Self Learning AI Models Transforming Automotive Research and Development
Topic: AI Self Improvement Tools
Industry: Automotive and Transportation
Discover how self-learning AI models are transforming automotive R&D and testing by enhancing data analysis efficiency and vehicle performance in this insightful article
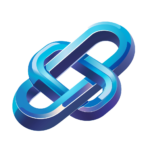
Self-Learning AI Models: Accelerating Automotive R&D and Testing
Introduction to Self-Learning AI in the Automotive Sector
As the automotive industry continues to evolve, the integration of artificial intelligence (AI) into research and development (R&D) processes has become increasingly vital. Self-learning AI models, which adapt and improve over time, are at the forefront of this transformation, enabling manufacturers to enhance their R&D and testing procedures significantly. This article explores how these advanced AI self-improvement tools can be implemented in the automotive sector and highlights specific tools and products that are leading the charge.
The Role of AI in Automotive R&D
Artificial intelligence plays a crucial role in various stages of automotive R&D, from design and simulation to testing and validation. By leveraging self-learning models, automotive engineers can streamline processes, reduce costs, and enhance vehicle performance. The ability of these models to analyze vast amounts of data and learn from it allows for more informed decision-making and faster innovation cycles.
Key Benefits of Self-Learning AI Models
- Enhanced Data Analysis: Self-learning AI can process and analyze data from multiple sources, leading to more accurate insights and predictions.
- Improved Testing Efficiency: AI-driven simulations can reduce the time and resources required for physical testing, enabling quicker iterations.
- Predictive Maintenance: By analyzing vehicle performance data, self-learning models can predict potential failures and suggest maintenance before issues arise.
- Personalized User Experience: AI can adapt vehicle systems based on driver behavior, enhancing comfort and safety.
Implementation of AI Self-Improvement Tools
Implementing self-learning AI models in automotive R&D requires a strategic approach. Here are some steps manufacturers can take to integrate these technologies effectively:
1. Data Collection and Management
Robust data collection is essential for self-learning AI models. Automotive companies should invest in advanced data management systems to gather data from various sources, including vehicle sensors, customer feedback, and market trends.
2. Choosing the Right AI Tools
Selecting appropriate AI tools is critical to successful implementation. Here are a few examples of AI-driven products that can enhance automotive R&D:
TensorFlow
TensorFlow is an open-source machine learning framework that provides tools for building and training self-learning models. Automotive engineers can use TensorFlow to develop predictive models for vehicle performance and safety features.
IBM Watson
IBM Watson offers AI solutions that can analyze large datasets, enabling automotive companies to gain insights into consumer preferences and vehicle performance. Its natural language processing capabilities can also enhance customer interactions through chatbots and virtual assistants.
Autodesk Generative Design
This tool uses AI algorithms to explore design alternatives based on specified parameters. Automotive designers can leverage generative design to optimize vehicle structures for weight, strength, and manufacturability, leading to more efficient designs.
3. Continuous Learning and Adaptation
Self-learning AI models thrive on continuous data input. Manufacturers should establish systems for ongoing data collection and model training, ensuring that AI tools evolve alongside technological advancements and changing consumer needs.
Case Studies: Successful Implementation of AI in Automotive R&D
Several automotive companies have successfully integrated self-learning AI models into their R&D processes:
Tesla
Tesla’s Autopilot feature utilizes self-learning AI to improve its autonomous driving capabilities. By analyzing data from its fleet of vehicles, the AI continuously learns from real-world driving conditions, enhancing safety and functionality over time.
Ford
Ford has implemented AI in its vehicle testing processes, using machine learning algorithms to predict outcomes based on historical data. This approach has significantly reduced the time required for testing new vehicle prototypes.
Conclusion
The automotive industry stands on the brink of a technological revolution, with self-learning AI models driving innovation in R&D and testing. By embracing these advanced tools, manufacturers can not only accelerate their development processes but also enhance vehicle performance and safety. As AI continues to evolve, the potential for improved automotive solutions is virtually limitless.
Keyword: self learning AI automotive R&D