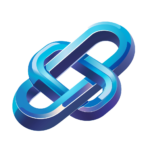
AI Powered Drug Discovery Screening Workflow for Effective Results
AI-driven drug discovery screening workflow enhances therapeutic target identification data collection compound optimization predictive modeling and experimental validation for effective results
Category: AI Coding Tools
Industry: Biotechnology
AI-Driven Drug Discovery Screening Workflow
1. Define Objectives and Requirements
1.1 Identify Therapeutic Targets
Utilize AI algorithms to analyze biological data and identify potential therapeutic targets based on disease mechanisms.
1.2 Establish Screening Criteria
Define the parameters for screening compounds, including efficacy, safety, and pharmacokinetics.
2. Data Collection and Preprocessing
2.1 Gather Relevant Biological Data
Collect data from various sources, including genomic, proteomic, and clinical databases.
2.2 Data Cleaning and Normalization
Employ AI tools such as DataRobot or KNIME to preprocess and normalize data for analysis.
3. Compound Library Preparation
3.1 Curate Compound Libraries
Utilize AI-driven platforms like MolPort to curate and optimize compound libraries for screening.
3.2 Virtual Screening
Implement molecular docking simulations using AI tools such as AutoDock Vina to predict compound-target interactions.
4. Predictive Modeling
4.1 Build Predictive Models
Use machine learning algorithms from platforms like TensorFlow or Scikit-learn to build predictive models for drug efficacy and toxicity.
4.2 Model Validation
Validate models using cross-validation techniques and external datasets to ensure robustness and reliability.
5. Experimental Validation
5.1 In Vitro Testing
Conduct laboratory experiments to validate the predictions made by AI models, utilizing high-throughput screening technologies.
5.2 Data Analysis
Analyze experimental data using AI-driven analytics tools such as GraphPad Prism for statistical evaluation and visualization.
6. Iterative Optimization
6.1 Feedback Loop
Incorporate experimental results back into AI models to refine predictions and improve compound selection.
6.2 Continuous Learning
Utilize reinforcement learning techniques to continuously enhance the drug discovery process based on new data.
7. Documentation and Reporting
7.1 Generate Reports
Create comprehensive reports summarizing findings, methodologies, and recommendations using tools like Tableau for data visualization.
7.2 Regulatory Compliance
Ensure that all documentation meets regulatory standards for drug development and submit to appropriate authorities.
8. Collaboration and Communication
8.1 Stakeholder Engagement
Facilitate communication between interdisciplinary teams using collaboration tools such as Slack or Microsoft Teams.
8.2 Share Findings
Publish research findings and methodologies in scientific journals to contribute to the broader scientific community.
Keyword: AI drug discovery workflow