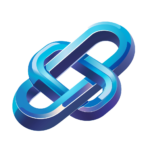
AI Driven Predictive Customer Churn Analysis Workflow Guide
AI-driven predictive customer churn analysis helps businesses identify key metrics set goals gather and integrate data develop and refine models and implement strategies to reduce churn
Category: AI Analytics Tools
Industry: Technology and Software
Predictive Customer Churn Analysis
1. Define Objectives
1.1. Identify Key Metrics
Determine the key performance indicators (KPIs) that will measure customer churn, such as churn rate, customer lifetime value (CLV), and retention rate.
1.2. Set Goals
Establish specific goals for reducing churn, such as minimizing churn by a certain percentage within a defined timeframe.
2. Data Collection
2.1. Gather Customer Data
Collect relevant customer data from various sources, including CRM systems, customer support interactions, and transaction histories.
2.2. Data Integration
Utilize AI-driven tools like Tableau or Power BI to integrate and visualize data from disparate sources for comprehensive analysis.
3. Data Preparation
3.1. Data Cleaning
Implement data cleaning processes to remove duplicates, correct inaccuracies, and handle missing values.
3.2. Feature Engineering
Utilize AI algorithms to identify and create relevant features that may influence churn, such as customer engagement scores and purchase patterns.
4. Model Development
4.1. Select AI Algorithms
Choose appropriate AI algorithms for predictive analysis, such as logistic regression, decision trees, or neural networks.
4.2. Tool Utilization
Employ AI-driven platforms like IBM Watson Studio or Google Cloud AI for model training and validation.
5. Model Evaluation
5.1. Performance Metrics
Evaluate model performance using metrics such as accuracy, precision, recall, and F1 score.
5.2. Model Refinement
Refine the model based on evaluation results, adjusting algorithms and features as necessary to improve predictive accuracy.
6. Implementation
6.1. Integration into Business Processes
Integrate the predictive model into existing business processes, utilizing tools like Salesforce Einstein for real-time insights.
6.2. Staff Training
Provide training for staff on how to interpret model outputs and implement strategies to reduce churn based on predictive insights.
7. Monitoring and Reporting
7.1. Continuous Monitoring
Establish a system for continuous monitoring of churn rates and model performance using dashboards from tools like Looker.
7.2. Feedback Loop
Create a feedback loop to refine the model based on ongoing data and insights, ensuring adaptability to changing customer behaviors.
8. Strategic Action
8.1. Customer Engagement Strategies
Develop targeted engagement strategies based on predictive insights, such as personalized marketing campaigns or proactive customer support.
8.2. Evaluation of Impact
Regularly evaluate the impact of implemented strategies on churn rates and customer satisfaction, adjusting as necessary to optimize results.
Keyword: Predictive customer churn analysis