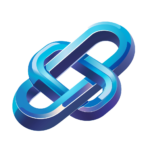
AI Integration for Real Time Load Balancing and Demand Response
AI-driven workflow enhances real-time load balancing and demand response in energy sector optimizing efficiency and improving customer satisfaction
Category: AI Communication Tools
Industry: Energy and Utilities
Real-Time Load Balancing and Demand Response
Overview
This workflow outlines the integration of AI communication tools in the energy and utilities sector for effective real-time load balancing and demand response strategies.
Workflow Steps
1. Data Collection
Utilize AI-driven data collection tools to gather real-time consumption data from various sources, including smart meters and IoT devices.
- Example Tools: Siemens MindSphere, GE Digital’s Predix
2. Data Analysis
Implement AI algorithms to analyze the collected data for patterns in energy usage and demand fluctuations.
- Example Tools: IBM Watson, Microsoft Azure Machine Learning
3. Demand Forecasting
Use predictive analytics to forecast energy demand based on historical data and real-time inputs.
- Example Tools: EnergyHub, AutoGrid
4. Load Balancing Algorithms
Apply AI-driven load balancing algorithms to optimize energy distribution across the grid, ensuring stability and efficiency.
- Example Tools: OpenAI’s GPT for optimization models, Google Cloud AI
5. Demand Response Activation
Engage demand response programs by sending automated alerts to consumers, encouraging them to reduce or shift their energy usage during peak times.
- Example Tools: EnerNOC, Enel X
6. Real-Time Monitoring
Utilize AI communication tools for continuous monitoring of energy consumption and grid performance.
- Example Tools: Schneider Electric’s EcoStruxure, Oracle Utilities
7. Feedback Loop
Establish a feedback mechanism where consumer responses and system performance data are analyzed to refine forecasting and load balancing strategies.
- Example Tools: Tableau for data visualization, Salesforce for customer engagement
8. Reporting and Optimization
Generate reports on energy usage, demand response effectiveness, and load balancing efficiency, using AI to identify areas for improvement.
- Example Tools: Power BI, Qlik
Conclusion
Through the integration of AI communication tools, energy and utility companies can enhance their real-time load balancing and demand response capabilities, leading to improved operational efficiency and customer satisfaction.
Keyword: AI driven load balancing solutions