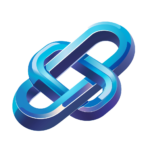
Text-To-4D - Detailed Review
Video Tools
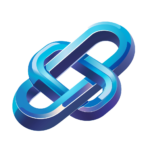
Text-To-4D - Product Overview
Text-To-4D Overview
Primary Function
Text-To-4D is an AI-driven tool focused on generating dynamic, animated 3D scenes from natural-language descriptions. This technology combines the capabilities of video and 3D generative models to produce 4D content, which includes both 3D geometry and time dimensions.
Target Audience
The primary users of Text-To-4D include professionals in digital content creation, such as those in the video game industry, visual effects, augmented and virtual reality (AR/VR), and advertising. This tool is particularly useful for animators, 3D artists, and anyone looking to generate animated 3D assets efficiently.
Key Features
Dynamic 3D Scene Generation
Text-To-4D allows users to generate dynamic 3D scenes from text prompts. This involves creating 3D objects that can change over time, capturing both the spatial and temporal aspects of the scene.
Compositional Generation Framework
The method, such as the one proposed in “Align Your Gaussians” (AYG), combines text-to-image, text-to-video, and 3D-aware multiview diffusion models to ensure high-quality visual appearance, realistic geometry, and temporal consistency.
Score Distillation and Diffusion Models
The system leverages score distillation methods and diffusion models to optimize the 4D object generation. This includes using text-to-video models to capture temporal dynamics and text-to-image models to maintain high visual quality across all time frames.
Motion Amplification and Regularization
Techniques like motion amplification and various motion regularizers are used to enhance the realism of the generated motion and ensure smooth 4D sequences.
Autoregressive Generation
Text-To-4D can extend the length of 4D sequences and combine different dynamic scenes with changing text guidance using an autoregressive generation scheme.
Multi-Resolution and High-Quality Rendering
The method supports multi-resolution feature grids and super-resolution fine-tuning to improve the resolution and quality of the generated scenes.
By integrating these features, Text-To-4D simplifies the process of creating dynamic 3D content, making it more accessible and efficient for a wide range of applications.
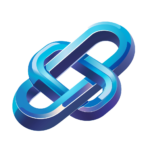
Text-To-4D - User Interface and Experience
User Interface
A user interface for a Text-to-4D generation tool would likely be designed to be intuitive and user-friendly. Here are some key elements it might include:Text Prompt Input
A clear and prominent text field where users can input their text prompts or descriptions of the 4D scenes they want to generate.Customization Options
Drop-down menus, checkboxes, or sliders to allow users to customize various aspects such as styles, themes, and specific details of the 4D generation (e.g., dynamic motions, geometry, and texture).Preview and Generation Buttons
Simple and clearly labeled buttons to initiate the generation process and preview the results.Feedback and Error Messages
User-friendly feedback mechanisms to inform users about the status of their generation requests and any errors that might occur.Ease of Use
For a tool to be easy to use, it should follow these guidelines:Clear Instructions
The interface should provide clear instructions or tooltips to help users understand what each option does.Minimal Steps
The process of generating a 4D scene should be streamlined, requiring minimal steps from the user.Real-time Feedback
The system should provide real-time feedback as the user inputs their text and customizes their options, helping them see how their choices affect the output.Overall User Experience
The overall user experience would be enhanced by:Responsive Design
The interface should be responsive and work well on various devices, ensuring that users can generate 4D scenes whether they are using a desktop, laptop, or mobile device.Iterative Process
Allowing users to easily make changes and see the results in real-time can make the iteration process smoother and more engaging.Help Resources
Providing accessible help resources, such as tutorials or FAQs, can assist users who encounter issues or need further guidance.Example from Similar Tools
Tools like Stable Video and Runway ML, which generate videos from text prompts, offer insights into what a user-friendly interface might look like. These tools typically include:Simple Text Input Field
A simple text input field for the user’s prompt.Customization Options
Customization options for styles, themes, and other details.Preview Feature
A preview feature to review the generated video before finalizing it.Post-Generation Adjustments
Options to adjust animations, transitions, and other elements post-generation. Since the specific website you mentioned does not have detailed information available in the sources I accessed, these general principles and examples from similar tools provide a framework for what a user interface for a Text-to-4D generation tool might aim to achieve.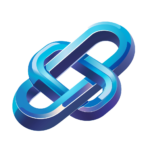
Text-To-4D - Key Features and Functionality
The Text-To-4D Generation Technology
The Text-To-4D generation technology, exemplified by Meta AI’s “Make-A-Video3D” (MAV3D) model, incorporates several key features that make it a powerful tool for creating dynamic 3D scenes from text prompts.
Text-to-Video Prior
One of the primary features is the use of a text-to-video diffusion model to generate a reference video. This video serves as a direct prior for the 4D generation process, ensuring the dynamic amplitude and authenticity of the generated content. The reference video is used to guide both the static 3D generation and the dynamic generation stages.
Two-Stage Generation
The 4D generation process is divided into two stages:
Static 3D Generation
This stage uses the input text and the first frame of the reference video to generate a static 3D model. It employs joint supervision from 2D and 3D Score Distillation Sampling (SDS) losses to ensure diversity and 3D consistency.
Dynamic Generation
In this stage, the model introduces a customized SDS loss to maintain multi-view consistency and a video-based SDS loss to improve temporal consistency. Direct priors from the reference video are used to enhance the quality of geometry and texture.
Prior-Switching Training Strategy
To avoid conflicts between different priors and fully leverage their benefits, the model uses a prior-switching training strategy. This approach allows the model to switch between different priors during training, ensuring that each prior contributes effectively to the generation process.
Dynamic Modeling Representation
The model includes a dynamic modeling representation composed of a deformation network and a topology network. This ensures dynamic continuity while modeling topological changes, enriching the generated motion and maintaining coherence across different views and time.
Multi-Stage Optimization
The MAV3D model employs a multi-stage static-to-dynamic optimization scheme. This involves several motion regularizers to encourage realistic motion and improve model convergence. Additionally, super-resolution fine-tuning (SRFT) is used to enhance the resolution of the generated scenes.
Flexible 4D Scene Representation
The model uses a new 4D scene representation that allows for flexible modeling of scene motion. This representation is crucial for capturing the dynamic changes in the scene over time and from various viewpoints.
User Interaction and Customization
Users can generate dynamic 3D scenes by providing natural-language descriptions. The system can also be used to generate animated 3D assets for applications such as video games, visual effects, augmented reality, and virtual reality. The ability to view generated scenes from arbitrary viewpoints enhances user interaction and customization.
Integration with Other Technologies
The Text-To-4D technology builds on the foundation of video representation learning and generative architectures, making text-to-video generation models a natural bridge between 2D video and 3D/4D scene generation. This integration enables more precise control over video elements like objects, motion, lighting, and narrative structure, which is beneficial for creative industries and healthcare applications.
These features collectively enable the creation of highly realistic and dynamic 3D scenes from text prompts, making the Text-To-4D technology a significant advancement in AI-driven content creation.
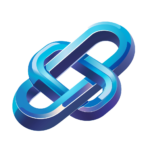
Text-To-4D - Performance and Accuracy
Evaluating the Performance and Accuracy of Text-To-4D Generation
Evaluating the performance and accuracy of Text-To-4D generation involves examining several key aspects, particularly from the research papers and methodologies described.
Performance Metrics
Text-To-4D generation methods, as outlined in the research, are evaluated on several metrics:
Appearance Quality (AQ)
This metric assesses how visually appealing the generated 4D scenes are. Studies like the one on TC4D show that users significantly prefer the results from TC4D over other methods in terms of appearance quality.
3D Structure Quality (SQ)
This evaluates the structural integrity and coherence of the generated 3D scenes. While TC4D performs well in many areas, it does not always show a statistically significant preference over other methods in structure quality.
Motion Quality (MQ) and Motion Amount (MA)
These metrics are crucial for assessing the realism and amount of motion in the generated scenes. TC4D and other advanced methods, such as 4Dynamic, introduce techniques like local deformation models and customized SDS losses to ensure high-quality and realistic motion. These methods are generally preferred by users for their motion quality and amount.
Optimization and Generation Stages
The performance of Text-To-4D generation is significantly improved through multi-stage optimization schemes:
Static-to-Dynamic Optimization
This involves first generating a static 3D scene using a Text-to-Image model and then augmenting it with dynamic elements. This approach helps in achieving high-quality results and avoiding visual artifacts.
Temporal-Aware Super-Resolution Fine-Tuning
This stage enhances the resolution of the generated videos, ensuring high-resolution outputs that are crucial for detailed and realistic scenes.
Limitations and Areas for Improvement
Despite the advancements, there are several limitations and areas that need improvement:
Temporal Consistency
One of the significant challenges is maintaining temporal consistency, especially when dealing with multiple frames and long videos. Methods like GPT4V struggle with describing videos accurately as the number of frames increases, leading to temporal confusion.
Data Scarcity and Annotation
Creating large-scale, high-quality video captions is time-consuming and challenging, even for humans. This limits the training data available for these models, which can impact their performance.
Realism and Dynamic Motions
Ensuring the realism and authenticity of dynamic motions remains a challenge. Methods like 4Dynamic address this by using video priors and customized SDS losses, but there is still room for improvement in achieving more realistic and diverse motions.
User Preference and Engagement
User studies play a crucial role in evaluating the performance of Text-To-4D generation. For instance, TC4D was preferred by 85% of users in overall comparisons, indicating high engagement and satisfaction with the generated scenes.
In summary, while Text-To-4D generation has made significant strides in terms of appearance quality, motion realism, and user preference, it still faces challenges related to temporal consistency, data annotation, and achieving highly realistic dynamic motions. Addressing these areas will be key to further improving the performance and accuracy of these models.
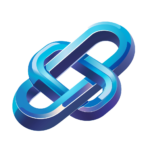
Text-To-4D - Pricing and Plans
Current Pricing Information
As of the current information available, there is no detailed pricing structure or plans outlined for the Text-To-4D model associated with the Make-A-Video3D project.
Technical and Functional Aspects
The resources provided focus on the technical and functional aspects of the model, such as its capabilities in generating dynamic 3D scenes from text descriptions, but do not include any information on pricing or subscription plans.
Contact for Specific Pricing Details
If you are looking for specific pricing details, it is recommended to contact the developers or the organization behind the Make-A-Video3D project directly, as this information may not be publicly available at this time.
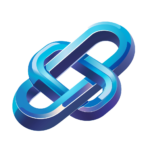
Text-To-4D - Integration and Compatibility
Integration and Compatibility of Text-To-4D Technologies
The integration and compatibility of Text-To-4D technologies, such as those developed by Meta AI (MAV3D) and other similar frameworks, can be analyzed from several perspectives:Integration with Other Tools
Text-To-4D generation models, like MAV3D, are built on the foundation of existing generative models for images and videos. These models integrate pre-trained text-to-image and text-to-video models to generate dynamic 3D scenes. For instance, MAV3D combines the benefits of video and 3D generative models by using a pre-trained text-to-video (T2V) model and enhancing it with 4D scene representation and multi-stage optimization schemes.Compatibility Across Platforms
While the specific platforms and devices compatible with Text-To-4D tools are not explicitly detailed in the available resources, here are some general insights:Rendering and Viewing
The generated 3D scenes can be rendered from arbitrary viewpoints, suggesting compatibility with various rendering engines and 3D visualization tools. This flexibility makes it possible to view the generated scenes on different devices that support 3D rendering, such as computers, VR headsets, and potentially mobile devices with 3D capabilities.Training and Development
The training process for these models often involves large datasets and significant computational resources. This implies that the development and training of these models are likely to be done on high-performance computing environments or cloud services that support GPU acceleration. Once trained, the models can be deployed on a variety of platforms, depending on the specific implementation and optimization.Open-Source Availability
Some of these models, such as the 4D-fy method, are planned to be made publicly available along with their codes and evaluation procedures. This open-source approach facilitates integration with other tools and platforms, as developers can adapt and optimize the models for their specific needs.Cross-Device Compatibility
Given the nature of the generated content (dynamic 3D scenes), compatibility would depend on the device’s ability to handle 3D graphics and video rendering. Here are a few points to consider:Desktop and Laptop Computers
These devices are likely to be fully compatible, given their typical hardware capabilities for handling 3D graphics and video rendering.Mobile Devices
Compatibility may vary depending on the device’s hardware specifications, particularly the GPU and processing power. High-end mobile devices with advanced GPUs could potentially handle the rendering of these 3D scenes.VR and AR Devices
These devices are well-suited for viewing dynamic 3D scenes and would likely be compatible, given their specialized hardware for 3D rendering and immersive experiences. In summary, while specific details on platform and device compatibility are limited, the integration of Text-To-4D technologies with other tools is facilitated by their foundation on existing generative models. The compatibility across different devices will largely depend on the device’s capability to handle 3D graphics and video rendering.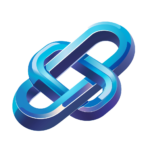
Text-To-4D - Customer Support and Resources
The Text-To-4D Technology
The Text-To-4D technology, as represented by the MAV3D (Make-A-Video3D) method, is primarily focused on generating dynamic 3D scenes from text descriptions. This technology is geared more towards creative and technical applications such as video games, visual effects, and augmented or virtual reality, rather than customer support.
Customer Support Options
There is no specific information available on customer support options provided by the Text-To-4D technology. The resources and documentation available are more technical and oriented towards developers and users interested in generating 3D dynamic scenes, rather than providing customer service.
Additional Resources
Technical Documentation
The resources provided include detailed technical papers and explanations on how the MAV3D method works, including the multi-stage optimization scheme and the use of score distillation methods.
Generated Samples
Users can view generated samples of dynamic 3D scenes created from text descriptions on the project’s website.
Community and Forums
While not explicitly mentioned, users might find community forums or discussion groups related to the broader field of AI-generated content where they can ask questions and share knowledge.
Summary
In summary, the Text-To-4D technology does not offer traditional customer support options like those found in customer service systems. Instead, it provides technical resources and examples for users interested in the generation of dynamic 3D scenes.
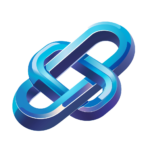
Text-To-4D - Pros and Cons
Advantages of Text-To-4D Generation
Realistic and Dynamic Scenes
Text-To-4D generation, as seen in methods like MAV3D and 4Dynamic, allows for the creation of highly realistic and dynamic 3D scenes from natural language prompts. These scenes can be rendered from arbitrary viewpoints, making them versatile for various applications such as video games, visual effects, and augmented and virtual reality.
Multi-Stage Optimization
The MAV3D method employs a multi-stage static-to-dynamic optimization scheme, which includes motion regularizers to encourage realistic motion and super-resolution fine-tuning to improve the resolution of the generated scenes. This approach enhances video quality and aids in model convergence.
Hybrid Priors and Supervision
The 4Dynamic method uses hybrid priors, including a text-to-video diffusion model to generate a reference video, which guides the static and dynamic generation stages. This ensures dynamic amplitude and authenticity, improving the realism and temporal consistency of the generated scenes.
Dynamic Modeling and Topological Changes
Techniques like those in 4Dynamic and Text-to-4D with Dynamic 3D Gaussians incorporate dynamic modeling representations, such as deformation networks and topology networks. These ensure dynamic continuity and model topological changes, enriching the generated motion and maintaining high visual quality across all time frames.
Versatility in Input
These methods can generate 4D scenes not only from text but also from monocular videos, expanding their applicability in different scenarios.
Disadvantages of Text-To-4D Generation
Data Scarcity
One of the significant challenges is the lack of readily available collections of 4D models with textual annotations. This scarcity makes training and validating these models more difficult compared to 2D image and video generation.
Technical Challenges
Reconstructing the shape of deformable objects from video is highly challenging. This requires innovative solutions, such as distilling 4D reconstructions from generated videos or using customized loss functions to ensure multi-view and temporal consistency.
Computational Requirements
Generating high-quality 4D scenes involves complex processes, including multi-stage optimization, super-resolution fine-tuning, and the use of multiple models (e.g., text-to-video and text-to-image models). These processes can be computationally intensive and may require significant resources.
Optimization Stability
Ensuring stable optimization during the generation process is crucial but challenging. Methods often need to employ novel regularization techniques to maintain stability and achieve vivid dynamic scenes.
In summary, while Text-To-4D generation offers the ability to create realistic and dynamic 3D scenes with various applications, it faces challenges related to data availability, technical complexity, and computational demands.
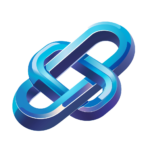
Text-To-4D - Comparison with Competitors
When comparing the Text-To-4D dynamic scene generation tool, such as MAV3D (Make-A-Video3D), with other AI-driven video tools in the same category, several unique features and potential alternatives stand out.
Unique Features of MAV3D
- 4D Dynamic Scene Generation: MAV3D is distinct in its ability to generate three-dimensional dynamic scenes from text descriptions, incorporating both 3D space and time. This allows for the creation of animated 3D assets that can be rendered from arbitrary viewpoints, which is particularly useful for applications in video games, visual effects, augmented reality, and virtual reality.
- Multi-Stage Optimization: MAV3D uses a multi-stage static-to-dynamic optimization scheme, which includes a Text-to-Image (T2I) model to fit a static 3D scene to a text prompt, followed by augmenting the 3D scene model with dynamics. This process is enhanced by a new temporal-aware Score Distillation Sampling (SDS) loss and motion regularizers to ensure realistic motion.
- Super-Resolution Fine-Tuning: The tool also employs super-resolution fine-tuning to improve the resolution of the generated scenes, making the output more detailed and high-quality.
Potential Alternatives
Text-to-Video Tools
While not specifically focused on 4D scene generation, several text-to-video tools offer impressive capabilities that might be considered as alternatives or complementary tools:
- Veed: Veed is excellent for generating complete videos with AI, including voiceovers, music, and footage. It guides users through the process step-by-step and offers various style options. However, it does not generate 3D dynamic scenes but is great for creating entire videos from text prompts.
- Sora: Sora, by OpenAI, generates entire scenes from simple text prompts using a storyboard feature. It is good for creating dreamy, atmospheric content but may struggle with human and animal movements. Sora does not generate 4D scenes but is useful for creating coherent and visually appealing videos.
- Synthesia: Synthesia focuses on creating studio-quality videos with AI avatars and supports over 140 languages. It is ideal for training videos, internal communications, and marketing but does not generate 3D or 4D scenes.
Other 3D/4D Generation Tools
- General Text-to-Video Editing: Tools like those developed by the Harvard AI Robotics Lab focus on text-to-video editing and 4D scene generation. These tools allow precise modification of existing videos or creation of new ones, controlling dynamic elements like motion, lighting, and narrative flow through text prompts. While they share some similarities with MAV3D, they may not offer the same level of 4D scene generation specificity.
Key Differences
- Dimensionality: MAV3D is specifically designed for generating dynamic 3D scenes, which is a unique feature compared to most text-to-video tools that focus on 2D video generation.
- Customization and Control: MAV3D’s multi-stage optimization and super-resolution fine-tuning provide a high level of control and customization over the generated scenes, which may not be as detailed in other text-to-video tools.
- Application Focus: While other tools are more geared towards general video creation, marketing, and communication, MAV3D is tailored for applications requiring detailed 3D dynamic scenes, such as video games and virtual reality.
In summary, MAV3D stands out for its specialized capability in generating dynamic 3D scenes from text descriptions, making it a valuable tool for specific use cases that require this level of detail and realism. However, for more general video creation needs, tools like Veed, Sora, and Synthesia might be more suitable alternatives.
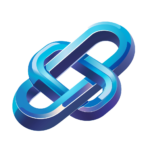
Text-To-4D - Frequently Asked Questions
Here are some frequently asked questions about the Text-To-4D system, specifically the MAV3D method, along with detailed responses:
What is Text-To-4D and how does it work?
Text-To-4D, using the MAV3D method, is a system that generates three-dimensional dynamic scenes from text descriptions. It combines the benefits of video and 3D generative models by utilizing a 4D dynamic Neural Radiance Field (NeRF) optimized for scene appearance, density, and motion consistency. This is achieved by querying a Text-to-Video (T2V) diffusion-based model.What is the role of Neural Radiance Fields (NeRF) in Text-To-4D?
NeRF plays a crucial role in Text-To-4D by allowing the system to model 3D scenes in a way that captures their appearance, density, and motion. The 4D NeRF is optimized to ensure consistent and realistic motion in the generated dynamic scenes.How does the system handle the lack of 3D and 4D training data?
The MAV3D method does not require any 3D or 4D data for training. Instead, it relies on Text-Image pairs and unlabeled videos to train the T2V model. This approach helps bypass the data scarcity problem in 3D and 4D generation.Can the generated scenes be viewed from any angle?
Yes, the dynamic video output generated by MAV3D can be viewed from any camera location and angle. This flexibility allows users to render the scenes in various perspectives, enhancing the realism and usability of the generated content.What are the key innovations in the MAV3D method?
The MAV3D method introduces several key innovations, including a new 4D scene representation, a multi-stage static-to-dynamic optimization scheme, and super-resolution fine-tuning (SRFT). These innovations help improve video quality, model convergence, and the resolution of the generated scenes.How does the system ensure realistic motion in the generated scenes?
To ensure realistic motion, the MAV3D method uses several motion regularizers and a multi-stage optimization scheme. Additionally, it employs a customized Score Distillation Sampling (SDS) loss and video-based SDS loss to improve temporal and multi-view consistency.Can the generated scenes be integrated into other 3D environments?
Yes, the dynamic scenes generated by MAV3D can be composited into any 3D environment, making them versatile for various applications such as video games, visual effects, and augmented or virtual reality.Is there an online demo available to test the Text-To-4D system?
Yes, there is an online web demo available where users can view the generated 4D videos in different modes, including a mesh mode that allows viewing the scenes from any angle.What are the potential applications of the Text-To-4D system?
The Text-To-4D system has several potential applications, including generating animated 3D assets for video games, creating visual effects, and enhancing augmented or virtual reality experiences.How does the system handle text prompts with specific objects or actions?
The system can generate scenes based on detailed text prompts, including specific objects or actions. For example, if an object is mentioned in the text prompt, the system will include it in the generated scene and ensure it behaves according to the described action.What are the challenges faced by Text-To-4D generation?
Text-To-4D generation faces challenges such as ensuring realism and sufficient dynamic motions. The existing methods have to overcome these challenges by introducing new scene representations, optimization schemes, and supervision methods.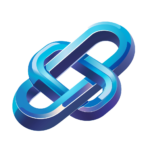
Text-To-4D - Conclusion and Recommendation
Final Assessment of Text-To-4D
Text-To-4D is a groundbreaking AI-driven tool that combines the capabilities of video and 3D generative models to create dynamic 4D scenes from text descriptions. Here’s a comprehensive assessment of its benefits and who would most benefit from using it.Key Benefits
Advanced Scene Representation
The system introduces a new 4D scene representation that allows for flexible modeling of scene motion, which is crucial for generating realistic and coherent dynamic scenes.
Multi-Stage Optimization
It employs a multi-stage static-to-dynamic optimization scheme, utilizing motion regularizers to enhance video quality and improve model convergence. This ensures more realistic motion in the generated scenes.
Super-Resolution Fine-Tuning
The tool includes a super-resolution fine-tuning (SRFT) stage to improve the resolution of the generated videos, resulting in higher quality output.
Who Would Benefit Most
Content Creators
Independent filmmakers, video producers, and content creators can significantly benefit from Text-To-4D. It democratizes the process of creating high-quality, dynamic 3D content, reducing the need for extensive technical expertise and expensive equipment.
Advertising and Marketing
Brands and marketers can use this technology to generate engaging, personalized video content quickly and efficiently. This can be particularly useful for creating dynamic ads and promotional materials that resonate with specific audience segments.
Research and Development
Researchers in the field of AI and computer vision can leverage Text-To-4D to advance their work in 4D scene generation, benefiting from the open-source code and the potential for rapid improvements in 2D video generation.
Overall Recommendation
Text-To-4D is a powerful tool for anyone looking to generate high-quality, dynamic 4D content from text descriptions. Its ability to produce realistic motion, enhance video quality, and improve resolution makes it an invaluable asset for content creators, marketers, and researchers.
Engagement and Factual Accuracy
The tool’s user study results show a significant preference for the generated scenes, with 85% of comparisons favoring Text-To-4D over other methods. This indicates high engagement and satisfaction with the output quality.
In summary, Text-To-4D is a highly recommended tool for those seeking to create dynamic, high-quality 4D content efficiently. Its innovative approach and significant improvements in scene generation make it a valuable resource in the video tools AI-driven product category.