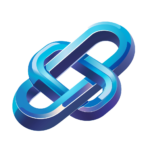
AI Integrated Weather Forecasting and Risk Assessment Workflow
AI-enabled weather forecasting and risk assessment optimizes agriculture through data collection analysis risk evaluation and actionable insights for improved crop management
Category: AI Business Tools
Industry: Agriculture
AI-Enabled Weather Forecasting and Risk Assessment
1. Data Collection
1.1. Weather Data Acquisition
Utilize AI-driven platforms such as IBM Watson Weather and Climacell to gather real-time weather data, historical climate patterns, and satellite imagery.
1.2. Soil and Crop Data Gathering
Integrate sensors and IoT devices to collect soil moisture levels, temperature, and crop health data. Tools like CropX and AgriWebb can facilitate this process.
2. Data Processing and Analysis
2.1. Data Integration
Consolidate weather and agricultural data using AI platforms such as Google Cloud AI or Agriculture.ai to create a comprehensive dataset for analysis.
2.2. Predictive Analytics
Employ machine learning algorithms to analyze patterns and predict weather events. Tools like Microsoft Azure Machine Learning can be utilized for developing predictive models.
3. Risk Assessment
3.1. Risk Identification
Identify potential risks to crops based on predictive analytics. Consider factors such as extreme weather conditions, pest outbreaks, and disease spread.
3.2. Risk Evaluation
Utilize AI-based risk assessment tools like FarmLogs or Climate FieldView to evaluate the severity and impact of identified risks on agricultural operations.
4. Decision Support
4.1. Actionable Insights Generation
Generate actionable insights using AI tools to recommend best practices for mitigating risks, such as irrigation adjustments or crop rotation strategies.
4.2. Stakeholder Communication
Communicate insights to stakeholders using platforms like Slack or Trello to ensure timely decision-making and collaborative action.
5. Implementation and Monitoring
5.1. Execution of Recommendations
Implement the recommended strategies using agricultural management systems such as Ag Leader Technology or Trimble Ag Software.
5.2. Continuous Monitoring
Utilize AI-driven monitoring tools like Sentera or DroneDeploy to continuously track crop health and environmental conditions, allowing for real-time adjustments.
6. Feedback Loop
6.1. Performance Evaluation
Assess the effectiveness of implemented strategies through data analysis and feedback collection, using tools like Tableau for visualization.
6.2. Iterative Improvement
Refine predictive models and risk assessment strategies based on performance data to enhance future forecasting accuracy and agricultural resilience.
Keyword: AI-driven weather forecasting tools