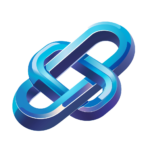
Whisper (by OpenAI) - Detailed Review
Speech Tools
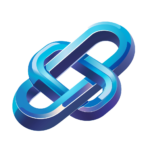
Whisper (by OpenAI) - Product Overview
Introduction to OpenAI Whisper
OpenAI Whisper is an advanced Automatic Speech Recognition (ASR) system developed by OpenAI, released in September 2022. Here’s a breakdown of its primary function, target audience, and key features:Primary Function
Whisper’s main purpose is to transcribe spoken language into written text with high accuracy. It can handle speech-to-text transcription and translate speech from various languages into English.Target Audience
The primary intended users of Whisper are AI researchers studying aspects such as robustness, generalization, capabilities, biases, and constraints of the model. However, it is also useful for developers, particularly those working on English speech recognition and other multilingual applications. Additionally, it can be beneficial for product demos, academic projects, and indie initiatives.Key Features
- Multilingual Support: Whisper can transcribe and translate speech in around 100 languages, making it highly versatile for global use.
- Accuracy and Performance: Trained on 680,000 hours of supervised data, Whisper achieves an average word error rate of 8.06%, indicating it is about 92% accurate. It performs well in diverse acoustic conditions, including noisy environments and multilingual audio.
- Model Sizes: Whisper is available in several model sizes, ranging from 39 million to 1.55 billion parameters. Larger models offer higher accuracy but at the cost of longer processing times and higher computational costs.
- Transformer Architecture: Whisper uses an end-to-end deep learning model based on an encoder-decoder Transformer architecture. This allows it to contextualize words and handle long-range dependencies, enhancing transcription accuracy.
- Additional Capabilities: Beyond basic transcription, Whisper can be fine-tuned for tasks like live-streaming transcription, speaker diarization, voice activity detection, and recognizing industry-specific jargon and terms.
- Noise Reduction and Error Correction: Whisper features powerful algorithms for noise reduction and error correction, making it effective in noisy environments and capable of correcting minor speech errors like stumbles and mispronunciations.
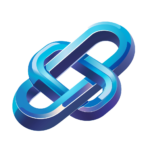
Whisper (by OpenAI) - User Interface and Experience
User Interface and Experience of OpenAI’s Whisper
The user interface and experience of OpenAI’s Whisper speech recognition system can vary depending on how you choose to use it, but here are some key points to consider:
Using Whisper via Web UI
For users who prefer a more straightforward approach, tools like the Whisper Web UI provide an easy-to-use interface. This web-based tool allows you to upload audio files or record audio directly, which is then transcribed into text using the OpenAI Whisper API. The process is relatively simple: you upload your audio, and the system transcribes it, handling accents, background noise, and technical language effectively. This method is particularly useful for those on mobile phones or Windows computers, as it eliminates the need to install any software.
Local Installation
For more advanced users, Whisper can be run locally on their computers. This involves downloading the necessary files from GitHub and running some code to install the system. While this method is free and does not require any subscription fees, it does require technical knowledge and resources to set up and maintain.
Integration with Other Apps
Whisper can also be integrated into other applications, such as the ChatGPT app for Android and iOS. Here, Whisper handles voice input, allowing users to quickly interact with ChatGPT using voice commands. This integration makes the interaction feel more natural and responsive, similar to talking to a trusted assistant.
Ease of Use
Despite its advanced capabilities, Whisper’s ease of use can vary. For those using the web UI or integrated apps, the process is generally straightforward and user-friendly. However, for users who choose to run Whisper locally, the setup process can be more technical and time-consuming. Users have noted that while Whisper is highly accurate, the lack of a simple download and install process can be a barrier for less technical users.
User Experience
The overall user experience with Whisper is highly positive in terms of accuracy and functionality. Whisper’s ability to transcribe speech accurately, even in noisy environments and with various accents, makes it a valuable tool. It can handle multiple languages, translate them into English, and maintain correct punctuation and syntax, which reduces the need for manual corrections. Users have reported high satisfaction with Whisper’s performance, noting that it can significantly speed up tasks such as writing documents, transcribing meetings, and adding subtitles to videos.
Real-Time Transcription
One of the standout features of Whisper is its real-time transcription capability. This allows users to see the text as they speak, which is particularly useful in applications like customer service call centers, where agents can focus on the conversation rather than taking notes. This real-time feature enhances productivity and improves the overall user experience.
Conclusion
In summary, Whisper’s user interface is flexible and can be accessed through various methods, each with its own level of ease of use. While it may require some technical effort to set up locally, the web-based and app-integrated versions offer a more user-friendly experience. The system’s high accuracy and real-time transcription capabilities make it a highly effective and engaging tool for a wide range of applications.
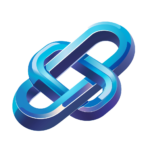
Whisper (by OpenAI) - Key Features and Functionality
OpenAI’s Whisper Overview
Whisper is a sophisticated automatic speech recognition (ASR) system that boasts several key features and functionalities, making it a powerful tool in the speech tools AI-driven product category.Speech-to-Text Transcription
Whisper’s primary function is to transcribe spoken language into text. It achieves this through an end-to-end deep learning model based on an encoder-decoder Transformer architecture. The input audio is split into 30-second chunks, converted into a log-Mel spectrogram, and then processed by the encoder to generate a mathematical representation of the audio. This representation is then decoded using a language model to predict the most likely sequence of text tokens.Multilingual Support and Translation
Whisper is trained on a vast dataset of 680,000 hours of multilingual, supervised data, with about one-third of this data being non-English. This training enables Whisper to transcribe speech in multiple languages and translate non-English languages into English. The model uses special tokens to direct the decoder to perform tasks such as language identification and translation.Language Identification and Timestamps
Whisper can identify the language of the input speech and provide phrase-level timestamps. Special tokens are used to specify tasks such as `<|transcribe|>` or `<|translate|>`, and to indicate whether timestamps are present or not. If timestamps are requested, the decoder predicts them relative to the segment, quantized to 20 ms intervals.Voice Activity Detection
The model includes voice activity detection, indicated by the `<|nospeech|>` token. This helps in identifying segments of the audio where there is no speech, enhancing the accuracy of the transcription.Contextual Understanding
Whisper’s Transformer architecture allows it to keep track of how multiple words and sentences relate to each other, enabling it to consider long-range dependencies and contextualize words. This capability helps in filling gaps in the transcript based on the broader context of the sentences transcribed.Special Tokens for Task Direction
Whisper uses various special tokens to direct the decoder to perform specific tasks. These include tokens for language identification, task specification (e.g., `<|startoftranscript|>` and `<|endoftranscript|>`), and voice activity detection. These tokens ensure that the model can handle multiple tasks within a single framework.Fine-Tuning and Optimization
Whisper can be optimized and fine-tuned for specific tasks and domains. For example, it can be made more sensitive to industry-specific jargon and terms, or fine-tuned to recognize new languages, dialects, and accents. This flexibility makes Whisper versatile for various use cases, such as live-streaming transcription and speaker diarization.Applications
The model has diverse applications, including transcribing meetings, converting educational materials into text, enabling voice assistants, and automatic captioning. It enhances accessibility and communication between humans and machines, making it a valuable tool across various industries.Conclusion
In summary, Whisper’s integration of AI through its Transformer architecture and large, diverse training dataset makes it highly accurate and versatile for a wide range of speech recognition tasks. Its ability to handle multiple languages, provide timestamps, and detect voice activity further enhances its utility in real-world applications.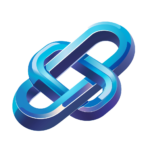
Whisper (by OpenAI) - Performance and Accuracy
When Evaluating OpenAI’s Whisper in the Speech-to-Text Category
Accuracy and Error Rates
Whisper is renowned for its high accuracy in speech-to-text transcription. It achieves a word error rate (WER) of 7.88% for the large-v3
model and 7.75% for the turbo
model, which is competitive with other top models like Universal-2.
- In a comparison with Google’s Speech-to-Text API, Whisper demonstrated a significant reduction in errors, with 45% fewer corrections per transcription.
- Whisper also excels in alphanumeric transcription, with the
large-v3
model achieving a 3.84% Alphanumerics WER, outperforming other models in this category.
Handling Diverse Languages and Acoustic Conditions
Whisper is highly adaptable to diverse languages and challenging acoustic conditions. It supports 99 languages, although it is more biased towards English due to the majority of its training data being in English.
- The model performs well in noisy and multilingual audio environments, making it versatile for various applications.
Limitations
Real-Time Transcription
Whisper lacks real-time transcription capabilities, making it unsuitable for live customer support, media broadcasts, or legal use cases that require immediate transcription.
Resource Requirements
Running Whisper, especially the large-v3
model, is resource-intensive, requiring significant GPU power and memory. This can be costly when scaling up to handle large transcription volumes.
Advanced Features
Whisper does not offer advanced features such as speaker diarization, noise reduction, or PII/PCI redaction, which are often necessary in professional environments. These features would need to be implemented separately.
File Size Limitations
Whisper has a file size limit of 25MB per audio file and a maximum duration of 30 seconds, which can complicate the workflow for handling large audio files.
Maintenance and Cost
While Whisper is open-source, maintaining it at scale can be expensive due to the need for powerful hardware, AI expertise, and ongoing server costs. This can exceed $300,000 annually for businesses transcribing hundreds of hours of audio each month.
Areas for Improvement
- Fine-Tuning for Specific Use Cases: Whisper may require fine-tuning to achieve consistently accurate results in business environments, especially for non-English languages and accents.
- Additional Features: Integrating features like real-time transcription, speaker diarization, and noise reduction would enhance Whisper’s usability in more demanding applications.
In summary, Whisper is a highly accurate speech-to-text model with strong performance in various conditions, but it comes with significant resource requirements and lacks some advanced features necessary for certain professional use cases.
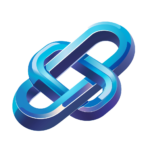
Whisper (by OpenAI) - Pricing and Plans
The Pricing Structure for OpenAI’s Whisper Model
The pricing structure for OpenAI’s Whisper model, an AI-driven speech-to-text tool, can be outlined as follows:
Usage Through Azure Services
When using the Whisper model via Azure services, the pricing is as follows:
- Azure Speech Batch with Whisper model: $0.36 per hour.
- Whisper in Azure OpenAI Service: Also $0.36 per hour.
There are volume discounts available:
- 20% discount for 2,000 hours.
- 35% discount for 10,000 hours.
- 50% discount for 50,000 hours.
Direct Usage Through OpenAI
For direct usage through OpenAI’s API, you need to refer to OpenAI’s pricing page, as specific details are not provided in the general FAQs. However, here are some key points:
- The Whisper API is no longer free in the playground since March 1st, 2023.
Free Option – Open Source
Whisper is also available as an open-source project, which means it is free to use, distribute, and modify. However, this requires technical expertise to download and run the code from the GitHub repository. There are no subscription fees, but it will require time and resources to install and use the software.
Key Features
- Languages Supported: Whisper supports transcription in multiple languages, with varying levels of accuracy. It can transcribe and translate up to 99 languages into English.
- File Formats: Supported file formats include m4a, mp3, webm, mp4, mpga, wav, and mpeg. Files must be under 25 MB in size.
- Usage Scenarios: Whisper is suitable for various applications such as transcribing class notes, meetings, podcasts, and adding subtitles to videos.
In summary, while there is a free open-source option that requires technical setup, the primary commercial usage is priced at $0.36 per hour with volume discounts, and specific pricing details for the OpenAI API should be checked on their pricing page.
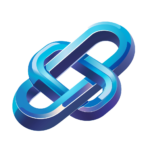
Whisper (by OpenAI) - Integration and Compatibility
The OpenAI Whisper Model
The OpenAI Whisper model, a state-of-the-art speech-to-text and translation tool, integrates seamlessly with various platforms and devices, offering a range of features and compatibility options.
Programming Languages and Frameworks
Whisper API supports integration with popular programming languages such as Python, JavaScript, and TensorFlow. This makes it easy to incorporate into existing applications and workflows. For example, you can perform a simple transcription using the OpenAI Whisper API with less than six lines of code, which is straightforward and involves minimal complexity.
Cloud Services
Whisper can be accessed through the Azure OpenAI Service or Azure AI Speech. When using the Azure OpenAI Service, it is ideal for quickly transcribing audio files one at a time, translating audio from other languages into English, and providing prompts to guide the output. It supports file formats like mp3, mp4, mpga, m4a, wav, and webm. On the other hand, the Azure AI Speech service is better for transcribing large batches of audio files, handling files larger than 25MB (up to 1GB), and supporting diarization to distinguish between different speakers.
Language Support and Customization
Whisper supports transcription and translation in multiple languages, with support for 98 languages, although only those with a word error rate (WER) below 50% are listed as supported. Users can fine-tune the model for specific languages, accents, or specialized jargon, which enhances its versatility.
File Size and Format
The Whisper API has a default file size limit of 25 MB, but you can handle larger files by breaking them into chunks or using compressed audio formats. For larger files, the Azure AI Speech service can handle files up to 1 GB.
Regional Availability
The Whisper model is available in various regions depending on whether you use the Azure OpenAI Service or Azure AI Speech. For Azure OpenAI, it is available in regions such as East US 2, India South, and West Europe, among others. For Azure AI Speech, it is available in regions like Australia East, East US, and UK South.
Integration with Other Services
Whisper integrates well with existing workflows and other software platforms. For instance, it can be used within the Google Cloud Platform ecosystem, although the setup might require additional configuration steps. The API documentation is clear and easy to navigate, making integration straightforward.
Specific Use Cases
In addition to general speech-to-text applications, Whisper can be integrated into specialized systems. For example, the Whisper Hearing System, though not directly related to the OpenAI Whisper model, demonstrates how AI-driven speech recognition can be integrated into medical devices like hearing aids, showing the potential for similar integrations in other fields.
Conclusion
Overall, the OpenAI Whisper model offers flexible integration options, making it a versatile tool for various speech recognition and translation needs across different platforms and devices.
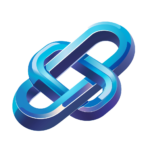
Whisper (by OpenAI) - Customer Support and Resources
Customer Support and Additional Resources for OpenAI’s Whisper
Documentation and Guides
OpenAI provides extensive documentation and guides to help users get started with Whisper. This includes detailed explanations of how Whisper works, its architecture, and how to use it for various tasks such as speech-to-text transcription, multilingual speech recognition, and speech translation.Community Resources
There is a vibrant community around Whisper, with numerous resources available on platforms like GitHub. Here, you can find a curated list of projects, tutorials, and applications built using Whisper. This includes tutorials on how to run Whisper, create speech-to-text applications, and integrate Whisper with other tools and frameworks.Model Variants and Implementations
Several model variants and implementations of Whisper are available, which can be useful for different use cases. For example, there are versions optimized for speed, such as Faster Whisper and Whisper JAX, as well as implementations in different programming languages and frameworks like C , Python, and JAX.Practical Applications and Tools
Whisper has been integrated into various practical applications, such as tools for generating subtitles for videos and podcasts, real-time transcription in call centers, and automatic speech recognition apps for mobile devices. These tools often come with user-friendly interfaces and APIs that make it easier to integrate Whisper into different systems.Performance Metrics and Benchmarks
For those interested in the technical performance of Whisper, there are detailed benchmarks available. These benchmarks compare Whisper’s performance against other ASR systems using datasets like Common Voice and LibriSpeech, providing insights into its accuracy and reliability.Customization and Fine-Tuning
Whisper allows for significant customization and fine-tuning to adapt to specific domains, languages, and accents. This flexibility is supported by the ability to fine-tune the model on additional data, making it suitable for a wide range of applications and industries.Limitations and Alternatives
While Whisper is highly capable, it does have some limitations, such as scalability challenges and the need for in-house AI expertise for large-scale deployment. For users who encounter these limitations, there are alternative ASR systems like Mozilla DeepSpeech, Kaldi, and commercial services from Google, Microsoft, and Amazon that might be more suitable.Conclusion
In summary, OpenAI’s Whisper is well-supported by a wealth of documentation, community resources, and practical tools, making it easier for users to implement and benefit from this advanced speech recognition technology.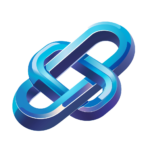
Whisper (by OpenAI) - Pros and Cons
Advantages of OpenAI Whisper
OpenAI Whisper, an open-source automatic speech recognition (ASR) system, offers several significant advantages:High Accuracy
Whisper is renowned for its high accuracy, boasting a word error rate (WER) of around 8.06%, which translates to about 92% accuracy.Multilingual Support
It can transcribe speech in multiple languages, supporting up to 99 languages, making it highly versatile for international projects.Open Source and Customizable
As an open-source model, Whisper can be modified and fine-tuned to meet specific needs, offering great flexibility for developers and researchers.Cost-Effective Initially
There are no licensing fees, making Whisper a cost-effective option for small teams or developers with technical expertise, at least initially.Adaptability to Challenging Conditions
Whisper performs well in noisy environments and with diverse speech patterns, including accents and domain-specific jargon.Disadvantages of OpenAI Whisper
Despite its advantages, Whisper also has several significant limitations:No Real-Time Transcription
Whisper is not designed for real-time transcription and is better suited for batch processing and pre-recorded audio. This makes it unsuitable for live events, customer support, or legal use cases requiring immediate transcription.High Resource Requirements
Running Whisper, especially the larger models like Large-v3, is resource-intensive, requiring significant GPU power and memory. This can be costly in terms of infrastructure, particularly when scaling up.Limited Features
Whisper lacks advanced features such as speaker diarization, noise reduction, and PII/PCI redaction, which are often necessary in professional environments. These features would need to be implemented separately.File Size Limitations
Whisper has a file size limit of 25MB per audio file, which means large audio files need to be split into smaller chunks, adding complexity to the workflow.Total Cost of Ownership
While Whisper is free to use initially, the cost of maintaining and scaling it can be high. This includes investments in powerful hardware, hiring AI specialists, and managing ongoing server costs, which can exceed $300k annually for large-scale transcription needs.Hallucinations and Fabrications
Whisper has been observed to sometimes invent text or entire sentences, known as hallucinations, which can be problematic, especially in critical applications. These fabrications can include harmful content and occur at a rate of about 1-2%. By considering these points, you can make an informed decision about whether Whisper is the right fit for your specific speech-to-text needs.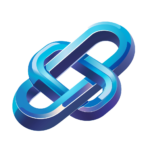
Whisper (by OpenAI) - Comparison with Competitors
When Comparing OpenAI’s Whisper with Other Speech-to-Text Tools
Several key aspects stand out, including its unique features, accuracy, and potential alternatives.
Unique Features of Whisper
- Transformer Architecture: Whisper uses an end-to-end deep learning model based on a Transformer architecture, which allows it to capture long-range dependencies and contextualize words more effectively than traditional speech recognition models.
- Multilingual Support and Translation: Whisper can transcribe and translate speech in multiple languages, including language identification and phrase-level timestamps. It also supports speech-to-English translation, making it versatile for global applications.
- Noise Reduction and Error Correction: Whisper is notable for its ability to handle noisy environments and correct minor speech errors, such as stumbles and mispronunciations, ensuring accurate transcriptions even in challenging audio settings.
- Open Source: Whisper is open source, which allows developers to modify and improve the model, unlocking a wide range of potential applications and improvements.
Comparison with Competitors
Google Cloud Speech-to-Text
- Accuracy and Language Support: Google Cloud Speech-to-Text offers high accuracy and supports over 120 languages, but it may be more expensive and requires a stable internet connection. Unlike Whisper, it does not have the same level of noise reduction and error correction capabilities.
- Integration: Google Cloud Speech-to-Text may require more technical expertise for integration compared to Whisper, which can be more straightforward due to its open-source nature.
Microsoft Azure Speech Service
- Accuracy and Real-time Processing: Microsoft Azure Speech Service also offers high accuracy and real-time processing, but its language support is moderate compared to Whisper’s extensive multilingual capabilities.
- Pricing: The pricing model for Azure can be variable, which might be less predictable than Whisper’s costs, especially if you are using it through third-party providers.
Deepgram
- Accuracy and Features: Deepgram claims higher accuracy and richer features compared to Whisper, including lower operating costs and faster processing speeds. However, Whisper’s open-source nature and broader community support can be a significant advantage.
- Cost and Speed: Deepgram’s pricing and speed factors vary, but it generally offers competitive rates and fast processing times, similar to Whisper’s performance metrics.
Speechmatics
- Language Support and Customization: Speechmatics offers wide language support and customization options, similar to Whisper. However, its API can be complex to integrate, and the pricing structure may be more complicated.
- Accuracy: Speechmatics has high accuracy, but its performance in noisy environments might not match Whisper’s advanced noise reduction capabilities.
Amazon Transcribe
- Accuracy and Integration: Amazon Transcribe has moderate accuracy and good integration capabilities, but its language support is limited compared to Whisper. It also lacks real-time processing, which is a key feature of Whisper.
Potential Alternatives
- Deepgram: For those seeking higher accuracy and additional features, Deepgram could be a viable alternative. It offers richer features, lower operating costs, and faster processing speeds, although it may not have the same level of community support as Whisper.
- Speechmatics: If customization and extensive language support are critical, Speechmatics could be an alternative. However, it may require more technical expertise for integration and has a more complex pricing structure.
- Google Cloud Speech-to-Text: For applications requiring high accuracy and extensive language support with a more established platform, Google Cloud Speech-to-Text is a strong option, despite its potential higher costs and internet dependency.
Conclusion
In summary, Whisper stands out due to its advanced Transformer architecture, multilingual support, noise reduction capabilities, and open-source nature. While competitors like Deepgram, Speechmatics, and Google Cloud Speech-to-Text offer strong alternatives with unique strengths, Whisper’s overall package makes it a compelling choice for many speech-to-text applications.
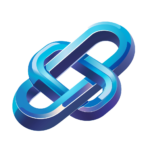
Whisper (by OpenAI) - Frequently Asked Questions
What is OpenAI Whisper?
OpenAI Whisper is an automatic speech recognition (ASR) system that transcribes speech into text and translates speech from various languages to English. It is based on an end-to-end deep learning model using an encoder-decoder Transformer architecture.
What are the key features of Whisper?
Whisper can transcribe speech into text, translate speech from multiple languages to English, and perform tasks like language identification, phrase-level timestamps, and speaker diarization. It can also be fine-tuned for specific tasks, such as recognizing industry-specific jargon and handling different accents and dialects.
How was Whisper trained?
Whisper was trained on a vast dataset of 680,000 hours of multilingual and multitask supervised data collected from the internet and academic resources. About one-third of this data (117,000 hours) is multilingual, enabling transcription and translation in 99 languages.
What file formats and sizes are supported by the Whisper API?
The Whisper API supports file formats such as m4a, mp3, webm, mp4, mpga, wav, and mpeg. The file size limit is 25 MB. If the file is larger than 25 MB, you need to follow the guidance on handling long inputs.
Is the transcription received in a streaming style?
No, the transcription is not received in a streaming style. You need to upload the entire audio file for transcription.
Can I send links to audio files instead of uploading them?
No, you cannot send links to audio files. You must upload the audio file in one of the supported formats.
How does Whisper handle background noise and speech errors?
Whisper uses powerful algorithms to focus on the important parts of the speech, reducing the impact of background noise and correcting minor speech errors like stumbles and mispronunciations. This makes it effective in noisy environments.
What is the role of prompts in Whisper?
Prompts can help stitch together multiple audio segments by providing context from prior segments. You can also use fictitious prompts to steer the model to use specific spellings or styles. However, prompts are limited to 224 tokens, and any longer prompts will only consider the last 224 tokens.
Is Whisper free to use?
Starting March 1st, 2023, Whisper is no longer free in the OpenAI playground. You need to refer to the pricing page for details on using the Whisper API.
What are some potential applications of Whisper?
Whisper can be used in various applications such as transcription services, voice assistants, customer service, accessibility tools for the hearing-impaired, medical and legal transcriptions, and more.
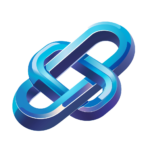
Whisper (by OpenAI) - Conclusion and Recommendation
Final Assessment of OpenAI Whisper
OpenAI Whisper is a highly advanced speech-to-text (STT) model that has revolutionized the field of speech recognition. Here’s a comprehensive overview of its features, benefits, and who would most benefit from using it.
Key Features
- Whisper is built on an end-to-end deep learning model using a Transformer architecture, which allows it to capture long-range dependencies and contextualize speech accurately.
- It can transcribe speech into text and translate speech from various languages to English, making it highly versatile for multilingual applications.
- The model is trained on a vast dataset of 680,000 hours of supervised data, including 117,000 hours of multilingual data, enabling it to support 99 languages, many of which are low-resource languages.
Accuracy and Reliability
- Whisper stands out for its high accuracy and reliability, even in noisy environments. It uses advanced algorithms for noise reduction and error correction, ensuring that it captures the essence of what is being said despite background noise or speech errors.
Practical Use Cases
- Whisper can be used in various applications such as transcribing customer calls in real-time to enhance customer service, indexing podcasts and audio content for better accessibility and searchability, and conducting automated market research by analyzing customer feedback.
- It is also useful for live-streaming transcription, speaker diarization, and recognizing industry-specific jargon and terms after fine-tuning.
Who Would Benefit Most
- Businesses and Enterprises: Companies can leverage Whisper to improve customer service by transcribing and analyzing customer calls, gathering insights from customer feedback, and enhancing their products and services.
- Content Creators: Podcasters and audio content creators can use Whisper to generate text-based versions of their content, improving accessibility and searchability.
- Developers: Developers can integrate Whisper into various applications, such as virtual assistants, hands-free controls, and speech analytics, due to its open-source nature and the ability to fine-tune it for specific tasks.
- Individuals with Hearing Impairments: Whisper can significantly improve accessibility by providing accurate transcriptions of spoken content, helping individuals with hearing impairments to engage more fully with audio materials.
Recommendation
Given its accuracy, versatility, and wide range of applications, OpenAI Whisper is highly recommended for anyone looking to integrate advanced speech-to-text capabilities into their projects or operations. Its ability to handle multiple languages, accents, and noisy environments makes it a valuable tool for both commercial and personal use. However, it is important to note that fine-tuning and optimization may be necessary to fully adapt Whisper to specific use cases, which could require additional resources and expertise.