extensive customization options and a design studio feel, but its focus is on enhancing product photos rather than advanced segmentation tasks.
- Claid.ai: Provides a full suite of tools for enhancing and generating product photos but does not match SAM’s advanced segmentation features.
General Differences
- Specialization: SAM is highly specialized in image segmentation, making it a go-to tool for tasks that require precise and flexible segmentation. Other tools, while powerful in their own right, are more generalized towards product photography and image enhancement.
- Training Data: The SA-1B dataset used by SAM is unparalleled in size and diversity, giving SAM a significant edge in handling a wide range of segmentation tasks.
- Zero-Shot Capability: SAM’s ability to perform well in zero-shot scenarios sets it apart from other models that might require specific training for each task.
In summary, while other AI tools excel in various aspects of image processing and product photography, the Segment Anything Model (SAM) by Meta AI is uniquely positioned due to its advanced architecture, zero-shot performance, and large-scale training data, making it an indispensable tool for complex image segmentation tasks.
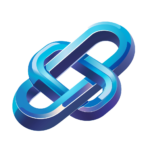
Segment Anything Model (SAM) by Meta AI - Frequently Asked Questions
Frequently Asked Questions about the Segment Anything Model (SAM) by Meta AI
What is the Segment Anything Model (SAM)?
The Segment Anything Model (SAM) is a state-of-the-art image segmentation model developed by Meta AI. It is designed for promptable segmentation tasks, allowing it to generate high-quality segmentation masks from various input prompts such as points, boxes, or text cues.
How does SAM work?
SAM works by using a combination of an image encoder, a prompt encoder, and a lightweight mask decoder. The image encoder generates image embeddings, the prompt encoder embeds the input prompts, and the mask decoder combines these embeddings to produce segmentation masks. This architecture enables flexible prompting and real-time mask computation.
What kind of input prompts can SAM handle?
SAM can handle a variety of input prompts, including spatial clues like points or bounding boxes, as well as text cues. This versatility allows users to specify objects they want to isolate in an image using different types of inputs.
What dataset was SAM trained on?
SAM was trained on the SA-1B dataset, which consists of over 11 million images and 1.1 billion segmentation masks. This large-scale dataset ensures SAM’s accuracy and reliability across various segmentation tasks.
What are some use cases for SAM?
SAM has several practical applications, including image editing, object tracking in videos, automated image annotation, medical imaging analysis, and aiding autonomous vehicle systems by detecting and separating road signs, pedestrians, and other obstacles.
How does SAM perform in zero-shot tasks?
SAM demonstrates strong zero-shot performance, meaning it can adapt to new image distributions and tasks without requiring additional training. This makes it a versatile tool for various segmentation tasks without the need for extensive fine-tuning.
Can SAM be fine-tuned for specific tasks?
Yes, SAM can be fine-tuned for specific tasks. Users can follow a step-by-step walkthrough to fine-tune the model for their particular needs, which can enhance its performance on specialized datasets or tasks.
What is the difference between SAM and SAM 2?
SAM 2 is an extension of the original SAM model, designed to handle video segmentation in addition to image segmentation. SAM 2 uses a streaming architecture that processes video frames one at a time and was trained on the SA-V dataset, which includes videos and masklets (object masks over time).
How can I use SAM in my projects?
To use SAM, you need to install the necessary dependencies and utilize the provided API. You can run inference with SAM using Python or through a command-line interface (CLI). There are also resources available for downloading related datasets and code.
Is SAM suitable for real-time applications?
Yes, SAM is designed for real-time performance. Its architecture prioritizes real-time mask computation, making it suitable for interactive applications such as video editing, AR/VR, and other real-time image processing tasks.
Where can I find more resources and support for SAM?
You can find more resources, including code, datasets, and documentation, on the official Meta AI website and other related resources like the Encord blog and Ultralytics documentation. For specific issues or questions, you can also contact support@segment-anything.com.
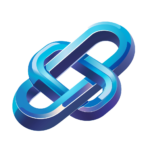
Segment Anything Model (SAM) by Meta AI - Conclusion and Recommendation
Final Assessment of the Segment Anything Model (SAM)
The Segment Anything Model (SAM) by Meta AI is a revolutionary tool in the image segmentation domain, offering unprecedented versatility and accuracy. Here’s a comprehensive assessment of SAM, including its benefits and the groups that would most benefit from its use.Key Features and Capabilities
SAM stands out due to its advanced architecture, which includes an image encoder, a prompt encoder, and a lightweight mask decoder. This design enables flexible prompting, real-time mask computation, and the ability to handle ambiguous segmentation tasks.Zero-Shot Performance and Adaptability
One of SAM’s most significant advantages is its zero-shot transfer capability, allowing it to adapt to new image distributions and tasks without prior specific training. This is made possible by its training on the extensive SA-1B dataset, which contains over 1 billion segmentation masks from 11 million diverse images.Promptable Segmentation
SAM is optimized for promptable segmentation tasks, enabling users to generate valid segmentation masks using various prompts such as spatial or text clues. This feature makes it highly versatile and efficient for a wide range of applications.Applications and Use Cases
SAM has a broad spectrum of applications across various industries:- Medical Imaging: SAM is highly effective in segmenting regions of interest like tumors or specific organs, providing more accurate diagnostic support.
- Autonomous Systems: It can segment out elements in camera feeds, such as pedestrians, vehicles, and other objects, which is crucial for autonomous vehicles.
- Environmental Monitoring: SAM can classify and map different types of land cover, aiding in urban planning, agriculture, and environmental monitoring.
- AI-Assisted Labeling: It significantly streamlines the process of labeling images by automatically identifying and segmenting objects, reducing the time and effort required for manual annotation.
Who Would Benefit Most
SAM would be particularly beneficial for:- AI Researchers and Developers: Those working on computer vision tasks can leverage SAM’s advanced segmentation capabilities to improve their workflows and achieve precise segmentation outcomes with minimal configuration.
- Healthcare Professionals: SAM’s precision in medical imaging can enhance diagnostic accuracy and treatment precision, making it a valuable tool in healthcare.
- Autonomous Vehicle Engineers: The model’s ability to segment objects in real-time is crucial for the development of autonomous systems.
- Environmental Scientists: SAM can aid in analyzing satellite imagery for climate change studies, land cover mapping, and other environmental monitoring tasks.