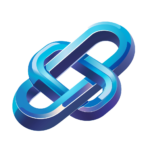
AI Driven Predictive Analytics for Market Trend Forecasting
AI-driven predictive analytics enhances market trend forecasting by defining objectives collecting data preparing models and optimizing strategies for better outcomes
Category: AI Agents
Industry: Marketing and Advertising
Predictive Analytics for Market Trend Forecasting
1. Define Objectives
1.1 Identify Key Performance Indicators (KPIs)
Establish the metrics that will measure the success of the predictive analytics initiative, such as customer acquisition cost, conversion rates, and return on investment.
1.2 Set Forecasting Goals
Determine the specific market trends to forecast, such as consumer behavior shifts, emerging product demand, or seasonal sales patterns.
2. Data Collection
2.1 Gather Historical Data
Utilize data from previous marketing campaigns, sales reports, and customer feedback to build a robust dataset.
2.2 Integrate Real-Time Data Sources
Incorporate data from social media, online reviews, and web traffic analytics. Tools such as Google Analytics and Hootsuite can facilitate this integration.
3. Data Preparation
3.1 Clean and Organize Data
Ensure data accuracy by removing duplicates, filling in missing values, and standardizing formats.
3.2 Feature Engineering
Create relevant features that may influence market trends, such as seasonality indicators or demographic segmentation.
4. Model Selection
4.1 Choose Predictive Modeling Techniques
Select appropriate AI-driven algorithms for forecasting, such as:
- Time Series Analysis (e.g., ARIMA)
- Machine Learning Models (e.g., Random Forest, Gradient Boosting)
- Deep Learning Approaches (e.g., LSTM networks)
4.2 Utilize AI Tools
Implement tools such as IBM Watson Studio, Microsoft Azure Machine Learning, or Google Cloud AI to build and deploy predictive models.
5. Model Training and Validation
5.1 Train the Model
Use historical data to train the selected model, ensuring it learns the underlying patterns and relationships.
5.2 Validate Model Performance
Test the model using a separate dataset to evaluate its accuracy and reliability, employing metrics such as Mean Absolute Error (MAE) and R-squared.
6. Implementation and Monitoring
6.1 Deploy the Predictive Model
Integrate the model into existing marketing systems, utilizing platforms like Salesforce or HubSpot for seamless execution.
6.2 Monitor Performance
Continuously track model predictions against actual market trends, adjusting strategies as necessary. Use dashboards from tools like Tableau or Power BI for real-time insights.
7. Reporting and Optimization
7.1 Generate Reports
Create comprehensive reports detailing forecast outcomes, insights, and actionable recommendations for marketing strategies.
7.2 Optimize Marketing Strategies
Refine marketing campaigns based on predictive insights, employing A/B testing to measure effectiveness and further enhance targeting.
8. Feedback Loop
8.1 Collect Feedback
Gather feedback from marketing teams and stakeholders to assess the accuracy and utility of the forecasts.
8.2 Iterate and Improve
Continuously improve the predictive analytics process by incorporating new data and insights, ensuring the model evolves with changing market conditions.
Keyword: predictive analytics market trends