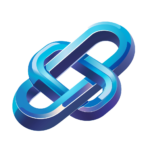
AI Powered Continuous Learning Recommendation Engine for Career Growth
AI-driven Continuous Learning Recommendation Engine enhances career development in consulting by providing personalized learning paths based on skill gaps and industry trends
Category: AI Career Tools
Industry: Consulting Services
Continuous Learning Recommendation Engine
Objective
To develop a Continuous Learning Recommendation Engine that leverages artificial intelligence to enhance career development within consulting services.
Workflow Steps
1. Data Collection
Gather data from various sources to inform the recommendation engine.
- Internal Data: Employee performance reviews, skill assessments, and career progression data.
- External Data: Industry trends, market demands, and emerging technologies.
2. Data Processing
Utilize AI algorithms to process and analyze the collected data.
- Natural Language Processing (NLP): Analyze job descriptions and industry reports to identify key skills and competencies.
- Machine Learning: Implement supervised learning to classify skills and predict learning paths.
3. Skill Gap Analysis
Identify skill gaps by comparing current employee skills with industry requirements.
- AI Tools: Use tools like Skillsoft and LinkedIn Learning to benchmark employee skills against industry standards.
4. Personalized Learning Recommendations
Generate tailored learning paths based on individual employee profiles and identified skill gaps.
- AI-Driven Products: Implement platforms such as Coursera for Business and Udacity to recommend relevant courses and certifications.
5. Implementation of Learning Programs
Facilitate the enrollment of employees in recommended courses and training programs.
- AI Tools: Use Edmodo for tracking course enrollment and progress.
6. Continuous Feedback Loop
Establish a feedback mechanism to assess the effectiveness of the learning programs.
- AI Tools: Utilize Qualtrics for gathering employee feedback and measuring learning outcomes.
7. Performance Evaluation
Evaluate employee performance post-training to measure the impact of the learning recommendations.
- AI Tools: Implement Tableau for data visualization of performance metrics and learning effectiveness.
8. Iteration and Improvement
Refine the recommendation engine based on feedback and performance data to enhance future learning recommendations.
- Machine Learning: Continuously train the model with new data to improve accuracy and relevance of recommendations.
Conclusion
The Continuous Learning Recommendation Engine serves as a strategic tool to empower consulting service employees through personalized learning opportunities, thereby fostering a culture of continuous improvement and professional development.
Keyword: continuous learning recommendation engine