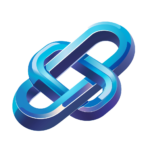
Autonomous Quality Control with AI Integration in Manufacturing
Discover how AI-driven workflow enhances autonomous quality control in manufacturing by optimizing processes and ensuring compliance for superior product quality
Category: AI Business Tools
Industry: Automotive
Autonomous Quality Control in Manufacturing
1. Assessment of Quality Control Needs
1.1 Identify Critical Quality Parameters
Determine the specific quality parameters that are crucial for automotive manufacturing, such as dimensional accuracy, surface finish, and material integrity.
1.2 Analyze Current Quality Control Processes
Evaluate existing quality control workflows to identify inefficiencies and areas for improvement.
2. Integration of AI Technologies
2.1 Selection of AI Tools
Choose appropriate AI-driven tools that can enhance quality control processes. Examples include:
- Computer Vision Systems: Utilize AI-powered cameras to inspect parts for defects in real-time, such as those offered by Cognex and Keyence.
- Predictive Analytics Software: Implement tools like IBM Watson or Microsoft Azure Machine Learning to predict potential failures based on historical data.
- Robotic Process Automation (RPA): Use RPA tools like UiPath to automate repetitive quality inspection tasks.
2.2 Data Collection and Integration
Gather data from various sources, including sensors, production machines, and quality control logs. Ensure seamless integration with existing manufacturing systems.
3. Implementation of AI-Driven Quality Control
3.1 Real-time Monitoring
Deploy AI systems to monitor production lines in real-time, enabling immediate detection of anomalies and defects.
3.2 Automated Decision Making
Utilize AI algorithms to analyze data and make decisions regarding product quality, such as approving or rejecting parts based on predefined criteria.
4. Continuous Improvement and Feedback Loop
4.1 Performance Analysis
Regularly assess the performance of AI quality control systems by analyzing accuracy rates, false positives, and overall efficiency.
4.2 Iterative Refinement
Continuously refine AI models based on feedback and new data to improve detection capabilities and reduce errors.
5. Reporting and Compliance
5.1 Generate Quality Reports
Automate the generation of quality control reports for compliance and auditing purposes, ensuring transparency in quality assurance processes.
5.2 Regulatory Compliance
Ensure that all quality control measures meet industry standards and regulations, utilizing AI tools to facilitate compliance documentation.
6. Training and Development
6.1 Staff Training Programs
Implement training programs for staff on the use of AI tools and the importance of quality control in manufacturing.
6.2 Knowledge Sharing
Encourage a culture of knowledge sharing regarding best practices in quality control and the role of AI in enhancing manufacturing processes.
Keyword: autonomous quality control manufacturing