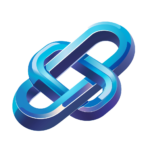
AI Integration in Drug Discovery and Development Workflow
AI-powered drug discovery streamlines the process from problem identification to post-market surveillance enhancing efficiency and accuracy in developing new therapies
Category: AI Analytics Tools
Industry: Healthcare
AI-Powered Drug Discovery and Development
1. Problem Identification
1.1 Define Therapeutic Area
Identify the specific disease or condition that requires new drug development.
1.2 Gather Preliminary Data
Collect existing research, clinical data, and patient feedback to understand the current landscape.
2. Data Collection and Integration
2.1 Acquire Relevant Datasets
Utilize databases such as PubMed, ClinicalTrials.gov, and proprietary datasets to gather comprehensive information.
2.2 Data Integration
Implement tools like Tableau or Microsoft Power BI to integrate and visualize data from multiple sources.
3. AI Model Development
3.1 Select AI Analytics Tools
Choose appropriate AI tools such as IBM Watson or Google Cloud AI for data analysis and model training.
3.2 Train Machine Learning Models
Utilize algorithms to identify patterns and predict drug efficacy. Tools like TensorFlow or PyTorch can be leveraged for model development.
4. Drug Candidate Identification
4.1 Virtual Screening
Employ AI-driven virtual screening tools such as Schrödinger or OpenEye to identify potential drug candidates.
4.2 Predictive Modeling
Use predictive modeling to assess the pharmacokinetics and toxicity of drug candidates with tools like ADMET Predictor.
5. Preclinical Testing
5.1 In Silico Testing
Conduct in silico simulations to predict the biological activity of drug candidates.
5.2 Data Analysis and Refinement
Analyze results using AI analytics tools to refine drug candidates. Tools such as DataRobot can be used for automated machine learning.
6. Clinical Trials
6.1 Trial Design Optimization
Utilize AI tools like Medidata or Oracle’s Siebel CTMS to optimize clinical trial design and patient recruitment.
6.2 Real-Time Monitoring
Implement AI for real-time monitoring of trial data to ensure compliance and safety using platforms like IBM Watson Health.
7. Regulatory Approval
7.1 Submission Preparation
Prepare regulatory submissions using AI tools to streamline documentation and compliance checks.
7.2 Predictive Analytics for Approval
Employ predictive analytics to assess the likelihood of regulatory approval based on historical data.
8. Post-Market Surveillance
8.1 Ongoing Data Collection
Collect real-world data post-launch using AI tools to monitor drug performance and patient outcomes.
8.2 Continuous Improvement
Utilize AI analytics to identify areas for improvement and inform future drug development processes.
Keyword: AI driven drug discovery process