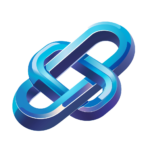
Personalized Accessibility Settings with AI Integration Workflow
Discover AI-driven personalized accessibility settings that adapt to user needs through machine learning enhancing user experience and satisfaction in telecommunications.
Category: AI Accessibility Tools
Industry: Telecommunications
Personalized Accessibility Settings Using Machine Learning
1. Define User Accessibility Needs
1.1 User Profile Creation
Collect data on individual user preferences, disabilities, and specific accessibility requirements through surveys and interviews.
1.2 Needs Assessment
Analyze collected data to identify common patterns and unique needs among users.
2. Data Collection and Preparation
2.1 Data Sources
Utilize existing databases, user feedback, and telemetry data from telecommunications devices to gather relevant information.
2.2 Data Cleaning and Structuring
Process the gathered data to ensure it is clean, structured, and ready for machine learning algorithms.
3. Machine Learning Model Development
3.1 Model Selection
Choose appropriate machine learning algorithms (e.g., decision trees, neural networks) based on the complexity of user needs.
3.2 Training the Model
Train the model using the prepared dataset to recognize patterns and predict personalized settings for users.
3.3 Testing and Validation
Conduct rigorous testing to validate the accuracy and reliability of the model’s predictions.
4. Implementation of AI-Driven Tools
4.1 Integration with Telecommunications Platforms
Integrate machine learning models with existing telecommunications systems to provide real-time accessibility adjustments.
4.2 Examples of AI-Driven Products
- Speech Recognition Software: Tools like Google Speech-to-Text can be utilized to convert spoken language into text for users with hearing impairments.
- Text-to-Speech Applications: Solutions such as Amazon Polly can transform written text into spoken words, aiding users with visual impairments.
- Customizable User Interfaces: Machine learning can be employed to adapt user interfaces in applications like Zoom or Microsoft Teams based on user preferences.
5. Continuous Learning and Improvement
5.1 User Feedback Loop
Establish a feedback mechanism to gather user experiences and suggestions for further personalization.
5.2 Model Refinement
Regularly update the machine learning models based on new data and user feedback to enhance accuracy and user satisfaction.
6. Compliance and Ethical Considerations
6.1 Accessibility Standards
Ensure that all personalized settings comply with relevant accessibility standards (e.g., WCAG) and regulations.
6.2 Data Privacy
Implement robust data protection measures to safeguard user information and maintain trust.
7. Reporting and Analytics
7.1 Performance Metrics
Track the effectiveness of personalized settings through user engagement analytics and accessibility success rates.
7.2 Reporting
Generate reports for stakeholders to demonstrate the impact of personalized accessibility settings on user experience and satisfaction.
Keyword: Personalized accessibility settings machine learning