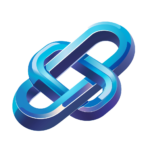
Optimize Workforce Productivity with AI Driven Analysis Solutions
AI-driven workforce productivity analysis enhances efficiency by defining objectives collecting data analyzing results and implementing actionable solutions for continuous improvement
Category: AI Analytics Tools
Industry: Manufacturing
Workforce Productivity Analysis
1. Define Objectives
1.1 Identify Key Performance Indicators (KPIs)
Establish relevant KPIs such as production output, downtime, and employee efficiency.
1.2 Set Goals
Define specific productivity improvement targets based on historical data and industry benchmarks.
2. Data Collection
2.1 Gather Historical Data
Utilize existing manufacturing data, including production logs, employee performance records, and machine utilization rates.
2.2 Implement IoT Sensors
Deploy IoT devices to collect real-time data on machinery performance and workforce activities.
3. Data Analysis
3.1 Utilize AI Analytics Tools
Employ AI-driven analytics tools such as:
- IBM Watson: For predictive analytics to forecast workforce needs and optimize scheduling.
- Siemens MindSphere: To analyze machine data and assess productivity trends.
- Tableau: For visualizing data insights and identifying areas for improvement.
3.2 Perform Root Cause Analysis
Use AI algorithms to identify factors contributing to productivity loss, such as equipment failures or workflow bottlenecks.
4. Develop Action Plan
4.1 Recommend Solutions
Based on analysis, propose actionable solutions such as:
- Process automation using robotic process automation (RPA) tools.
- Workforce training programs tailored to identified skill gaps.
4.2 Set Implementation Timeline
Establish a timeline for executing recommended changes and assign responsibilities to relevant teams.
5. Implementation
5.1 Execute Action Plan
Implement the recommended solutions while ensuring minimal disruption to production.
5.2 Monitor Progress
Continuously track the impact of changes using AI tools to assess improvements in productivity metrics.
6. Review and Optimize
6.1 Evaluate Results
Analyze post-implementation data to determine the effectiveness of the changes made.
6.2 Iterate and Improve
Refine processes based on feedback and ongoing data analysis to ensure continuous productivity enhancement.
7. Reporting
7.1 Create Comprehensive Reports
Generate detailed reports using tools like Microsoft Power BI to present findings and recommendations to stakeholders.
7.2 Share Insights
Disseminate key insights and data-driven recommendations across the organization to foster a culture of continuous improvement.
Keyword: AI driven workforce productivity analysis