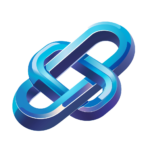
AI Driven Predictive Maintenance Workflow for Grid Assets
Discover how AI-driven predictive maintenance enhances grid asset management through data collection integration modeling and continuous improvement strategies
Category: AI Analytics Tools
Industry: Energy and Utilities
Predictive Maintenance for Grid Assets
1. Data Collection
1.1 Identify Data Sources
Gather data from various sources including:
- SCADA systems
- IoT sensors on grid assets
- Historical maintenance records
- Weather data
1.2 Data Integration
Utilize data integration tools to consolidate data into a centralized system. Examples include:
- Apache Kafka
- Talend
2. Data Preprocessing
2.1 Data Cleaning
Remove inconsistencies and outliers from the dataset to ensure accuracy.
2.2 Feature Engineering
Create relevant features that may impact asset performance. This can include:
- Operating conditions
- Usage patterns
3. Predictive Modeling
3.1 Model Selection
Select appropriate AI models for predictive analysis. Consider using:
- Random Forest
- Support Vector Machines (SVM)
- Neural Networks
3.2 Model Training
Train the selected models using historical data to identify patterns and predict failures.
3.3 Model Validation
Validate the models using a separate dataset to ensure reliability and accuracy.
4. Implementation of AI Analytics Tools
4.1 Deployment of AI Solutions
Implement AI-driven platforms for real-time monitoring and predictive analytics. Tools to consider include:
- IBM Maximo
- GE Digital’s Predix
- Siemens MindSphere
4.2 Real-Time Monitoring
Utilize dashboards and visualization tools to monitor asset health and performance in real-time.
5. Maintenance Scheduling
5.1 Predictive Maintenance Alerts
Generate alerts based on AI predictions for timely maintenance interventions.
5.2 Resource Allocation
Optimize resource allocation for maintenance tasks based on predictive insights.
6. Continuous Improvement
6.1 Performance Review
Regularly review the performance of predictive maintenance strategies and models.
6.2 Model Refinement
Continuously refine models based on new data and insights to improve accuracy.
6.3 Feedback Loop
Establish a feedback loop to incorporate lessons learned into future predictive maintenance efforts.
Keyword: Predictive maintenance for grid assets