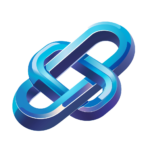
H2O.ai - Detailed Review
Research Tools
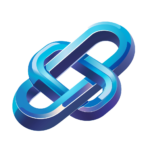
H2O.ai - Product Overview
Overview
H2O.ai is a leading provider of AI and machine learning solutions, aimed at helping businesses leverage advanced algorithms and data science to drive innovation and growth.
Primary Function
H2O.ai’s primary function is to provide a comprehensive platform for building, deploying, and managing machine learning models. The platform is designed to accelerate the data science workflow, enabling users to develop highly accurate predictive models quickly and efficiently. This is achieved through automated machine learning (autoML) capabilities, feature engineering, and model deployment tools.
Target Audience
H2O.ai caters to a diverse range of industries, including finance, healthcare, retail, manufacturing, and technology. The platform is accessible to both data scientists and business users, making it versatile for organizations of all sizes, from small startups to large corporations. This broad appeal is due to its user-friendly interface and the ability to handle various data types and use cases.
Key Features
Automated Machine Learning (autoML)
H2O.ai’s autoML capabilities automate the entire machine learning workflow, from feature transformation to model selection, monitoring, and deployment. This includes automated feature selection and engineering, which simplify the process of building accurate models.
Advanced Algorithms
The platform incorporates a wide range of algorithms such as deep learning, gradient boosting, and random forests. These algorithms are essential for tasks like classification, regression, and clustering.
H2O Driverless AI
This product accelerates the data science workflow by automating the process of building highly accurate predictive models. It is particularly useful for users who need to develop models quickly without extensive manual intervention.
H2O-3
An open-source, distributed machine learning platform that allows users to build and deploy machine learning models at scale. It is fast, scalable, and easy to use.
H2O Wave
A lightweight, open-source platform for building and deploying interactive web applications. It enables users to create data-driven applications that can be easily shared and accessed.
Cross-Team Collaboration
The platform enhances collaboration among teams by providing tools for model management, deployment, and monitoring. This includes features like model validation and an app store for rapid prototyping and deployment of AI applications.
Conclusion
Overall, H2O.ai’s products and services are designed to make AI accessible and actionable for a wide range of users, helping organizations to make data-driven decisions and drive business innovation.
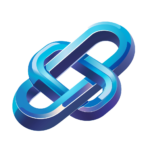
H2O.ai - User Interface and Experience
User Interface Overview
The user interface of H2O.ai, particularly in its research tools and AI-driven products, is designed to be intuitive and accessible, even for users with limited technical expertise.Navigation and Layout
H2O.ai’s interface, such as in the Document AI – Publisher, features several key navigation elements. The left navigation bar allows users to move between different pages like Document sets, Annotation sets, Models, Jobs, and Published Pipelines, each displaying the number of items on the page. The upper navigation bar changes depending on the page and provides tasks that can be performed without selecting a file or with batch operations. For example, on the Project page, you can delete the project, export project images, or import various sets and models.Information and Actions
The Info button at the end of each set, model, or job row provides access to detailed information, logs, and the ability to update names and descriptions. Additionally, the drop-down arrow next to the Info button offers primary actions such as editing, renaming, splitting, exporting, or deleting sets.H2O Flow Interface
H2O Flow, a web-based interactive environment, combines code execution, text, mathematics, plots, and rich media in a single document. This interface allows users to import files, build models, make predictions, and present their work interactively. It blends command-line computing with a graphical user interface, enabling users to perform H2O operations without writing code. Users can click through operations, and the interface provides input prompts, interactive help, and example flows to guide them.Ease of Use
Despite the advanced capabilities, H2O.ai aims to make machine learning accessible to a broad demographic, including data scientists and business analysts. The platform offers automatic visualization of model performance, comprehensive auto-documentation of models, and features like AutoML that automate the selection, training, and tuning of machine learning models. These features significantly reduce the barriers to entry for users without deep technical expertise.User Experience
The user experience is enhanced by the intuitive design of the interface. H2O Flow, for instance, allows users to capture, rerun, annotate, present, and share their workflow seamlessly. The platform supports continuous learning and optimization, enabling models to adapt to new data and changing patterns. Tools for model interpretation, such as feature importance scores, partial dependence plots, and SHAP values, help users understand and trust their models.Learning Curve
However, it is worth noting that while the interface is user-friendly, there can still be a steep learning curve for beginners. Users need a good understanding of machine learning concepts and data science techniques to fully leverage the platform’s advanced features.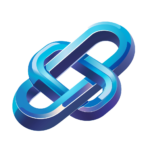
H2O.ai - Key Features and Functionality
H2O.ai Overview
H2O.ai offers a suite of AI-driven products that are highly integrated and designed to streamline various aspects of machine learning, data analysis, and model deployment. Here are the main features and functionalities of these products:H2O AI Cloud
H2O AI Cloud (HAIC) is an end-to-end AI platform that enables users to create, deploy, monitor, and share data models and AI applications. Here are its key components:AI App Store
This allows users to discover and deploy pre-built AI applications.H2O Enterprise Steam
Part of the HAIC, this component is focused on enterprise-level AI deployment and management.H2O Driverless AI
This is a core component that automates many aspects of the machine learning workflow, which is discussed in more detail below.H2O-3
This is the core machine learning engine of H2O.ai, supporting a wide range of algorithms.H2O MLOps
This component focuses on the operationalization of machine learning models, making it easier to deploy and manage models in production environments.H2O Driverless AI
Driverless AI is a significant part of the H2O.ai ecosystem, offering several key features:Automatic Feature Engineering
Driverless AI employs a library of algorithms and feature transformations to automatically engineer new, high-value features from the dataset. This process is crucial for extracting accurate results from machine learning algorithms.Automated Machine Learning
It automates the process of creating, selecting, and tuning machine learning models, which includes data visualization, feature engineering, predictive modeling, and model explanation. This automation helps in saving time and resources that would otherwise be spent on manual model tuning.Flexibility of Data and Deployment
Driverless AI can work with various data sources such as Hadoop HDFS, Amazon S3, and others. It can be deployed on multiple platforms including clouds (AWS, Azure, Google Cloud), on-premises, and on machines with CPUs or GPUs.Model Documentation
Driverless AI provides an Autoreport (Autodoc) for each experiment, which includes details about the data used, validation schema, model and feature tuning, and the final model created. This feature helps in documenting and summarizing the workflow used in building machine learning models.H2O AutoInsights
H2O AutoInsights is integrated within the H2O AI Cloud and is designed to automatically explore and discover hidden insights in the data using statistical and machine learning analyses.Automated Data Analysis
AutoInsights can prep the data and extract insights, presenting them in interactive visuals and easy-to-understand narrative summaries. This helps in clarifying and elevating the value of the data.Integration and Scalability
H2O.ai products are designed to integrate seamlessly with popular data science tools and environments such as R, Python, Apache Spark, and Hadoop. This integration allows for scalable data processing and machine learning, making it suitable for large datasets.Model Explainability and Deployment
H2O.ai provides features like model explainability, which helps in understanding the inner workings of the models. This is crucial for trust-building and compliance. Models created in H2O.ai can be easily deployed into production environments using REST APIs and scoring pipelines.Predictive Analytics and NLP
H2O.ai supports a wide array of machine learning algorithms, including Generalized Linear Models (GLM), Gradient Boosting Machines (GBM), Deep Learning, and more. It also includes Natural Language Processing (NLP) capabilities, which can be used to generate automated response suggestions based on historical data and predictive analysis. By leveraging these features, H2O.ai makes AI and machine learning more accessible and user-friendly, enabling widespread adoption across various industry sectors.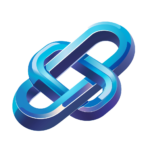
H2O.ai - Performance and Accuracy
Evaluating H2O.ai in AI-Driven Research Tools
Evaluating the performance and accuracy of H2O.ai in the AI-driven research tools category involves several key aspects, including its capabilities, limitations, and areas for improvement.
Performance
H2O.ai is recognized for its strong performance in handling large-scale machine learning tasks. Here are some highlights:
- The platform supports continuous learning and optimization, allowing models to adapt to new data and changing patterns, which enhances their performance over time.
- H2O.ai’s hyperparameter tuning tools are particularly effective, enabling continuous optimization to improve model performance.
- The platform is scalable and can handle complex algorithms and large datasets with ease, making it suitable for various industries such as finance, healthcare, and retail.
Accuracy
In terms of accuracy, H2O.ai offers several features that contribute to reliable and accurate predictions:
- The platform provides tools for evaluating model performance using specific metrics, stopping metrics, and performance graphs. For example, the `h2o.performance()` function in R or `model_performance()` in Python helps in assessing a model’s performance on a given dataset.
- H2O.ai’s AutoML feature, while not fully automated, is helpful in saving time by performing random searches for hyperparameter optimization and model stacking, which can lead to more accurate models.
Limitations
Despite its strengths, H2O.ai has some limitations:
- Resource-Intensive Operations: Running large-scale machine learning models can be computationally intensive, requiring substantial hardware or cloud resources, which can increase costs and processing times.
- Limited Support for Certain Algorithms: While H2O.ai supports a wide range of machine learning algorithms, it may not cover all niche or highly specialized algorithms, requiring users to integrate additional tools or custom implementations.
- Steep Learning Curve: Although H2O.ai provides a user-friendly interface, it still presents a steep learning curve for beginners due to the need for a good understanding of machine learning concepts and data science techniques.
- Data Preparation: H2O.ai’s AutoML does not perform data preparation steps such as feature engineering, class balancing, or filtering correlated features, which must be done manually.
Areas for Improvement
To further enhance its performance and accuracy, H2O.ai could focus on the following areas:
- Expanded Algorithm Support: Incorporating more specialized algorithms to cater to a broader range of use cases.
- Automated Data Preparation: Integrating automated data preparation steps into the AutoML process to make the platform more end-to-end.
- Resource Optimization: Improving the efficiency of resource usage to reduce costs and processing times for large-scale models.
Overall, H2O.ai is a powerful tool for machine learning and predictive analytics, offering strong performance and accuracy. However, users need to be aware of its limitations and the need for additional expertise and resources in certain areas.
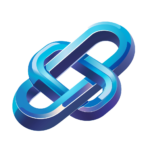
H2O.ai - Pricing and Plans
H2O.ai Pricing Structure
H2O.ai offers a diverse and flexible pricing structure to cater to a wide range of users, from individual hobbyists to large enterprises. Here’s a breakdown of the different plans and features:Free Open-Source Version
H2O.ai provides a free open-source version that allows users to access core functionalities without any cost. This version includes a wide array of machine learning algorithms and is ideal for those who want to experiment with machine learning models without a financial commitment. The open-source tools, such as H2O, Driverless AI, Sparkling Water, and H2O Wave, are licensed under the Apache License, Version 2.0.Enterprise Solutions
For organizations requiring advanced features and support, H2O.ai offers enterprise-level solutions. Here are some key points:H2O.ai AI Cloud
- Cost: $50,000 per unit, with a minimum purchase requirement of four units.
- Features: This plan includes enhanced capabilities such as real-time data scoring, automated machine learning, regularization techniques, and distributed in-memory computing. It is designed for larger-scale deployments and offers significant support and advanced features not available in the open-source version.
Subscription Models
H2O.ai’s pricing for enterprise subscriptions can vary significantly:- Subscriptions: Ranging from $300,000 for a 3-year subscription to $850,000 for a 5-year subscription with GPU support. These subscriptions are typically geared towards large enterprises and can be quite expensive.
Key Features Across Plans
- Real-time Data Scoring: Provides immediate insights from data as it is ingested.
- Automated Machine Learning: Streamlines the model-building process, making it accessible to users with varying levels of expertise.
- Regularization Techniques: Supports both L1 and L2 regularization to improve model performance and prevent overfitting.
- Distributed In-memory Computing: Facilitates high-speed data processing and model training across multiple nodes.
Deployment Options
H2O.ai solutions can be deployed in various environments:- Air-gapped, On-premises, Private, or Managed Cloud Deployments: Users have the flexibility to choose the deployment method that best suits their needs.
Additional Considerations
- Cost of Proprietary Tools: While the open-source version is free, the proprietary tools and enterprise solutions can be expensive, which may be a barrier for smaller organizations.
- Required Expertise: Advanced features may require a solid understanding of statistics and machine learning principles to utilize effectively.
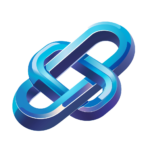
H2O.ai - Integration and Compatibility
Integration with H2O AI Hybrid Cloud
One of the key integration points is within the H2O AI Hybrid Cloud. Here, the AI App Store is tightly integrated with other components of the cloud, such as ML Engine management and Model management. This integration is facilitated through several mechanisms:
- Shared User Identity: The use of OpenID Connect (OIDC) allows for a single identity across all components of the H2O AI Hybrid Cloud. This enables Wave apps to make API calls on behalf of the user, leveraging the same OIDC access tokens accepted by other components.
- Shared Storage API: Apps within the App Store can use the same storage API as other components, ensuring transparent data access and processing without the need for configuring separate connectors or storage resources.
- Dependency Injection: Apps are loosely coupled with H2O AI Hybrid Cloud components through environment-based dependency injection, allowing for flexible and modular integration.
Model and ML Engine Management
For model deployments, the App Store relies on H2O MLOps, which requires configuring OIDC clients to obtain specific scopes (`ai.h2o.storage` and `ai.h2o.deploy`) for token authentication. This setup allows OIDC-enabled Wave apps to interact with MLOps on behalf of the user via the MLOps Python library.
Similarly, for ML engine management, the App Store integrates with H2O AI Engines (e.g., Driverless AI) by configuring the AI Engine Manager with OIDC platform public clients. This ensures that the necessary token scopes are obtained, and user roles are mapped correctly.
Compatibility Across Platforms
H2O.ai products are highly compatible across various deployment environments:
- Managed Cloud: Fully hosted and managed by H2O.ai, this option is SOC2 Type 2 and HIPAA compliant, offering a quick setup within a day. It includes end-to-end managed data science and machine learning platforms without the need for daily operations or maintenance.
- Hybrid Cloud: Customers can host the H2O AI Cloud in their Virtual Private Cloud (VPC) using Kubernetes on public clouds like AWS, Azure, or GCP, or on-premise using Kubernetes flavors like Red Hat OpenShift or HPE Ezmeral. This provides complete control over infrastructure, software updates, security, and compliance.
- On-Premise and Air-Gapped Environments: H2O.ai solutions can be deployed on-premise or in air-gapped environments, ensuring flexibility and control over data and infrastructure. These deployments are also SOC2 Type 2 and HIPAA compliant.
GPU-Accelerated Machine Learning
H2O4GPU, another product from H2O.ai, is an open-source, GPU-accelerated machine learning package. It is optimized for NVIDIA GPUs and can be used as a drop-in replacement for scikit-learn with support for GPUs on selected algorithms. This package is available with APIs in Python and R, making it compatible with a wide range of machine learning workflows.
In summary, H2O.ai’s products are designed to integrate seamlessly with various components of the H2O AI Hybrid Cloud and are compatible across different deployment environments, including managed cloud, hybrid cloud, on-premise, and air-gapped setups. This ensures a flexible and efficient AI solution that can be adapted to different user needs and infrastructure configurations.
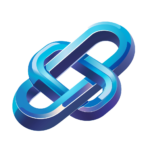
H2O.ai - Customer Support and Resources
H2O.ai Customer Support Overview
H2O.ai offers a comprehensive range of customer support options and additional resources to ensure users of their AI-driven products receive the assistance they need.
Support Channels
- Email Support: H2O.ai provides monitored email support, available 24×5 for silver support tier customers and 24×7 for gold and platinum support tier customers.
- Telephone Support: Customers can access manned telephone support, also available 24×5 for silver tier and 24×7 for gold and platinum tiers.
- Web Support: Support is available through the H2O.ai support portal, where customers can submit service requests and access various resources.
Service Scope
- Online Documentation and Resources: Users have access to extensive online documentation and resources to help them use the products effectively.
- Self-Serviced Community Support: H2O.ai offers community support where users can interact with each other and find solutions to common issues.
- Scheduled Live Calls: Customers can request scheduled live calls for more personalized support.
- Training Services: For gold and platinum support tiers, H2O.ai provides training services to help users get the most out of their products.
- Technical Account Manager (TAM), Customer Success Manager (CSM), and Data Scientist (DS) Consultation: These services are also available for gold and platinum support tiers, providing expert guidance and support.
Response Times
H2O.ai has defined response times for service-related incidents:
- P1 Priority Issues: Responded to within 1 hour.
- P2 Priority Issues: Responded to within 4 hours.
- P3 Priority Issues: Responded to within 1 business day.
Additional Resources
- Support Portal: Customers can access a dedicated support portal where they can submit requests, track issues, and find resources.
- Technical Support Engineers: Gold and platinum tier customers are assigned primary technical support engineers who are available 24×7.
- Knowledge Base: H2O.ai maintains a knowledge base that includes various resources and documentation to help users resolve issues on their own.
Product-Specific Support
For products like H2O Driverless AI, users benefit from automated machine learning capabilities, extensive feature engineering, model development, and interpretability tools. The platform includes an AI Wizard that provides proactive data science guidance, making it easier for users to build and deploy machine learning models efficiently.
By providing these comprehensive support options and resources, H2O.ai ensures that users can effectively utilize their AI-driven products and resolve any issues promptly.
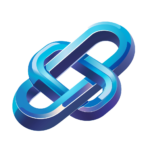
H2O.ai - Pros and Cons
Advantages of H2O.ai
H2O.ai offers several significant advantages that make it a compelling choice in the AI-driven research tools category:
User-Friendly Interface
H2O.ai is known for its easy-to-use platform, making it accessible to a broad range of users, including data scientists, developers, and business users. The intuitive UI and AppStore enable anyone to build AI applications without extensive coding skills.
Automated Machine Learning (AutoML)
H2O.ai’s AutoML feature automates the machine learning workflow, including algorithm selection, feature engineering, hyperparameter tuning, and model assessment. This significantly reduces the time and expertise required to deploy ML models.
Scalability and Performance
The platform is highly scalable and can handle large datasets and intensive workloads. It leverages distributed in-memory processing to accelerate machine learning tasks, making it suitable for big data environments.
Integration and Deployment
H2O.ai seamlessly integrates with existing systems and applications, and it offers flexible deployment options, including on-premises, cloud-based, and hybrid models. This flexibility allows users to adapt the AI solution to their specific needs and workflows.
Advanced Algorithms and Models
The platform includes a comprehensive collection of machine learning algorithms, such as gradient boosted machines, generalized linear models, deep learning, and more. These algorithms are highly scalable and efficient, capable of processing vast datasets.
Security and Compliance
H2O.ai provides advanced security and data governance features, ensuring data protection and compliance with standards like SOC2 Type 2 and HIPAA/HITECH. The platform also supports air-gapped, on-premises, and private cloud deployments.
Community and Support
H2O.ai fosters a strong community around data science and machine learning, offering industry-leading support from experienced data scientists and engineers. This includes training, dedicated account management, and 24/7 support.
Disadvantages of H2O.ai
While H2O.ai offers many benefits, there are also some notable drawbacks:
Advanced Functionality Limitations
Due to its advanced functionality, the platform may not be suitable for all users and businesses, particularly those with limited data science experience or resources.
Model Tuning and Validation Challenges
Some users may experience difficulties with model tuning and validation, especially if they lack extensive data science knowledge.
Data Modification Issues
Once data is uploaded to the platform, modifying it can be challenging.
Limited User Access
There are limitations on the number of users who can access each other’s information, which can hinder collaboration in some cases.
Edge Computing Support
H2O.ai does not currently support edge computing, which might be a limitation for certain use cases.
DataFrame Manipulation
The DataFrame manipulation capabilities in H2O.ai are considered primitive compared to tools like R and Pandas, which can be a drawback for some users.
By considering these pros and cons, users can make an informed decision about whether H2O.ai aligns with their specific needs and capabilities.
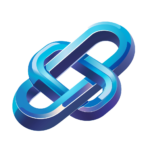
H2O.ai - Comparison with Competitors
When Comparing H2O.ai to Competitors
When comparing H2O.ai to its competitors in the AI-driven research tools category, several key features and differences stand out.
Unique Features of H2O.ai
- AutoML Capability: One of the standout features of H2O.ai is its automated machine learning (AutoML) functionality. This feature automatically selects, trains, and tunes a variety of machine learning models, saving time and effort by handling the complex aspects of model building. It evaluates multiple models and selects the best-performing one based on specified criteria.
- Model Interpretability: H2O.ai provides comprehensive tools for model interpretation, including feature importance scores, partial dependence plots, and SHAP (SHapley Additive exPlanations) values. These tools help in explaining the model’s behavior, ensuring transparency and trust in the machine learning models.
- End-to-End Workflow: The platform automates and streamlines the entire data science workflow, from data ingestion and preprocessing to model training and deployment. This makes it a holistic solution for data scientists and machine learning engineers.
Competitors and Alternatives
- DataRobot: DataRobot is another AI lifecycle platform that offers augmented intelligence, data engineering, and machine learning solutions. It integrates well with various sectors such as banking, healthcare, and retail. Unlike H2O.ai, DataRobot is not open-source and has a more focused approach on enterprise-level AI solutions. DataRobot also provides AutoML capabilities but is known for its broader ecosystem integrations and support for multiple industries.
- Dataiku: Dataiku is a platform that enables data experts and domain experts to work together to build data into their daily operations. It supports advanced analytics and generative AI, similar to H2O.ai, but has a stronger focus on collaboration and everyday AI use. Dataiku is not open-source and is more geared towards integrating AI into business operations.
- Amazon SageMaker: Amazon SageMaker is a fully managed service that provides a range of machine learning algorithms and tools. It supports AutoML and model interpretability but is part of the AWS ecosystem, which might be a consideration for those already invested in AWS services. Unlike H2O.ai, SageMaker is not open-source and has a more cloud-centric approach.
- Altair AI Studio: Altair AI Studio is often cited as a top alternative to H2O.ai. It offers a user-friendly interface and supports a wide range of machine learning tasks. However, it may lack the extensive AutoML and model interpretability features that H2O.ai provides.
- RapidMiner: RapidMiner offers a powerful and intuitive graphical user interface for designing analytic processes. It supports the reuse of R and Python code and has a strong community-driven approach. While it is user-friendly, it may not match the breadth of AutoML and model interpretability features offered by H2O.ai.
Key Considerations
When choosing between H2O.ai and its competitors, consider the following:
- Open-Source vs. Proprietary: H2O.ai is open-source, which can be a significant advantage for those who value community support and customization. In contrast, many of its competitors are proprietary.
- AutoML and Interpretability: If AutoML and model interpretability are critical, H2O.ai stands out with its comprehensive tools in these areas.
- Integration and Ecosystem: Consider the ecosystem you are already using. For example, Amazon SageMaker integrates well with AWS, while DataRobot has broad industry support.
- User Interface and Collaboration: Dataiku and Altair AI Studio offer user-friendly interfaces and strong collaboration features, which might be important for teams with diverse skill levels.
Each platform has its strengths, and the choice ultimately depends on your specific needs, such as the level of automation, interpretability, and integration required.
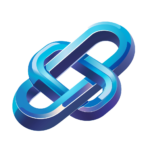
H2O.ai - Frequently Asked Questions
What is H2O.ai and what does it offer?
H2O.ai is a fully open-source, distributed in-memory machine learning platform. It supports a wide array of statistical and machine learning algorithms, including gradient boosted machines, generalized linear models, deep learning, and more. The platform is known for its linear scalability and industry-leading AutoML functionality, which automates the process of running through various algorithms and their hyperparameters to produce a leaderboard of the best models.
What are the key features of H2O.ai?
Key features of H2O.ai include Automated Machine Learning (AutoML), which streamlines the model-building process; real-time data scoring, enabling immediate insights from data; regularization techniques (L1 and L2) to prevent overfitting; and distributed in-memory computing for high-speed data processing and model training. Additionally, H2O.ai offers features like feature transformation, feature engineering, and explainable AI with white box models and interpretability methods.
What is the pricing structure for H2O.ai?
H2O.ai offers a variety of pricing options. The core functionality is available as a free open-source version, ideal for users who want to experiment with machine learning models without a financial commitment. For organizations seeking advanced features and support, H2O.ai provides enterprise-level solutions, such as the H2O AI Cloud, which is priced at $50,000 per unit with a minimum purchase requirement of four units.
What is H2O Driverless AI and how does it work?
H2O Driverless AI is an automatic machine learning platform that employs the techniques of expert data scientists in an easy-to-use application. It helps scale data science efforts by automating tasks, allowing data scientists to work on projects much faster. This platform can accomplish tasks in minutes rather than months, making it a valuable tool for speeding up data science projects.
What is H2O Document AI and its capabilities?
H2O Document AI is an engine within the H2O AI Cloud that helps organizations process and manage large amounts of unstructured data. It can classify documents, extract text, tables, and images, and group, label, and refine extracted information. H2O Document AI uses a combination of Intelligent Character Recognition (ICR), document layout understanding, and Natural Language Processing (NLP) to develop highly accurate models.
Can H2O.ai handle time series forecasting?
Yes, H2O.ai has capabilities specifically designed for time series forecasting. It offers unique feature engineering and AutoML capabilities to handle time series data, allowing users to easily fit and solve forecasting problems across various categories such as individual SKUs and product hierarchies.
Is H2O.ai user-friendly for non-experts?
H2O.ai is designed to be intuitive and user-friendly, making it accessible for non-experts. The platform includes a low-code framework that simplifies the development and deployment of AI applications, making it easier for users with varying levels of expertise to build and test machine learning models.
What kind of support does H2O.ai offer for different data types?
H2O.ai supports a variety of data types within a single dataset. It includes capabilities for natural language processing (NLP), computer vision, and other machine learning tasks. The platform is versatile and can handle different types of data, from structured to unstructured, including documents, images, and more.
How does H2O.ai ensure model interpretability and explainability?
H2O.ai provides explainable AI features, including white box models and various interpretability methods. These features help in understanding how the models make predictions, which is crucial for model transparency and trustworthiness. Additionally, the platform includes bias detection tools to ensure fairness in the models.
Is H2O.ai scalable for large-scale deployments?
Yes, H2O.ai is highly scalable and suitable for large-scale deployments. The platform uses distributed in-memory computing, which facilitates high-speed data processing and model training across multiple nodes, ensuring linear scalability and efficient handling of large datasets.
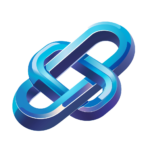
H2O.ai - Conclusion and Recommendation
Final Assessment of H2O.ai
H2O.ai is a formidable player in the AI-driven research tools category, offering a comprehensive suite of machine learning and predictive analytics solutions. Here’s a detailed assessment of who would benefit most from using H2O.ai and an overall recommendation.
Key Benefits and Features
- Automated Machine Learning (AutoML): H2O.ai’s AutoML capabilities are a standout feature, automatically selecting, training, and tuning a variety of machine learning models. This saves time and effort, making it easier for both novice and experienced data scientists to develop highly accurate predictive models.
- Comprehensive Data Science Lifecycle: The platform optimizes the entire data science workflow, from data ingestion and preprocessing to model training and deployment. It includes advanced features for machine learning interpretability and operations, ensuring higher accuracy, transparency, and faster adaptations.
- Cross-Team Collaboration: H2O.ai enhances collaboration between data scientists, developers, and machine learning engineers. It provides seamless integration of AI capabilities into existing solutions and supports comprehensive model monitoring and management.
- Smart Segmentation and Marketing: The platform is particularly useful for marketing teams, enabling advanced customer segmentation using a wide range of data, including browsing behavior, prior purchases, and demographics. This leads to more targeted marketing campaigns with higher conversion rates and revenues.
- Generative and Predictive AI: H2O.ai is at the forefront of converging generative and predictive AI, offering a range of models and tools for document processing, fraud detection, recommendation engines, and more. This makes it highly versatile for various business and social challenges.
Who Would Benefit Most
- Enterprise Businesses: Large enterprises looking to implement AI solutions to improve efficiency and decision-making processes can significantly benefit from H2O.ai. It is particularly useful in industries such as finance, healthcare, retail, and manufacturing.
- Data-Driven Companies: Organizations that rely heavily on data-driven insights to enhance their predictive analytics capabilities will find H2O.ai invaluable. The platform’s advanced analytics and predictive modeling tools help in making informed decisions and personalizing customer interactions.
- Startups and Tech Companies: Smaller tech companies and startups aiming to innovate and stay ahead of the competition can leverage H2O.ai’s AutoML and other features to quickly develop and deploy AI applications without extensive resources.
- Data Scientists and Machine Learning Engineers: Professionals in these roles will appreciate the platform’s advanced AutoML capabilities, model monitoring features, and the ability to integrate AI into existing solutions seamlessly.
Overall Recommendation
H2O.ai is a highly recommended platform for any organization or individual looking to leverage AI and machine learning to drive business growth and innovation. Its user-friendly interface, comprehensive features, and the ability to handle the entire data science lifecycle make it an excellent choice.
- Cost-Effectiveness: H2O.ai offers significant cost savings, particularly in query costs and document processing, making it an economical option for businesses of all sizes.
- Flexibility and Security: The platform can be deployed in various environments, including on-premise, air-gapped, and managed cloud, ensuring flexibility and security for sensitive data.
- Continuous Innovation: H2O.ai is continuously innovating, as evidenced by its inclusion in Gartner Magic Quadrants and Forrester Wave reports, ensuring users have access to the latest advancements in AI and machine learning.
In summary, H2O.ai is a powerful tool that democratizes AI, making it accessible and beneficial for a wide range of users, from data scientists and machine learning engineers to enterprise businesses and startups. Its comprehensive features, cost-effectiveness, and flexibility make it a top choice in the AI-driven research tools category.