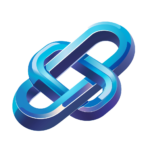
Optimize Precision Irrigation with AI and Machine Learning Insights
Optimize irrigation management with AI-driven machine learning for real-time data collection analysis and strategic decision making in agriculture
Category: AI Analytics Tools
Industry: Agriculture
Precision Irrigation Management Using Machine Learning
1. Data Collection
1.1 Sensor Deployment
Utilize soil moisture sensors, weather stations, and crop health sensors to gather real-time data.
1.2 Data Sources
Integrate data from satellite imagery and aerial drones for comprehensive monitoring.
2. Data Preprocessing
2.1 Data Cleaning
Remove any outliers and irrelevant data points to ensure accuracy.
2.2 Data Normalization
Standardize data formats and scales for consistency across datasets.
3. Machine Learning Model Development
3.1 Feature Selection
Identify key variables affecting irrigation needs, such as soil type, crop type, and weather conditions.
3.2 Model Selection
Choose appropriate machine learning algorithms, such as Random Forest, Support Vector Machines, or Neural Networks.
3.3 Training the Model
Utilize historical data to train the selected model, ensuring it can predict irrigation requirements accurately.
4. Implementation of AI Analytics Tools
4.1 Tool Selection
Evaluate and select AI-driven products such as:
- CropX: Provides soil monitoring and irrigation recommendations based on real-time data.
- Agribotix: Offers drone-based analytics for crop health and irrigation management.
- IBM Watson Decision Platform for Agriculture: Integrates AI to provide insights on irrigation optimization.
4.2 Integration with Existing Systems
Ensure selected tools can seamlessly integrate with existing farm management software.
5. Real-Time Monitoring and Adjustment
5.1 Automated Irrigation Systems
Implement smart irrigation systems that adjust water supply based on AI predictions.
5.2 Continuous Data Analysis
Utilize AI analytics tools for ongoing assessment and refinement of irrigation strategies.
6. Performance Evaluation
6.1 Key Performance Indicators (KPIs)
Establish KPIs such as water usage efficiency, crop yield improvement, and cost savings.
6.2 Feedback Loop
Incorporate feedback mechanisms to continuously improve the machine learning model based on new data and outcomes.
7. Reporting and Decision Support
7.1 Data Visualization
Use dashboards and visualization tools to present data insights to stakeholders.
7.2 Strategic Decision Making
Leverage insights gained from AI analytics to inform future irrigation strategies and agricultural practices.
Keyword: Precision irrigation management solutions