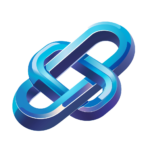
AI Driven Drug Discovery Pipeline Enhancing Efficiency Through Integration
Discover the AI-driven drug discovery pipeline that enhances target identification compound screening lead optimization and clinical trials for effective drug development
Category: AI App Tools
Industry: Pharmaceuticals
AI-Driven Drug Discovery Pipeline
1. Target Identification
The initial phase involves identifying biological targets associated with diseases.
Implementation of AI:
Utilize machine learning algorithms to analyze genomic data and identify potential targets.
Tools:
- DeepMind’s AlphaFold for protein structure prediction
- IBM Watson for Genomics to analyze genetic variations
2. Compound Screening
This stage focuses on screening chemical compounds that could interact with the identified targets.
Implementation of AI:
Employ AI-driven virtual screening techniques to predict compound-target interactions.
Tools:
- Schrödinger Suite for molecular modeling and simulations
- OpenEye Scientific Software for cheminformatics
3. Lead Optimization
Refining the chemical compounds to enhance their efficacy and reduce toxicity.
Implementation of AI:
Leverage predictive modeling to optimize lead compounds and assess their pharmacokinetic properties.
Tools:
- Insilico Medicine for AI-driven drug design
- Atomwise for deep learning in lead optimization
4. Preclinical Testing
This phase involves testing the optimized compounds in vitro and in vivo.
Implementation of AI:
Use AI to analyze preclinical data and predict outcomes based on historical datasets.
Tools:
- BioSymetrics for predictive analytics in preclinical studies
- DataRobot for automating machine learning processes
5. Clinical Trials
Conducting trials to evaluate the safety and efficacy of the drug in human subjects.
Implementation of AI:
Implement AI for patient stratification and monitoring during clinical trials.
Tools:
- Medidata for data analytics in clinical trials
- Oracle’s Siebel CTMS for trial management and patient tracking
6. Regulatory Submission
Preparing and submitting the required documentation to regulatory bodies.
Implementation of AI:
Utilize AI for document management and compliance checks to streamline the submission process.
Tools:
- Veeva Vault for regulatory document management
- IBM Watson for regulatory compliance analysis
7. Post-Market Surveillance
Monitoring the drug’s performance in the market and gathering real-world evidence.
Implementation of AI:
Employ AI to analyze real-world data and detect adverse events or efficacy issues.
Tools:
- SAS for advanced analytics in pharmacovigilance
- HealthVerity for real-world data analysis
Keyword: AI drug discovery pipeline