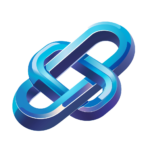
AI Driven Predictive Customer Churn Prevention Workflow Guide
AI-driven pipeline for predictive customer churn prevention includes data collection analysis segmentation and tailored retention strategies for improved customer loyalty
Category: AI Communication Tools
Industry: Finance and Banking
Predictive Customer Churn Prevention Pipeline
1. Data Collection
1.1 Customer Data Acquisition
Gather data from various sources, including:
- Transaction history
- Customer service interactions
- Feedback and surveys
- Social media engagement
1.2 Integration of AI Communication Tools
Utilize AI-driven platforms such as:
- Zendesk – for customer service data analysis
- Salesforce Einstein – for customer relationship management insights
2. Data Processing and Analysis
2.1 Data Cleaning
Ensure data integrity by removing duplicates and correcting inaccuracies.
2.2 Feature Engineering
Identify key features that influence customer retention, such as:
- Frequency of transactions
- Response time to inquiries
- Customer satisfaction scores
2.3 AI Model Development
Implement machine learning algorithms to predict churn. Tools to consider include:
- TensorFlow – for building predictive models
- RapidMiner – for data science workflows
3. Predictive Analytics
3.1 Model Training
Train the model using historical data to identify churn patterns.
3.2 Model Validation
Validate the model’s accuracy using a separate dataset to ensure reliability.
4. Customer Segmentation
4.1 Risk Assessment
Segment customers based on their likelihood to churn, utilizing tools like:
- Tableau – for visualizing customer segments
- Microsoft Power BI – for interactive reporting
4.2 Targeted Interventions
Develop tailored retention strategies for high-risk segments, such as:
- Personalized offers
- Proactive customer support outreach
- Enhanced loyalty programs
5. Implementation of Retention Strategies
5.1 Communication Channels
Utilize AI communication tools to engage customers through:
- Email campaigns
- Chatbots for real-time support
- Social media outreach
5.2 Monitoring and Feedback
Continuously monitor the effectiveness of retention strategies and gather customer feedback for adjustments.
6. Performance Evaluation
6.1 Key Performance Indicators (KPIs)
Define KPIs to measure success, including:
- Churn rate reduction
- Customer satisfaction improvement
- Increased customer lifetime value
6.2 Reporting and Insights
Generate reports using AI analytics tools to provide insights into the effectiveness of the churn prevention pipeline.
7. Continuous Improvement
7.1 Iterative Model Refinement
Regularly update the predictive model with new data to enhance accuracy.
7.2 Feedback Loop
Establish a feedback loop to incorporate insights from customer interactions into the model for ongoing improvement.
Keyword: Predictive customer churn prevention